- お役立ち記事
- Learning from Case Studies of Small Manufacturing Enterprises: How to Start AI Visual Inspection, Gather and Organize Image Information for Machine Learning, and Address Quality Assurance
Learning from Case Studies of Small Manufacturing Enterprises: How to Start AI Visual Inspection, Gather and Organize Image Information for Machine Learning, and Address Quality Assurance
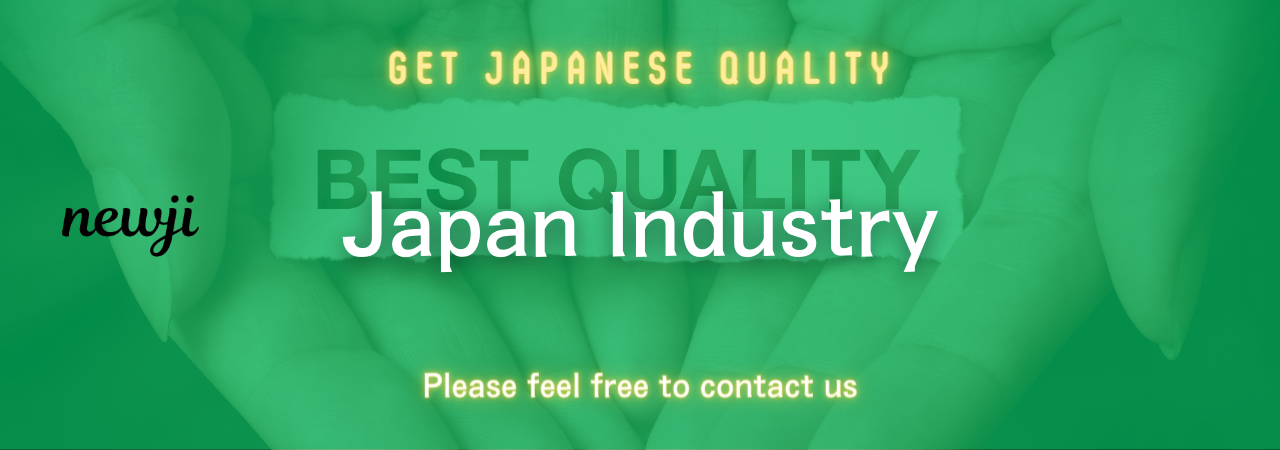
目次
Why Small Manufacturing Enterprises Should Embrace AI Visual Inspection
AI visual inspection is transforming the manufacturing industry.
It offers unparalleled accuracy, consistency, and efficiency in quality control.
For small manufacturing enterprises, adopting this technology can be a significant leap toward improving product quality and operational efficiency.
One compelling reason is cost-effectiveness.
Manual inspection can be labor-intensive and error-prone.
AI visual inspection reduces the need for human intervention, thereby cutting costs in the long run.
Furthermore, the precision of AI systems ensures minimal defects, leading to higher customer satisfaction.
This can result in fewer returns and complaints, ultimately boosting the enterprise’s reputation and profitability.
Steps to Start AI Visual Inspection in Small Manufacturing Enterprises
Assess Your Current Quality Control Processes
Before diving into AI, it’s crucial to understand your existing quality control processes.
Identify areas where defects most frequently occur and evaluate the current methods used to detect these issues.
This assessment will help pinpoint where AI can offer the most significant improvements.
Set Clear Objectives
Define what you aim to achieve with AI visual inspection.
Is it to reduce defect rates, speed up the inspection process, or improve overall product quality?
Having clear objectives will guide your implementation strategy and help measure the effectiveness of the AI system over time.
Choose the Right AI Solution
Not all AI systems are created equal.
Research various AI visual inspection solutions and choose one that fits your specific needs.
Consider factors like ease of integration, scalability, and cost.
Consulting with experts or vendors who specialize in AI for manufacturing can provide valuable insights and help you make an informed decision.
Gathering and Organizing Image Information for Machine Learning
Collect Quality Images
The success of an AI visual inspection system largely depends on the quality of the images fed into it.
Start by collecting high-resolution images of both defective and non-defective products.
This data will serve as the foundation for training your machine learning model.
Ensure the images are diverse, covering all possible defect types and variations.
The more comprehensive your image dataset, the better the AI system will perform.
Label the Images Accurately
Once you have collected the images, label them accurately.
Use clear and consistent labeling that specifies the type and location of defects.
Accurate labeling is critical for training the AI model effectively.
You can use various tools and software to streamline the labeling process.
In some cases, outsourcing this task to professionals may be more efficient.
Organize the Image Data
Proper organization of image data is essential.
