- お役立ち記事
- Managing Manufacturing Quality with SPC (Statistical Process Control)
Managing Manufacturing Quality with SPC (Statistical Process Control)
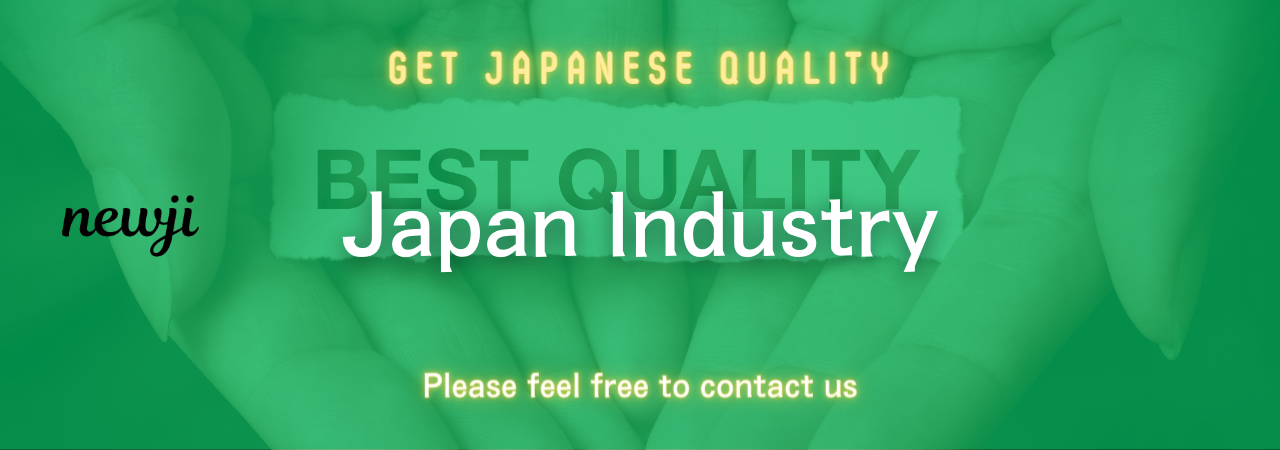
目次
What is SPC (Statistical Process Control)?
Statistical Process Control, or SPC, is a method used in manufacturing to monitor and control the quality of processes and products.
It involves the use of statistical tools and techniques to analyze production data and identify variations in processes.
SPC helps ensure that the production process remains consistent and produces items that meet quality standards.
By identifying and addressing variations early, manufacturers can prevent defects and reduce waste.
Why is SPC Important in Manufacturing?
Maintaining high quality in manufacturing processes is critical for several reasons.
It helps in reducing production costs, minimizes waste, and ensures customer satisfaction by delivering products that meet or exceed expectations.
Moreover, SPC helps in early detection of issues that could otherwise lead to major problems, saving both time and resources.
Using SPC, manufacturers can achieve consistent production quality, which is essential in competitive markets.
Reducing Costs and Waste
One of the main benefits of SPC is its ability to reduce costs and minimize waste.
By constantly monitoring production processes, manufacturers can identify deviations from the standard and take corrective actions before these deviations lead to defects.
This proactive approach prevents the production of substandard products, thereby saving money and reducing material wastage.
Improving Customer Satisfaction
Customer satisfaction hinges on the quality and reliability of the products they purchase.
SPC ensures that the products meet the desired quality standards, which in turn enhances customer trust and loyalty.
Happy customers are more likely to return for future purchases and recommend the products to others.
Key Tools Used in SPC
SPC relies on a number of statistical tools and techniques to analyze data and monitor production quality.
Some of the most commonly used tools in SPC include control charts, histograms, and Pareto charts.
Control Charts
Control charts are graphical tools used to plot data over time.
They help in identifying variations in the process and detecting trends or patterns that may indicate potential issues.
Control charts have upper and lower control limits that define the acceptable range of variation.
If the data points fall within these limits, the process is considered to be in control.
However, if the data points fall outside these limits, it may indicate a problem that needs to be addressed.
Histograms
Histograms are graphical representations of data distribution.
They provide a visual summary of how often different values occur in a dataset.
In SPC, histograms help in understanding the variation in the process and identifying areas that may need improvement.
By analyzing the shape and spread of the histogram, manufacturers can gain insights into the process performance.
Pareto Charts
Pareto charts are used to identify the most significant factors contributing to a problem.
