- お役立ち記事
- Lifetime distribution used in reliability
月間77,185名の
製造業ご担当者様が閲覧しています*
*2025年2月28日現在のGoogle Analyticsのデータより
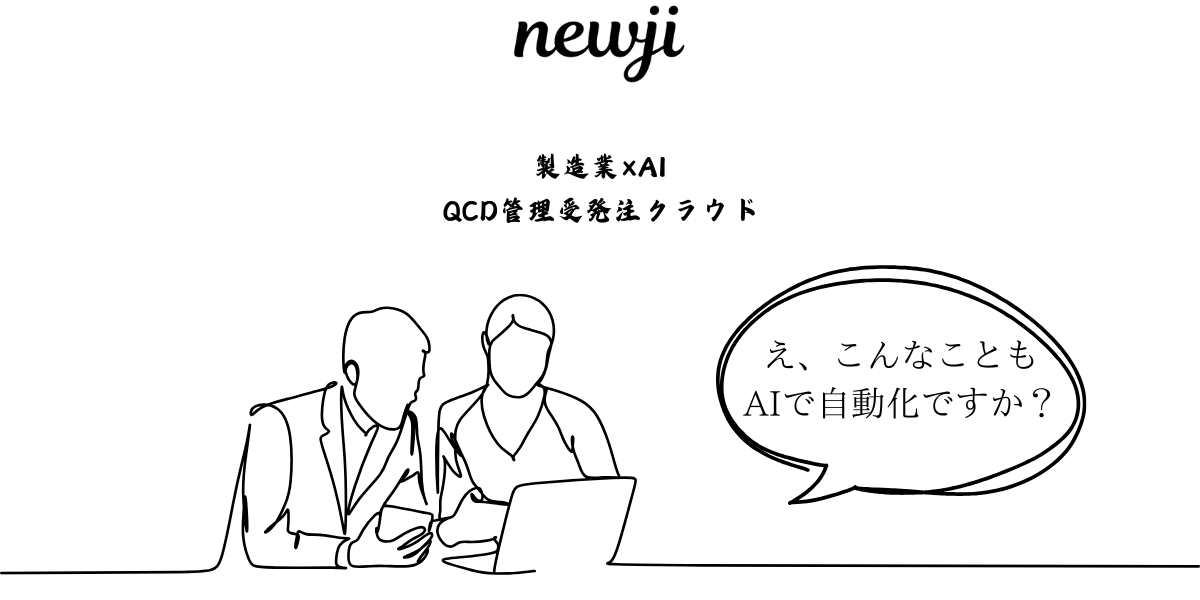
Lifetime distribution used in reliability
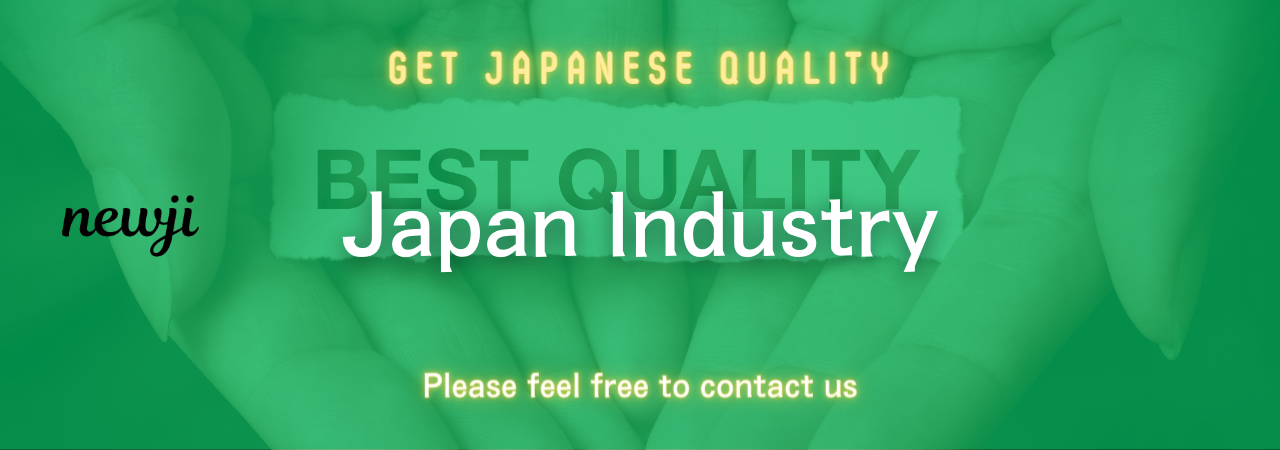
Understanding Lifetime Distribution in Reliability
Reliability is a crucial aspect of product design and engineering.
It refers to the probability that a product or system will perform its intended function over a specific period under stated conditions.
A key component in assessing reliability is understanding how long a product is likely to last before it fails, which is where lifetime distribution comes into play.
