- お役立ち記事
- Nonlinearity/uncertainty suppression methods and stabilization technologies in mechatronic control and their key points
月間76,176名の
製造業ご担当者様が閲覧しています*
*2025年3月31日現在のGoogle Analyticsのデータより
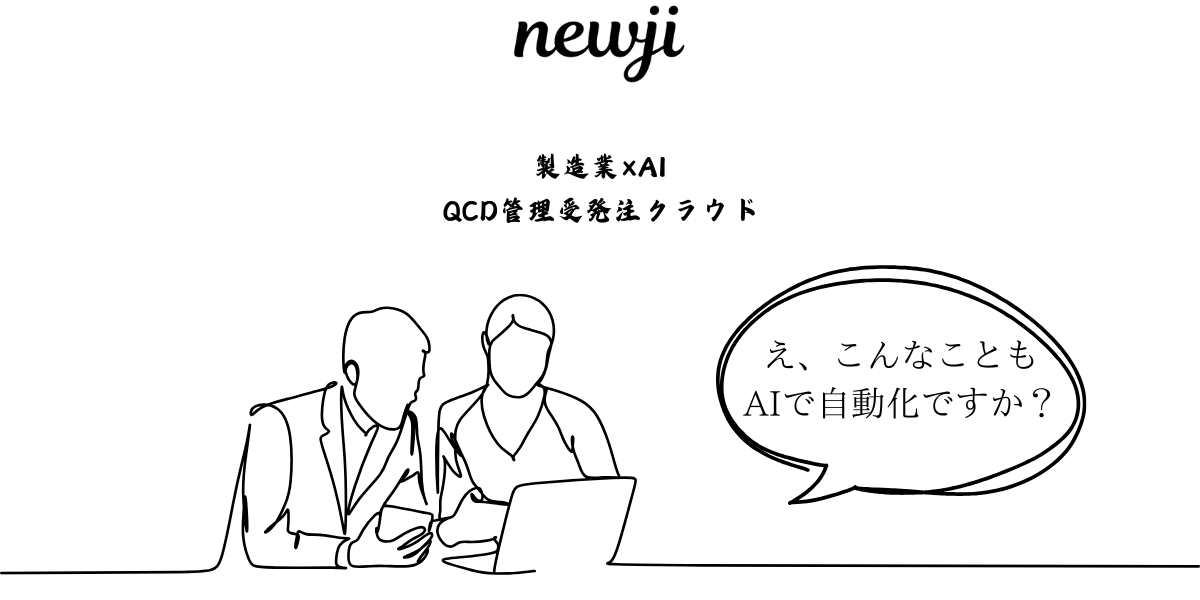
Nonlinearity/uncertainty suppression methods and stabilization technologies in mechatronic control and their key points
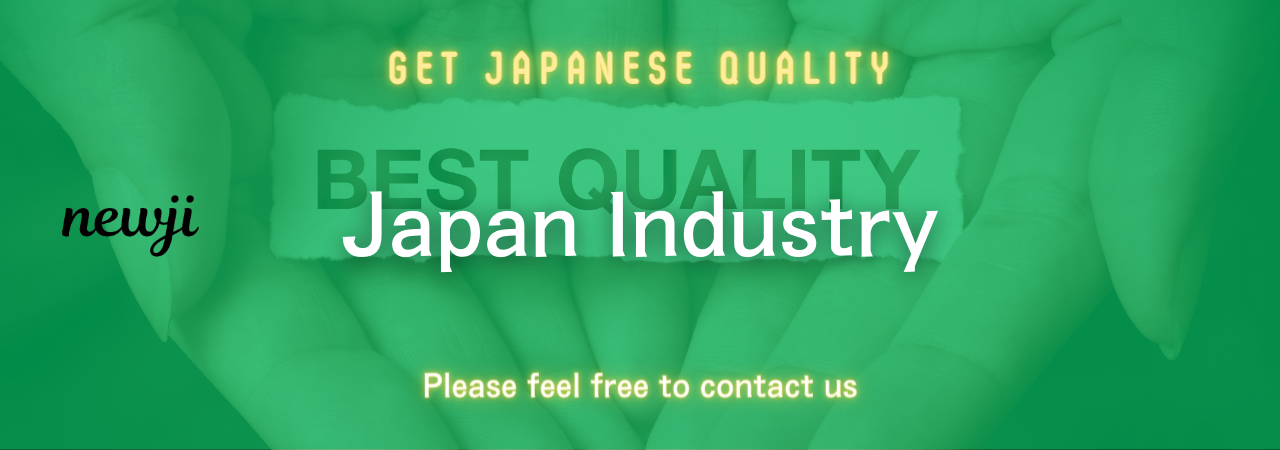
目次
Understanding Nonlinearity and Uncertainty in Mechatronic Systems
Mechatronics is an interdisciplinary branch of engineering that combines mechanical, electrical, and computer systems.
