- お役立ち記事
- Macro slip occurrence conditions and prevention methods
Macro slip occurrence conditions and prevention methods
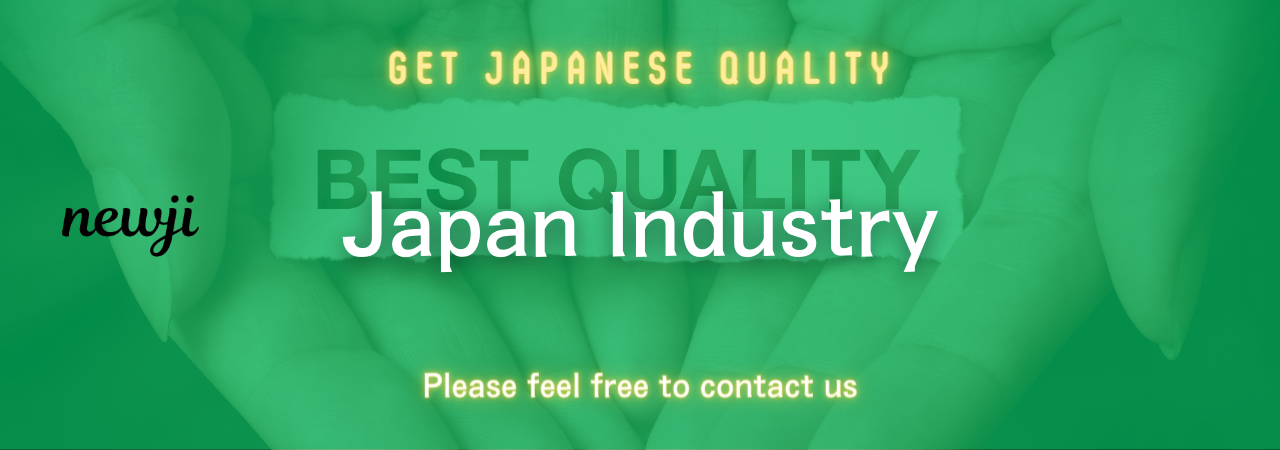
目次
Understanding Macro Slippage
Macro slip refers to the discrepancy that occurs in large-scale models like economic predictions or financial market simulations when predictions deviate from actual outcomes.
This phenomenon can cause significant problems in the accuracy and reliability of decision-making processes, especially in fields heavily reliant on data.
To effectively manage macro slippage, it is crucial to first understand its occurrence and the underlying factors contributing to it.
Causes of Macro Slippage
Several factors contribute to the occurrence of macro slip.
Firstly, the complexity of models is a significant contributor, where overly complex models may unintentionally incorporate inaccuracies.
Furthermore, data quality plays a critical role in slip occurrence. Poor quality or incomplete data can lead to improper model training, resulting in macro slippage.
Another factor is the dynamic nature of real-world environments.
Economic conditions, social behaviors, and technological advancements are constantly evolving, and any unexpected changes can lead to discrepancies between models and actual events.
Additionally, a lack of robustness in models can also contribute to slippage.
Models that are insufficiently robust are more susceptible to errors in novel scenarios not previously encountered during the training phase.
Effects of Macro Slippage
Macro slippage can have far-reaching effects across multiple domains.
In finance, it can lead to significant financial losses owing to incorrect trading strategies or risk assessments.
In economics, it might result in misguided policy-making, potentially causing adverse economic impacts.
Healthcare models experiencing slippage could lead to incorrect disease predictions or ineffective treatment planning.
Thus, understanding and managing macro slip is essential for maintaining the reliability of data-driven methods across these fields.
Methods to Prevent Macro Slip
Appropriately addressing macro slippage involves a combination of techniques aimed at enhancing model accuracy and reliability.
Below are some ways to prevent macro slip from occurring:
Improving Data Quality
Ensuring the highest quality of data is fundamental in reducing macro slip.
This involves collecting comprehensive, clean, and accurate datasets.
Regular audits and updates of data sources can help maintain data integrity, minimizing the chances of inaccuracies feeding into models.
Enhancing Model Simplicity
While complex models may seem appealing, simplicity can often yield better results by reducing the potential for error.
By incorporating only essential variables, it’s easier to pinpoint the sources of discrepancies in simpler models.
Modelers should focus on striking a balance between model complexity and interpretability to avoid unnecessary complications.
Building Robust Models
Robustness is key in model development.
Models should be tested across a wide range of scenarios, including those that anticipate potential environmental changes.
A diverse data set during training phases ensures the model can adapt and perform well under different and unforeseen conditions.
Updating Models Regularly
Regular model updates are essential for maintaining alignment with the prevailing real-world scenarios.
By incorporating recent data and trends, models can remain relevant and reduce the risk of slippage.
Continuous learning from new data helps models adapt and evolve, enhancing accuracy over time.
Collaborative Model Development
Engaging diverse stakeholders in the model development process fosters varied insights, leading to the creation of more holistic models.
Collaboration among professionals from differing domains can integrate multiple perspectives, resulting in well-rounded and robust models.
The Role of Technological Advancements
Technological advancements play a significant role in combating macro slip.
Continuous developments in machine learning and artificial intelligence offer powerful tools for handling complex data and refining predictive models.
New technology enables more sophisticated data handling, improved computational efficiency, and better predictive accuracy, all aiding in the reduction of macro slippage.
Automated Monitoring and Feedback Loops
Implementing automated monitoring systems provides real-time data tracking and model performance, allowing for immediate adjustments when discrepancies are detected.
Feedback loops ensure models learn from past errors, continuously refining and recalibrating to improve accuracy.
Utilizing Big Data Analytics
Big data analytics can harness vast amounts of information, offering deeper insights and uncovering patterns that simpler analyses might miss.
By effectively utilizing big data, models can be trained with highly nuanced, diversified datasets, which helps in minimizing slippage.
Conclusion
Macro slippage is a critical factor that can undermine the effectiveness of large-scale models, affecting diverse fields such as finance, healthcare, and economics.
By understanding the conditions under which macro slip occurs and by implementing preventive measures such as improving data quality, enhancing model simplicity, and leveraging modern technology, the accuracy and reliability of models can be significantly enhanced.
Constant vigilance and adaptability are paramount in reducing the impact of macro slippage, ensuring that models remain as accurate and useful as possible.
資料ダウンロード
QCD調達購買管理クラウド「newji」は、調達購買部門で必要なQCD管理全てを備えた、現場特化型兼クラウド型の今世紀最高の購買管理システムとなります。
ユーザー登録
調達購買業務の効率化だけでなく、システムを導入することで、コスト削減や製品・資材のステータス可視化のほか、属人化していた購買情報の共有化による内部不正防止や統制にも役立ちます。
NEWJI DX
製造業に特化したデジタルトランスフォーメーション(DX)の実現を目指す請負開発型のコンサルティングサービスです。AI、iPaaS、および先端の技術を駆使して、製造プロセスの効率化、業務効率化、チームワーク強化、コスト削減、品質向上を実現します。このサービスは、製造業の課題を深く理解し、それに対する最適なデジタルソリューションを提供することで、企業が持続的な成長とイノベーションを達成できるようサポートします。
オンライン講座
製造業、主に購買・調達部門にお勤めの方々に向けた情報を配信しております。
新任の方やベテランの方、管理職を対象とした幅広いコンテンツをご用意しております。
お問い合わせ
コストダウンが利益に直結する術だと理解していても、なかなか前に進めることができない状況。そんな時は、newjiのコストダウン自動化機能で大きく利益貢献しよう!
(Β版非公開)