- お役立ち記事
- Metal Fatigue and AI-Based Prediction for Strength and Lifetime
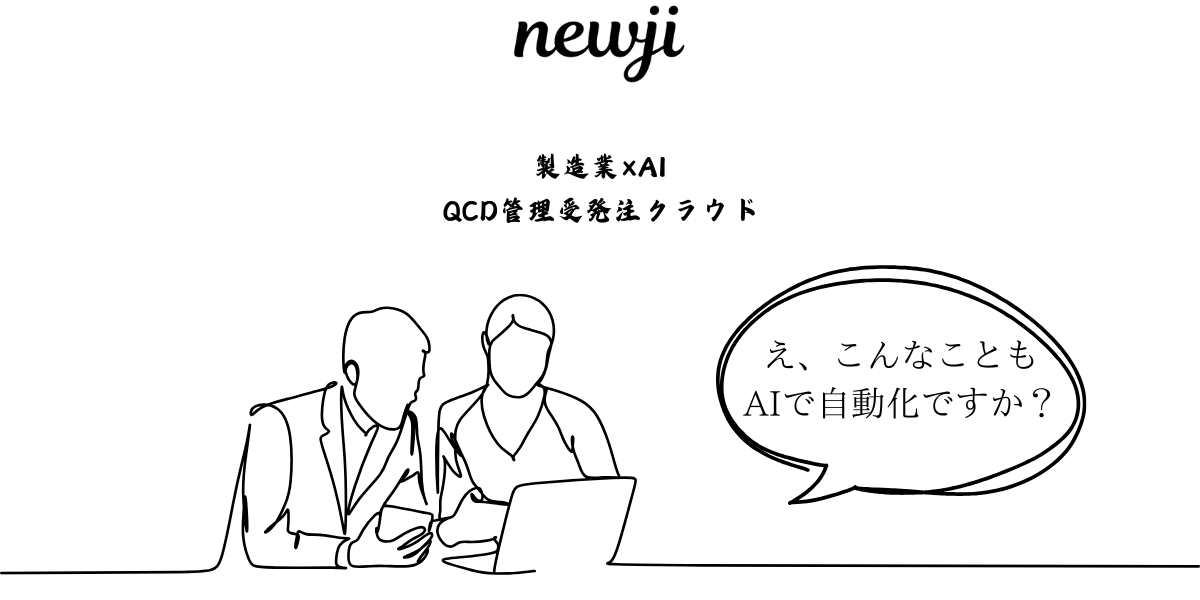
Metal Fatigue and AI-Based Prediction for Strength and Lifetime
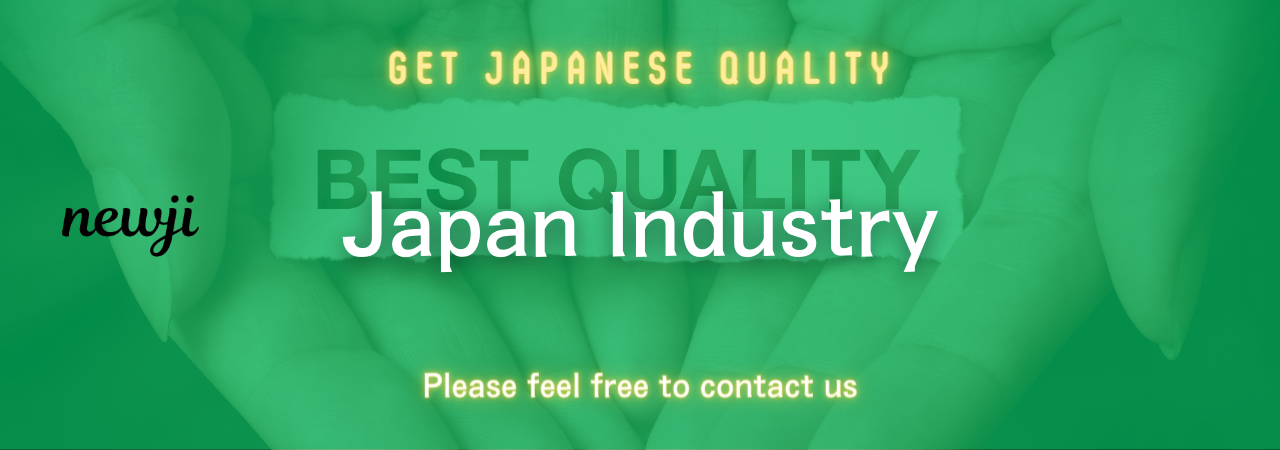
目次
Understanding Metal Fatigue
Metal fatigue is a critical issue in engineering and materials science, affecting the reliability and safety of various structures and components.
It refers to the progressive and localized structural damage that occurs when a material is subjected to repeated loading and unloading cycles.
Over time, this cyclic loading can lead to the initiation and growth of cracks, eventually resulting in catastrophic failure, even if the applied stress is well below the material’s ultimate tensile strength.
In everyday life, metal fatigue can be seen in a range of applications from aircraft wings to bridges, and even in small household items like paper clips.
The study of metal fatigue is essential for predicting the lifespan and ensuring the safety of materials used in critical structures.
The Science Behind Metal Fatigue
At the microscopic level, metal fatigue involves a complex interplay of physical factors.
