- お役立ち記事
- Modeling for efficient analysis
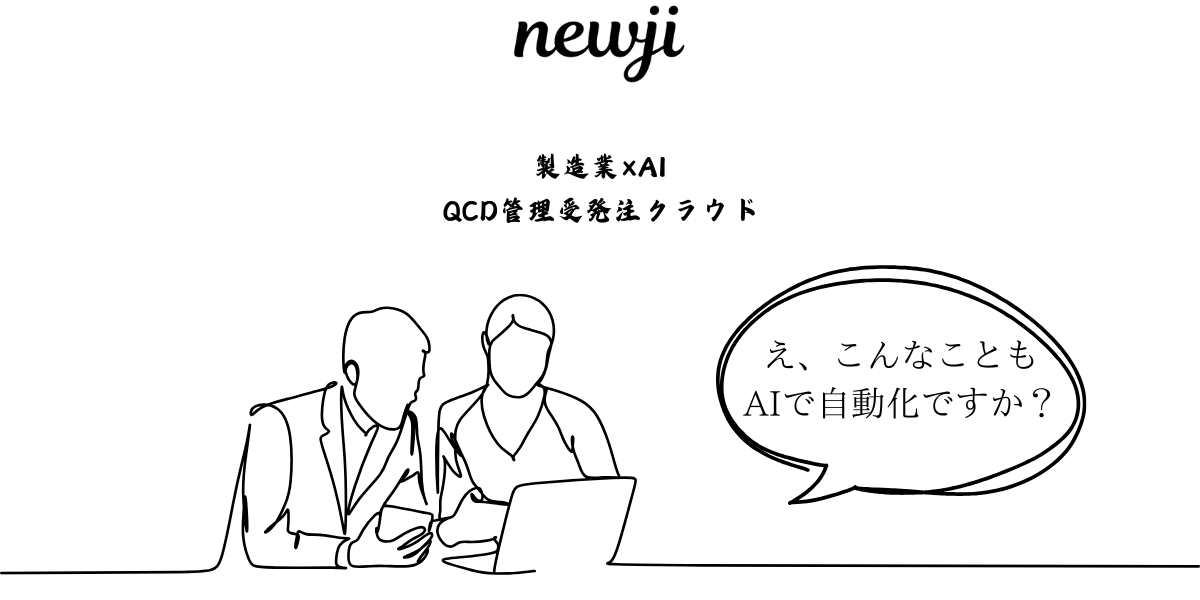
Modeling for efficient analysis
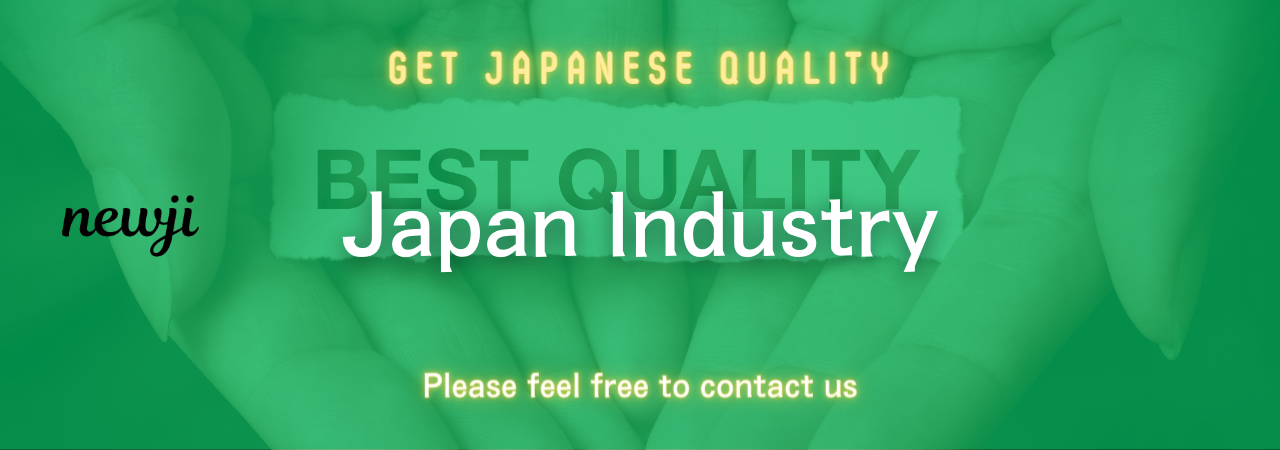
Understanding Modeling for Efficient Analysis
In today’s data-driven world, the ability to analyze data efficiently is crucial for making informed decisions.
The process of gathering, interpreting, and using data effectively can be greatly enhanced through modeling.
But what exactly is modeling, and how does it contribute to efficient analysis?
What is Modeling?
Modeling in the context of data analysis refers to the creation of abstract representations of real-world systems or processes.
These representations, or models, are built using mathematical and statistical methods to simulate the behavior of the actual system.