They are based on the Pareto principle, which states that 80% of the problems are caused by 20% of the factors.
Pareto charts help in prioritizing issues and focusing on the most critical ones.
This targeted approach allows manufacturers to address the most impactful problems first, leading to more efficient improvements.
Implementing SPC in Manufacturing
Implementing SPC in manufacturing involves several steps.
It requires a commitment to quality and a willingness to continuously monitor and improve processes.
Data Collection
The first step in implementing SPC is to collect data from the production process.
This data should be accurate and collected at regular intervals to provide a clear picture of the process performance.
Common data collection methods include measurements, inspections, and automated data collection systems.
Data Analysis
Once the data is collected, it must be analyzed using statistical tools and techniques.
The goal is to identify variations in the process and determine their causes.
This analysis helps in understanding the current state of the process and identifying areas that need improvement.
Implementing Improvements
After identifying the areas that need improvement, the next step is to implement corrective actions.
This may involve making adjustments to the process, changing materials, or training employees.
The aim is to bring the process back within control limits and ensure consistent quality.
Continuous Monitoring
SPC is not a one-time effort but a continuous process.
Once improvements are implemented, it is important to keep monitoring the process to ensure that it remains in control.
Regular monitoring helps in detecting new variations early and taking corrective actions promptly.
Challenges in Implementing SPC
While SPC offers numerous benefits, implementing it can be challenging.
It requires a cultural shift towards quality and continuous improvement.
Resistance to Change
One of the main challenges in implementing SPC is resistance to change.
Employees may be accustomed to existing processes and may be hesitant to adopt new methods.
Effective communication and training can help in overcoming this resistance and encouraging employees to embrace SPC.
Data Accuracy
The accuracy of the data collected is critical for the success of SPC.
Inaccurate data can lead to incorrect conclusions and ineffective corrective actions.
Therefore, it is important to ensure that data collection methods are reliable and accurate.
Resources and Training
Implementing SPC requires resources and training.
Employees need to be trained in statistical tools and techniques to effectively use SPC.
Additionally, resources such as software and equipment may be needed for data collection and analysis.
The Future of SPC in Manufacturing
As technology continues to advance, the future of SPC in manufacturing looks promising.
Automation and machine learning are expected to play a significant role in enhancing the capabilities of SPC.
Automation
Automation can streamline data collection and analysis, making the SPC process more efficient.
Automated systems can continuously monitor the production process and provide real-time insights, allowing for quicker and more effective corrective actions.
Machine Learning
Machine learning algorithms can analyze large datasets and identify complex patterns that may not be apparent through traditional statistical methods.
This can lead to more accurate predictions and better decision-making.
By integrating machine learning with SPC, manufacturers can achieve a higher level of quality control and continuous improvement.
In conclusion, SPC is a vital tool in manufacturing for ensuring consistent quality and continuous improvement.
By leveraging statistical tools and techniques, manufacturers can reduce costs, minimize waste, and improve customer satisfaction.
While implementing SPC can be challenging, the benefits far outweigh the effort.
With advancements in technology, the future of SPC looks even more promising, paving the way for more efficient and effective quality control in manufacturing.
資料ダウンロード
QCD調達購買管理クラウド「newji」は、調達購買部門で必要なQCD管理全てを備えた、現場特化型兼クラウド型の今世紀最高の購買管理システムとなります。
ユーザー登録
調達購買業務の効率化だけでなく、システムを導入することで、コスト削減や製品・資材のステータス可視化のほか、属人化していた購買情報の共有化による内部不正防止や統制にも役立ちます。
NEWJI DX
製造業に特化したデジタルトランスフォーメーション(DX)の実現を目指す請負開発型のコンサルティングサービスです。AI、iPaaS、および先端の技術を駆使して、製造プロセスの効率化、業務効率化、チームワーク強化、コスト削減、品質向上を実現します。このサービスは、製造業の課題を深く理解し、それに対する最適なデジタルソリューションを提供することで、企業が持続的な成長とイノベーションを達成できるようサポートします。
オンライン講座
製造業、主に購買・調達部門にお勤めの方々に向けた情報を配信しております。
新任の方やベテランの方、管理職を対象とした幅広いコンテンツをご用意しております。
お問い合わせ
コストダウンが利益に直結する術だと理解していても、なかなか前に進めることができない状況。そんな時は、newjiのコストダウン自動化機能で大きく利益貢献しよう!
(Β版非公開)