- お役立ち記事
- Multiple comparisons from analysis of variance
月間76,176名の
製造業ご担当者様が閲覧しています*
*2025年3月31日現在のGoogle Analyticsのデータより
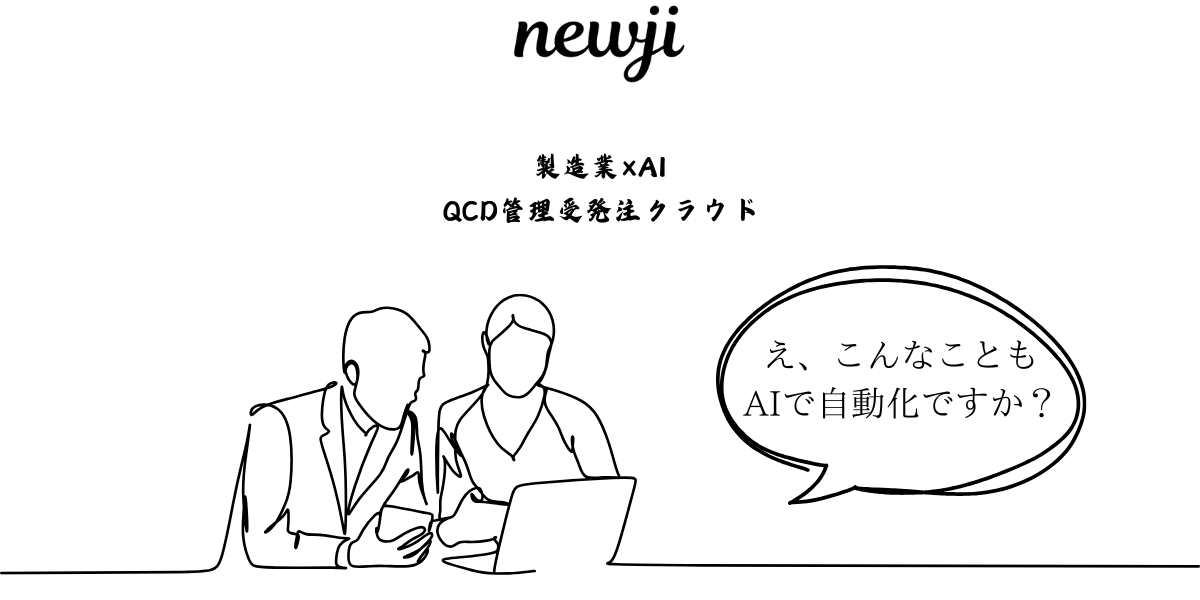
Multiple comparisons from analysis of variance
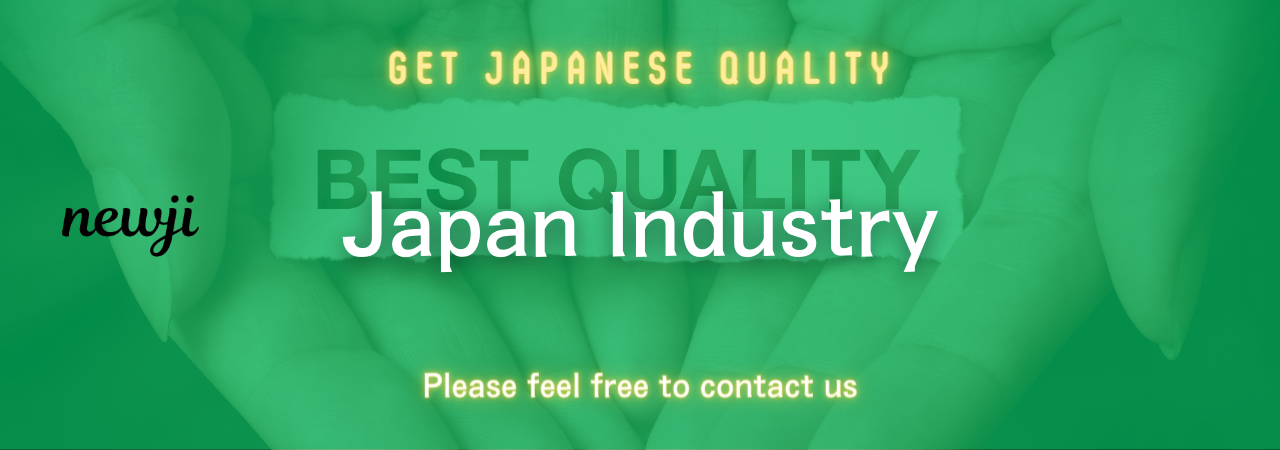
目次
Introduction to Analysis of Variance
Analysis of variance, commonly known as ANOVA, is a statistical method used to analyze differences among group means in a sample.
It is highly beneficial in research across various fields such as psychology, education, and agriculture.
ANOVA helps to determine whether any of the differences between the means of different groups are statistically significant.