Models help us predict outcomes, identify patterns, and test hypotheses without the need for direct experimentation.
Types of Models
There are several types of models used depending on the nature of the data and the objective of the analysis.
Some of the common types include:
1. **Predictive Models**: These models are used to predict future outcomes based on historical data.
They use techniques like regression analysis, time series analysis, and machine learning algorithms.
2. **Descriptive Models**: Descriptive models aim to summarize the data and provide insights into the relationships between variables.
Examples include clustering models and factor analysis.
3. **Prescriptive Models**: These models are used to recommend actions based on the predictions and insights gained from predictive and descriptive models.
They are typically used in optimization problems.
4. **Explanatory Models**: These models focus on explaining the relationships and dependencies between different variables in the data.
Benefits of Modeling for Efficient Analysis
Employing models in data analysis provides several benefits:
– **Improved Decision-Making**: By using models, organizations can make more informed decisions based on data-driven insights rather than intuition alone.
– **Cost-Effectiveness**: Models allow analysts to simulate and study different scenarios without incurring the costs associated with direct experimentation.
– **Risk Reduction**: By predicting potential outcomes, models can help organizations identify and mitigate risks before they become issues.
– **Efficiency**: Models streamline the analysis process by providing a structured framework to work within, saving time and resources.
Steps to Create a Model for Analysis
Creating a model involves several steps:
1. **Define the Problem**: Clearly articulate the question or issue that the model needs to address.
Understanding the problem is crucial in selecting the appropriate type and complexity of the model.
2. **Collect Data**: Gather relevant data that will be used to build and test the model.
The quality and quantity of data are critical for accurate modeling.
3. **Select the Model Type**: Choose the model type that aligns with the analysis objectives and the nature of the data.
4. **Develop the Model**: Construct the model using appropriate methods and algorithms.
This includes determining the structure, variables, and parameters needed.
5. **Validate the Model**: Test the model to ensure it adequately represents the real-world system and can make accurate predictions.
Validation may involve comparing model outputs with actual outcomes.
6. **Deploy the Model**: Once validated, implement the model into the decision-making process for ongoing analysis and optimization.
7. **Monitor and Update**: Continuously monitor the model’s performance and update it as needed to accommodate new data or changes in the system.
Challenges in Modeling
While modeling offers numerous advantages, it is not without challenges:
– **Data Quality**: The effectiveness of a model largely depends on the quality of the data used.
Incomplete or inaccurate data can lead to unreliable models.
– **Complexity**: Some models can become overly complex, making them difficult to interpret and manage.
– **Overfitting**: This occurs when a model is too closely tailored to the training data, potentially reducing its effectiveness on new data.
– **Resource Intensive**: Building models can be resource-intensive, requiring expertise in data analysis and computing power.
The Role of Technology in Modeling
Advancements in technology have significantly impacted the field of modeling, enhancing its efficiency and capabilities:
– **Computing Power**: With improved processing capabilities, more complex models can be developed and analyzed faster than ever before.
– **Machine Learning**: Algorithms that enable machines to learn from data without being explicitly programmed have expanded the possibilities for predictive and descriptive modeling.
– **Data Visualization**: Tools that allow for the graphical representation of data and model outputs help stakeholders understand and interpret the analysis results more easily.
– **Collaborative Platforms**: Cloud-based tools facilitate collaboration among teams, enabling them to work together on model development and analysis in real time.
Conclusion
Modeling is a powerful tool for efficient data analysis, providing structured, data-driven insights that can guide decision-making.
By understanding the different types of models and their applications, organizations can leverage them to gain a competitive edge in their respective fields.
Despite the challenges, with the right approach and technology, modeling can offer significant benefits, driving better outcomes and enhancing strategic planning.
資料ダウンロード
QCD調達購買管理クラウド「newji」は、調達購買部門で必要なQCD管理全てを備えた、現場特化型兼クラウド型の今世紀最高の購買管理システムとなります。
ユーザー登録
調達購買業務の効率化だけでなく、システムを導入することで、コスト削減や製品・資材のステータス可視化のほか、属人化していた購買情報の共有化による内部不正防止や統制にも役立ちます。
NEWJI DX
製造業に特化したデジタルトランスフォーメーション(DX)の実現を目指す請負開発型のコンサルティングサービスです。AI、iPaaS、および先端の技術を駆使して、製造プロセスの効率化、業務効率化、チームワーク強化、コスト削減、品質向上を実現します。このサービスは、製造業の課題を深く理解し、それに対する最適なデジタルソリューションを提供することで、企業が持続的な成長とイノベーションを達成できるようサポートします。
オンライン講座
製造業、主に購買・調達部門にお勤めの方々に向けた情報を配信しております。
新任の方やベテランの方、管理職を対象とした幅広いコンテンツをご用意しております。
お問い合わせ
コストダウンが利益に直結する術だと理解していても、なかなか前に進めることができない状況。そんな時は、newjiのコストダウン自動化機能で大きく利益貢献しよう!
(Β版非公開)