What is Lifetime Distribution?
Lifetime distribution is a statistical method used to predict the lifespan of a product or system.
It helps in determining the probability of a failure occurring during a specific time frame.
By analyzing historical data, engineers and designers can model how long a product is expected to last, which is essential for making informed decisions about design improvements, maintenance schedules, and warranty periods.
Types of Lifetime Distribution
Different types of lifetime distributions can be used depending on the nature of the product and the data available.
Some popular lifetime distributions include:
1. Exponential Distribution
The exponential distribution is one of the simplest and most commonly used lifetime distributions.
It assumes that the failure rate of a product is constant over time.
This is often applied to electronic components and systems where failures occur randomly and at a constant average rate.
2. Weibull Distribution
The Weibull distribution is versatile and can model various types of failure rates, such as increasing, decreasing, or constant.
It is suitable for products where the failure rate changes with time, like mechanical parts that might show wear and tear.
3. Normal Distribution
The normal distribution, also known as the Gaussian distribution, is used when failures tend to occur around a central value.
This type of distribution is often applied to products with components that degrade at a predictable rate, such as batteries.
4. Log-Normal Distribution
Similar to the normal distribution but with a logarithmic scale, this distribution is useful for products experiencing a wide range of operating conditions and production variances.
Why Use Lifetime Distribution?
Understanding and applying lifetime distribution in reliability engineering is vital for several reasons:
Product Development and Improvement
By analyzing lifetime data, engineers can identify weaknesses in a product’s design or materials, leading to improvements that enhance reliability and extend the product’s lifespan.
Cost Savings
Accurate lifetime predictions help companies optimize maintenance schedules, reduce downtime, and plan for replacements, thereby saving money on repairs and replacements.
Customer Satisfaction
A reliable product that lasts as expected improves customer satisfaction and loyalty.
Lifetime distribution enables companies to provide realistic warranties and after-sales service, building trust with their customers.
Risk Management
Understanding the likelihood of failure helps businesses manage risk by ensuring that safety and compliance standards are met and mitigating potential liabilities.
How to Use Lifetime Distribution Data?
To effectively use lifetime distribution data, it’s essential to follow a structured approach:
1. Data Collection
Collect accurate and comprehensive data on product lifespans, taking into account factors like environmental conditions, usage patterns, and failure modes.
2. Statistical Analysis
Use statistical software to analyze the data and determine the best-fitting lifetime distribution model for the product in question.
3. Model Validation
Validate the chosen model by comparing its predictions with actual data, ensuring it accurately represents the product’s performance in real-world conditions.
4. Implementation
Apply the insights gained from the model to improve product design, develop maintenance schedules, and plan for strategic replacements.
Challenges in Lifetime Distribution
While lifetime distribution is a powerful tool in reliability engineering, it’s not without challenges:
Data Quality
The accuracy of predictions heavily relies on the quality and completeness of the data collected.
Missing or biased data can lead to incorrect models and unreliable predictions.
Complex Systems
For complex systems with multiple interacting components, modeling lifetime distribution can be challenging due to the various interactions and dependencies present.
Changing Conditions
Products operating under changing conditions, such as varying temperatures or mechanical stresses, may require dynamic models that account for these variations.
Conclusion
Lifetime distribution is a critical component in the field of reliability engineering.
It provides valuable insights into how long products and systems are likely to last, helping engineers and designers make informed decisions.
By selecting the appropriate distribution model and using quality data, companies can improve their products’ reliability, manage costs effectively, and enhance customer satisfaction.
資料ダウンロード
QCD管理受発注クラウド「newji」は、受発注部門で必要なQCD管理全てを備えた、現場特化型兼クラウド型の今世紀最高の受発注管理システムとなります。
ユーザー登録
受発注業務の効率化だけでなく、システムを導入することで、コスト削減や製品・資材のステータス可視化のほか、属人化していた受発注情報の共有化による内部不正防止や統制にも役立ちます。
NEWJI DX
製造業に特化したデジタルトランスフォーメーション(DX)の実現を目指す請負開発型のコンサルティングサービスです。AI、iPaaS、および先端の技術を駆使して、製造プロセスの効率化、業務効率化、チームワーク強化、コスト削減、品質向上を実現します。このサービスは、製造業の課題を深く理解し、それに対する最適なデジタルソリューションを提供することで、企業が持続的な成長とイノベーションを達成できるようサポートします。
製造業ニュース解説
製造業、主に購買・調達部門にお勤めの方々に向けた情報を配信しております。
新任の方やベテランの方、管理職を対象とした幅広いコンテンツをご用意しております。
お問い合わせ
コストダウンが利益に直結する術だと理解していても、なかなか前に進めることができない状況。そんな時は、newjiのコストダウン自動化機能で大きく利益貢献しよう!
(β版非公開)