When conducting experiments or research, it’s often useful to compare multiple groups simultaneously.
For instance, we might want to compare student performances across different teaching methods or compare the yield of crops with different fertilizers.
ANOVA provides a reliable framework to make these comparisons.
Understanding Multiple Comparisons
While ANOVA can tell us if at least one of the group means is different from the others, it doesn’t specify which groups are different.
This is where multiple comparisons come into play.
Multiple comparisons involve conducting specialized tests to identify which specific groups are different from each other after determining that a difference exists.
Imagine conducting a test with multiple treatment groups, and your ANOVA analysis shows a significant difference.
To pinpoint which groups differ, you’ll need to perform additional tests known as post-hoc tests.
These tests allow us to perform pairwise comparisons while adjusting for the fact that we are making multiple comparisons.
Why Multiple Comparisons Are Important
Performing multiple comparisons is crucial because it reduces the likelihood of Type I errors.
A Type I error occurs when we incorrectly reject a true null hypothesis, effectively claiming that there is a difference when there isn’t one.
In the context of multiple testing, the more comparisons we make, the greater our chances of encountering Type I errors.
For example, if you’re comparing five groups, and you conduct individual t-tests for each pair, the probability of finding a false positive increases with each test.
Using post-hoc testing methods, however, allows you to control the overall error rate across all comparisons, maintaining the integrity of your results.
Common Post-Hoc Tests
There are several post-hoc tests commonly used in conjunction with ANOVA.
Each has its own strengths and applications.
Tukey’s HSD Test
Tukey’s Honest Significant Difference (HSD) test is among the most widely used post-hoc tests.
It compares all possible pairs of means, ensuring that the overall error rate remains constant.
Tukey’s test is particularly useful when you have equal sample sizes for each group, providing a strong control over Type I errors.
Bonferroni Correction
The Bonferroni correction is another technique used to address the issue of multiple comparisons.
It involves adjusting the significance level for each individual test based on the total number of comparisons being made.
While this method is straightforward and can be applied to any set of tests, it can sometimes be overly conservative, particularly when the number of comparisons is large.
Scheffé Test
The Scheffé test is one of the most flexible multiple comparison procedures available.
It can handle both equal and unequal sample sizes, making it particularly versatile.
Although it is less powerful than some other tests like Tukey’s when performing pairwise comparisons, it excels in complex comparisons involving linear contrasts of means.
Newman-Keuls Test
The Newman-Keuls test is a stepwise multiple comparison procedure.
It’s less conservative than the Bonferroni method, often providing more power to detect differences.
However, this flexibility comes at the expense of increased risk of Type I errors compared to more conservative post-hoc tests.
Choosing the Right Post-Hoc Test
Choosing the right post-hoc test depends on the specific conditions of your experiment and what you aim to achieve with your analysis.
Consider the size of your sample groups, the number of comparisons you need to make, and the balance between Type I error risk and test power.
For example, if your priority is minimizing Type I errors and you have equal sample sizes, Tukey’s HSD may be the best choice.
If your sample sizes are unequal or if you need to perform more complex comparisons, the Scheffé test might be more appropriate.
Additionally, understanding the assumptions behind each test, such as homogeneity of variance and normality, will help in selecting the most suitable test for your data.
Conclusion
In summary, multiple comparisons in ANOVA allow researchers to identify specific differences between group means after detecting an overall significant variation.
This is crucial for drawing accurate conclusions and making informed decisions based on statistical analysis.
By familiarizing yourself with different post-hoc tests and their applications, you can better manage the complexity of multiple testing while maintaining control over error rates.
Understanding these concepts will enhance your ability to conduct robust research and contribute valuable insights within your field of study or work.
資料ダウンロード
QCD管理受発注クラウド「newji」は、受発注部門で必要なQCD管理全てを備えた、現場特化型兼クラウド型の今世紀最高の受発注管理システムとなります。
ユーザー登録
受発注業務の効率化だけでなく、システムを導入することで、コスト削減や製品・資材のステータス可視化のほか、属人化していた受発注情報の共有化による内部不正防止や統制にも役立ちます。
NEWJI DX
製造業に特化したデジタルトランスフォーメーション(DX)の実現を目指す請負開発型のコンサルティングサービスです。AI、iPaaS、および先端の技術を駆使して、製造プロセスの効率化、業務効率化、チームワーク強化、コスト削減、品質向上を実現します。このサービスは、製造業の課題を深く理解し、それに対する最適なデジタルソリューションを提供することで、企業が持続的な成長とイノベーションを達成できるようサポートします。
製造業ニュース解説
製造業、主に購買・調達部門にお勤めの方々に向けた情報を配信しております。
新任の方やベテランの方、管理職を対象とした幅広いコンテンツをご用意しております。
お問い合わせ
コストダウンが利益に直結する術だと理解していても、なかなか前に進めることができない状況。そんな時は、newjiのコストダウン自動化機能で大きく利益貢献しよう!
(β版非公開)