- お役立ち記事
- Noise removal technology that utilizes differences in spectral statistics and shapes
Noise removal technology that utilizes differences in spectral statistics and shapes
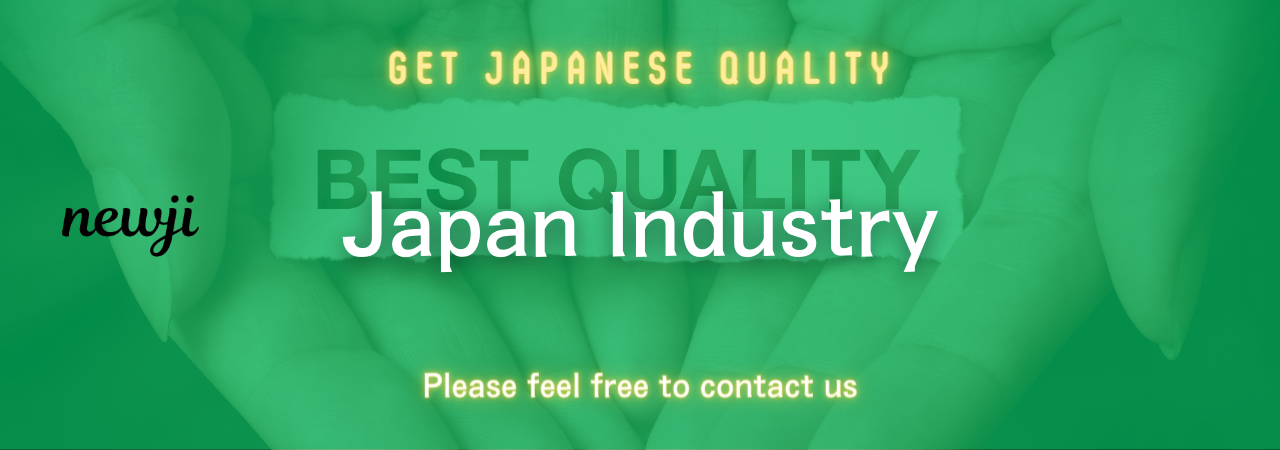
目次
Understanding Noise Removal Technology
Noise removal technology has been a topic of interest and development for many years.
It plays a crucial role in various fields, including audio processing, medical imaging, and telecommunications.
The technology aims to enhance the quality of signals by filtering out unwanted noise.
One of the innovative approaches to noise removal is the utilization of differences in spectral statistics and shapes.
In this article, we will explore how this method works and its applications.
What is Noise in Signal Processing?
Before delving into the specifics of the technology, it’s essential to understand what ‘noise’ means in the context of signal processing.
Noise refers to any unwanted or irrelevant information that interferes with the original signal.
This interference can degrade the quality of the intended signal, making it challenging to interpret or analyze.
Common sources of noise include electrical interference, equipment limitations, and environmental factors.
Different techniques have been developed over the years to mitigate the effects of noise and preserve the integrity of the original signal.
The Role of Spectral Statistics in Noise Removal
Spectral statistics involve the analysis of signals in the frequency domain rather than the time domain.
By examining the frequency components of a signal, it’s possible to identify and separate the noise from the actual data.
This process relies on statistical measures, such as mean and variance, to distinguish meaningful patterns from random variations.
In noise removal technology, differences in spectral statistics between noise and the desired signal can be leveraged effectively.
Noise often exhibits distinct statistical properties that differ from those of the actual signal.
By recognizing these differences, it’s possible to filter out the noise, preserving only the relevant information.
How Spectral Statistics Differ from Signal and Noise
Spectral statistics help differentiate between signal and noise based on their frequency characteristics.
While the signal typically manifests as a structured pattern with consistent frequency peaks, noise is more random and exhibits no specific pattern.
By analyzing these attributes, algorithms can be designed to identify and eliminate undesired noise while retaining the integrity of the original signal.
This approach is particularly effective in applications where the signal’s frequency characteristics are well-defined and distinct from the surrounding noise.