Initially, small surface or subsurface cracks begin to form at sites of high stress concentration, such as notches, welded joints, or material impurities.
These microscopic cracks grow incrementally with each stress cycle.
The propagation of these cracks can be influenced by environmental factors, such as temperature, corrosion, and surface finish.
Over time, these small cracks can expand and coalesce into larger cracks, eventually compromising the material’s structural integrity.
Traditionally, engineers have used S-N curves (Stress-Number of cycles) to predict the longevity of materials under cyclic loading.
These curves are generated through extensive laboratory testing, which measures the number of cycles to failure at different stress levels.
However, this approach can be time-consuming and may not account for all realistic operating conditions.
The Role of AI in Predicting Metal Fatigue
The advent of artificial intelligence (AI) has revolutionized many fields, and material science is no exception.
AI models can process vast datasets, identify patterns, and make predictions with high accuracy.
In terms of metal fatigue, AI can be used to predict the strength and lifetime of materials more precisely than traditional methods.
AI-Based Models for Fatigue Prediction
Machine learning algorithms, particularly neural networks, have been employed to model metal fatigue behavior.
These models can handle complex datasets that include various factors influencing fatigue life, such as stress amplitude, loading frequency, and environmental conditions.
By training AI models on historical data from fatigue tests, they can learn the intricate relationships between these variables and the material’s fatigue performance.
Once trained, these models can quickly predict fatigue life for new scenarios, significantly reducing the time and cost associated with experimental testing.
Advantages of AI-Based Predictions
AI-based prediction models offer several advantages over traditional fatigue analysis methods.
Firstly, they can handle multi-disciplinary inputs and provide a more holistic assessment of fatigue life, considering various influencing factors simultaneously.
Secondly, AI models can offer real-time predictions and updates as new data becomes available, enhancing the ability to monitor and manage the health of structures.
Moreover, AI can uncover hidden patterns and insights that may not be immediately apparent through conventional analysis.
This capability enables more accurate assessments and improved decision-making, leading to safer and more efficient designs.
Applications of AI in Metal Fatigue Management
The integration of AI in fatigue prediction can have numerous applications across different industries.
Aerospace and Aviation
In the aerospace sector, predicting metal fatigue is crucial for ensuring the safety and reliability of aircraft.
AI can be used to model fatigue in components like turbine blades, landing gears, and structural frames.
By accurately predicting fatigue life, maintenance and inspection schedules can be optimized, reducing downtime and operational costs.
Automotive Industry
In the automotive industry, metal fatigue can affect vehicle components such as suspension systems, axles, and engine parts.
AI can help design more durable vehicles by predicting fatigue life and identifying potential weak points in the design phase.
This can lead to the development of safer and longer-lasting vehicles.
Infrastructure and Construction
Bridges, buildings, and other infrastructure elements are subject to continuous cyclic loading due to environmental factors and human activity.
AI can be used to anticipate metal fatigue in these structures, ensuring timely maintenance and extending their service life.
This is especially valuable in regions prone to harsh weather conditions or seismic activity.
Challenges and Future Directions
While AI offers promising solutions for predicting metal fatigue, there are still challenges to overcome.
Data quality and availability remain significant concerns, as AI models rely on accurate and comprehensive datasets to make reliable predictions.
Additionally, the complexity of creating robust models that generalize well to new scenarios requires ongoing research and development.
Looking forward, the combination of AI with other advanced technologies, such as IoT (Internet of Things) and sensors, could offer even more precise and responsive fatigue management solutions.
These technologies could facilitate continuous monitoring of structures, providing real-time data for AI models to update fatigue predictions dynamically.
Conclusion
Metal fatigue is a critical consideration in the design and maintenance of structures exposed to cyclic loading.
AI provides a powerful tool for predicting and managing fatigue, offering benefits such as improved accuracy, efficiency, and insights into material behavior.
By harnessing AI, industries can enhance safety, optimize maintenance, and extend the lifespan of their critical assets.
As research and technology continue to evolve, the capabilities of AI in fatigue prediction are poised to expand, bringing even greater advancements in material science and engineering.
資料ダウンロード
QCD調達購買管理クラウド「newji」は、調達購買部門で必要なQCD管理全てを備えた、現場特化型兼クラウド型の今世紀最高の購買管理システムとなります。
ユーザー登録
調達購買業務の効率化だけでなく、システムを導入することで、コスト削減や製品・資材のステータス可視化のほか、属人化していた購買情報の共有化による内部不正防止や統制にも役立ちます。
NEWJI DX
製造業に特化したデジタルトランスフォーメーション(DX)の実現を目指す請負開発型のコンサルティングサービスです。AI、iPaaS、および先端の技術を駆使して、製造プロセスの効率化、業務効率化、チームワーク強化、コスト削減、品質向上を実現します。このサービスは、製造業の課題を深く理解し、それに対する最適なデジタルソリューションを提供することで、企業が持続的な成長とイノベーションを達成できるようサポートします。
オンライン講座
製造業、主に購買・調達部門にお勤めの方々に向けた情報を配信しております。
新任の方やベテランの方、管理職を対象とした幅広いコンテンツをご用意しております。
お問い合わせ
コストダウンが利益に直結する術だと理解していても、なかなか前に進めることができない状況。そんな時は、newjiのコストダウン自動化機能で大きく利益貢献しよう!
(Β版非公開)