Group the images into categories based on defect types, product types, or any other relevant criteria.
This will make it easier to feed the data into the machine learning model.
Use a structured format, such as folders or databases, to maintain and manage the image data.
Regular updates and maintenance of this dataset will be necessary to keep the AI system performing optimally.
Training the Machine Learning Model
Select the Appropriate Algorithm
Choosing the right algorithm is pivotal for the success of your AI visual inspection system.
Different algorithms have different strengths and weaknesses.
Consult with AI specialists to select the most suitable algorithm for your specific needs.
They can guide you in choosing between supervised learning, unsupervised learning, or other advanced techniques.
Start with a Pilot Project
Before fully deploying the AI system, start with a smaller pilot project.
This will allow you to test the system’s effectiveness in a controlled environment.
Monitor the results closely and make any necessary adjustments.
A successful pilot project can pave the way for a more extensive, large-scale implementation.
Addressing Quality Assurance with AI Visual Inspection
Consistent and Unbiased Inspections
One of the significant advantages of AI visual inspection is its consistency.
Unlike human inspectors, AI systems do not get tired or make subjective judgments.
This ensures uniform quality standards across all products.
Consistent inspections lead to fewer defects, higher product quality, and increased customer satisfaction.
It also helps in maintaining compliance with industry standards and regulations.
Real-Time Feedback and Adjustments
AI visual inspection systems provide real-time feedback, enabling immediate corrective actions.
If a defect is detected, the system can alert operators or even halt production to prevent further issues.
This real-time feedback loop is invaluable for maintaining high-quality standards and minimizing waste.
It also allows for ongoing improvement in the manufacturing process.
Data-Driven Decision Making
AI systems generate vast amounts of data that can be analyzed to gain insights into the manufacturing process.
This data can reveal patterns and trends, helping identify root causes of defects.
Manufacturers can use these insights to make informed, data-driven decisions.
This proactive approach to quality assurance leads to continuous improvement and innovation.
Case Studies of Successful AI Visual Inspection Implementations
Small Electronics Manufacturer
A small electronics manufacturer implemented AI visual inspection to improve the quality of its circuit boards.
Initially, the company faced high defect rates due to manual inspection errors.
By adopting AI, they achieved a 30% reduction in defects within six months.
The automated system provided consistent inspections, leading to higher product reliability and customer satisfaction.
Textile Industry Success
In the textile industry, a small enterprise used AI visual inspection to detect fabric defects.
Previously, manual inspection led to missed defects and customer complaints.
The AI system, trained with a diverse dataset of fabric images, significantly improved defect detection accuracy.
This led to a 20% increase in production efficiency and a notable reduction in returns.
Automotive Parts Manufacturer
A small automotive parts manufacturer faced challenges with quality control in its assembly line.
Implementing AI visual inspection allowed the company to detect assembly defects in real time.
This proactive approach reduced defective parts reaching customers by 40%.
The company also benefited from data-driven insights that helped optimize the manufacturing process.
By learning from these case studies, small manufacturing enterprises can understand the tangible benefits of AI visual inspection.
The key is to start with a clear plan, gather quality image data, and implement a system tailored to their specific needs.
Embracing AI technology in quality assurance is not just a trend.
It is a strategic move that can drive long-term success and competitiveness in the manufacturing industry.
資料ダウンロード
QCD調達購買管理クラウド「newji」は、調達購買部門で必要なQCD管理全てを備えた、現場特化型兼クラウド型の今世紀最高の購買管理システムとなります。
ユーザー登録
調達購買業務の効率化だけでなく、システムを導入することで、コスト削減や製品・資材のステータス可視化のほか、属人化していた購買情報の共有化による内部不正防止や統制にも役立ちます。
NEWJI DX
製造業に特化したデジタルトランスフォーメーション(DX)の実現を目指す請負開発型のコンサルティングサービスです。AI、iPaaS、および先端の技術を駆使して、製造プロセスの効率化、業務効率化、チームワーク強化、コスト削減、品質向上を実現します。このサービスは、製造業の課題を深く理解し、それに対する最適なデジタルソリューションを提供することで、企業が持続的な成長とイノベーションを達成できるようサポートします。
オンライン講座
製造業、主に購買・調達部門にお勤めの方々に向けた情報を配信しております。
新任の方やベテランの方、管理職を対象とした幅広いコンテンツをご用意しております。
お問い合わせ
コストダウンが利益に直結する術だと理解していても、なかなか前に進めることができない状況。そんな時は、newjiのコストダウン自動化機能で大きく利益貢献しよう!
(Β版非公開)