Utilizing Shape Differences for Noise Removal
In addition to spectral statistics, the shape or waveform of a signal can also be used to identify and filter out noise.
Every signal has a unique shape that represents how its amplitude changes over time.
By examining these shapes, it’s possible to detect anomalies caused by noise and remove them from the data.
Shape-based noise removal is particularly beneficial in scenarios where the signal maintains a consistent form.
Deviations from this form can be attributed to noise, allowing for targeted removal without affecting the signal’s core content.
Combining Spectral and Shape Analysis
The true potential of noise removal technology lies in combining both spectral statistics and shape analysis.
By leveraging differences in both aspects simultaneously, the effectiveness of noise removal can be significantly enhanced.
This comprehensive approach allows for a multi-dimensional analysis of the signal, ensuring that even subtle noise components are detected and eliminated.
The combination of these methods provides a robust framework for noise reduction, adaptable across various applications and industries.
Applications of Noise Removal Technology
The technology’s versatility means it finds application in a wide range of fields.
Below are some areas where noise removal technology, utilizing differences in spectral statistics and shapes, has proven to be highly effective.
Audio Processing
In audio processing, noise removal technology is vital for improving sound quality in music, speech, and broadcasting.
Spectral and shape analysis can effectively isolate background noise from the primary audio content, resulting in clearer, more immersive listening experiences.
This technology is used in various audio editing software and hardware devices to ensure optimal sound clarity and quality.
Medical Imaging
Medical imaging, such as MRI and CT scans, relies heavily on signal clarity to provide accurate diagnoses.
Noise in imaging data can obscure important details, making it difficult for healthcare professionals to assess patient conditions accurately.
By utilizing noise removal technology, medical devices can produce cleaner images, aiding in more reliable diagnostic outcomes.
Telecommunications
Reliability and clarity are crucial in telecommunications systems, where signal interference can affect communication quality.
Noise removal technologies harness differences in spectral statistics and shapes to reduce interference, ensuring that transmitted signals are clear and accurate.
This results in better voice and data communication, especially in noisy environments or over long distances.
Challenges and Future Directions
While noise removal technology has made significant strides, challenges remain.
Developing algorithms that can reliably distinguish between noise and signal in complex environments is an ongoing area of research.
Furthermore, balancing noise reduction with computational efficiency is crucial, especially in real-time applications.
As technology advances, we can expect noise removal methods to become more sophisticated and integrated with emerging technologies.
Machine learning and artificial intelligence may play a greater role in developing adaptive noise removal systems that can learn and improve over time.
Conclusion
Noise removal technology utilizing differences in spectral statistics and shapes represents a significant advancement in the field of signal processing.
This approach provides a comprehensive framework for enhancing signal quality across various applications.
As research continues, we can anticipate further innovations that will improve the effectiveness and applicability of noise removal techniques, cementing their importance in our increasingly digital world.
資料ダウンロード
QCD調達購買管理クラウド「newji」は、調達購買部門で必要なQCD管理全てを備えた、現場特化型兼クラウド型の今世紀最高の購買管理システムとなります。
ユーザー登録
調達購買業務の効率化だけでなく、システムを導入することで、コスト削減や製品・資材のステータス可視化のほか、属人化していた購買情報の共有化による内部不正防止や統制にも役立ちます。
NEWJI DX
製造業に特化したデジタルトランスフォーメーション(DX)の実現を目指す請負開発型のコンサルティングサービスです。AI、iPaaS、および先端の技術を駆使して、製造プロセスの効率化、業務効率化、チームワーク強化、コスト削減、品質向上を実現します。このサービスは、製造業の課題を深く理解し、それに対する最適なデジタルソリューションを提供することで、企業が持続的な成長とイノベーションを達成できるようサポートします。
オンライン講座
製造業、主に購買・調達部門にお勤めの方々に向けた情報を配信しております。
新任の方やベテランの方、管理職を対象とした幅広いコンテンツをご用意しております。
お問い合わせ
コストダウンが利益に直結する術だと理解していても、なかなか前に進めることができない状況。そんな時は、newjiのコストダウン自動化機能で大きく利益貢献しよう!
(Β版非公開)