- お役立ち記事
- Lightweight implementation of brightness correction including deep learning
月間76,176名の
製造業ご担当者様が閲覧しています*
*2025年3月31日現在のGoogle Analyticsのデータより
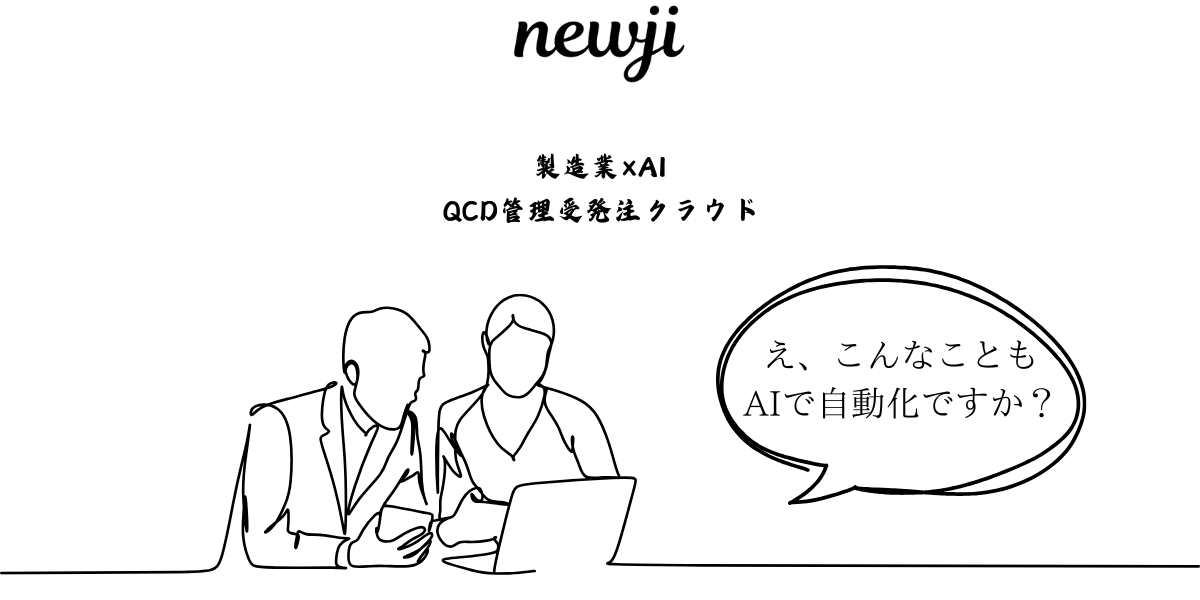
Lightweight implementation of brightness correction including deep learning
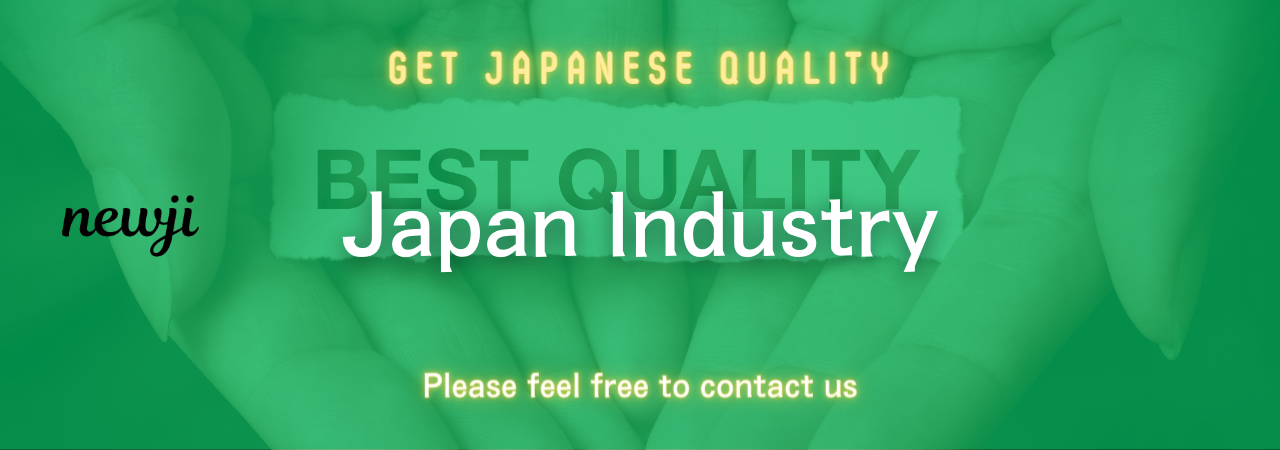
目次
Introduction to Brightness Correction
Brightness correction is a crucial element in digital imaging and video processing.
It plays a pivotal role in enhancing the visibility and details in a scene, ensuring that images or videos are neither too dark nor too bright.
This correction is vital for applications ranging from photography, security surveillance, automotive navigation, to even daily smartphone usage.
With the emergence of deep learning technology, the process of brightness correction has been revolutionized, improving both the quality and efficiency of this essential task.
The Need for Brightness Correction
Natural lighting conditions are rarely perfect.
Images captured under varying lighting can have portions that are too dark (underexposed) or excessively bright (overexposed).
This inconsistency hampers the ability to analyze or appreciate the real details present in the image.
Thus, achieving optimal brightness is necessary to ensure clarity and accuracy.
Brightness correction fixes inconsistencies in lighting, bringing images closer to how the human eye perceives the world.
Traditional vs. Deep Learning Approaches
Traditionally, brightness correction has been approached using algorithms that adjust pixel values based on predefined criteria.
While straightforward, these methods often fail when dealing with complex lighting sources or varying contrasts across an image.
Deep learning, on the other hand, offers a sophisticated alternative.
It leverages neural networks to learn patterns from datasets featuring diverse lighting conditions.
Through training, these networks can identify and correct anomalies in brightness more effectively than traditional methods.
Deep learning models can adapt to varying situations, offering a more dynamic and intelligent approach to brightness correction.
Advantages of Deep Learning in Brightness Correction
1. **Adaptability**: Deep learning models can generalize from training data to handle a wide range of lighting conditions, making them more versatile than rule-based systems.
2. **Automation**: Once trained, deep learning systems can perform brightness correction without human intervention, making them suitable for real-time applications.
3. **Accuracy**: These systems have proven to be more accurate, providing adjustments that preserve natural color hues and contrasts better than traditional techniques.
4. **Scalability**: Deep learning can scale easily to handle large volumes of image and video data, making it suited for industrial applications.
Implementing Lightweight Brightness Correction
One of the challenges with implementing deep learning is the resource intensity.
To address this, lightweight models have been developed.
These models are designed to run efficiently on devices with limited computational power such as smartphones or IoT devices, without compromising on performance.
Techniques for Lightweight Implementation
1. **Model Compression**: Techniques like quantization and pruning are utilized to reduce the size of the neural network model.
Quantization approximates the weights of the model to reduce memory usage, while pruning removes less critical neurons.
2. **Knowledge Distillation**: This involves training a smaller model (the student) to replicate the performance of a larger model (the teacher).
The student model learns to perform brightness correction effectively with fewer parameters.
3. **Neural Architecture Search (NAS)**: This automated process designs models optimized for specific tasks.
By using NAS, a custom architecture can be crafted to optimize brightness correction while minimizing resource usage.
Challenges and Considerations
Implementing lightweight deep learning models for brightness correction comes with its own set of challenges.
The primary concern is maintaining model performance while reducing computational requirements.
Additionally, training such models requires a comprehensive dataset that captures various lighting scenarios.
The training process can be intensive, needing powerful hardware and lengthy processing times.
Another consideration is the deployment environment.
Devices with varying processing capabilities need models that can still perform real-time corrections efficiently.
Balancing performance and resource use is crucial for successful implementation.
The Future of Brightness Correction
With ongoing research and development in AI and machine learning, the future of brightness correction appears promising.
Models are becoming increasingly efficient, offering higher performance with reduced load on system resources.
The use of cloud-based solutions may further enhance the accessibility and scalability of these technologies, allowing integration across a broader range of devices and applications.
Moreover, with advancements in computational photography, newer techniques are emerging to complement brightness correction.
These advancements could lead to even more seamless image and video processing experiences.
In conclusion, lightweight implementations of brightness correction using deep learning present a significant leap forward in image processing technology.
It opens up possibilities for more efficient, scalable, and automated brightness correction solutions that cater to various applications and devices.
As deep learning technology continues to evolve, we can expect even more impressive capabilities in enhancing the quality of visual content.
資料ダウンロード
QCD管理受発注クラウド「newji」は、受発注部門で必要なQCD管理全てを備えた、現場特化型兼クラウド型の今世紀最高の受発注管理システムとなります。
ユーザー登録
受発注業務の効率化だけでなく、システムを導入することで、コスト削減や製品・資材のステータス可視化のほか、属人化していた受発注情報の共有化による内部不正防止や統制にも役立ちます。
NEWJI DX
製造業に特化したデジタルトランスフォーメーション(DX)の実現を目指す請負開発型のコンサルティングサービスです。AI、iPaaS、および先端の技術を駆使して、製造プロセスの効率化、業務効率化、チームワーク強化、コスト削減、品質向上を実現します。このサービスは、製造業の課題を深く理解し、それに対する最適なデジタルソリューションを提供することで、企業が持続的な成長とイノベーションを達成できるようサポートします。
製造業ニュース解説
製造業、主に購買・調達部門にお勤めの方々に向けた情報を配信しております。
新任の方やベテランの方、管理職を対象とした幅広いコンテンツをご用意しております。
お問い合わせ
コストダウンが利益に直結する術だと理解していても、なかなか前に進めることができない状況。そんな時は、newjiのコストダウン自動化機能で大きく利益貢献しよう!
(β版非公開)