One of the significant challenges in mechatronic systems is dealing with nonlinearity and uncertainty in control processes.
Nonlinearity refers to the situation where a change in input does not result in a proportional change in output.
Uncertainty, on the other hand, involves unpredictability in system parameters or the external environment.
Addressing these issues is crucial for the stability and performance of mechatronic systems.
The Impact of Nonlinearity and Uncertainty
Nonlinear behaviors can arise from various sources in a mechatronic system.
These include friction, backlash in gears, or any component whose response isn’t directly proportional to the input.
Such nonlinear dynamics can complicate the control processes and lead to unexpected system behaviors.
Uncertainty can stem from sensor noise, model inaccuracies, or external disturbances.
These factors make it difficult to predict exactly how a system will behave under certain conditions.
When not addressed, these uncertainties can lead to performance degradation or even system instability.
Suppression Methods for Nonlinearity and Uncertainty
To ensure optimal performance, various methods have been developed to suppress the effects of nonlinearity and uncertainty.
Feedback Control
Feedback control is a widely used approach to counteract the issues of nonlinearity and uncertainty.
By continually measuring the output and adjusting inputs accordingly, feedback systems can adapt to changing conditions.
This adaptability helps stabilize the system, even when facing unpredictable disturbances.
Adaptive Control
Adaptive control systems are designed to adjust their parameters automatically to meet the varying conditions of the system.
They learn from the ongoing behavior of the system and modify control strategies in real-time.
This approach is particularly effective for systems with significant model uncertainties or slowly varying dynamics.
Robust Control
Robust control techniques focus on maintaining performance despite bounded uncertainties.
These systems are designed to withstand variations in parameters, ensuring stability and performance within a specific range of uncertainties.
Robust control is invaluable for systems operating in a dynamic environment where disturbances are expected.
Fuzzy Logic Control
Fuzzy logic control uses reasoning similar to human decision-making to manage uncertainty and nonlinearity.
Instead of relying on precise numerical data, it uses approximate values and linguistic terms.
This flexibility allows fuzzy logic systems to handle complex and vague information, making them suitable for systems with high uncertainty.
Stabilization Technologies in Mechatronic Control
Stabilization is a critical aspect of enhancing the performance and reliability of mechatronic systems.
Several technologies and strategies have been developed to achieve effective stabilization.
Model Predictive Control (MPC)
Model Predictive Control is an advanced method that uses a model of the system to predict future behavior.
By solving an optimization problem at each control step, MPC can take future constraints and disturbances into account.
This foresight improves system stability and performance, making MPC ideal for complex multivariable systems.
State Estimation Techniques
State estimation techniques, such as Kalman filters, are crucial in scenarios with incomplete or noisy measurements.
By estimating the true state of the system from observed data, state estimation helps in reducing the impact of uncertainties and enhancing the reliability of the control system.
Sliding Mode Control
Sliding mode control is used to maintain robustness against model uncertainties and external disturbances.
This method forces the system state to “slide” along a predetermined surface in the state space, addressing nonlinearities and ensuring stability.
Its simplicity and reliability make it popular in systems that require precise control.
Machine Learning for Predictive Control
The integration of machine learning into mechatronic control systems is a growing trend.
Machine learning algorithms can predict outcomes and optimize control strategies based on historical data.
Using techniques like neural networks, systems can adapt and improve their performance over time, enhancing their resilience to uncertainty and nonlinearity.
Key Points in Implementing Control and Stabilization
Implementing effective control and stabilization requires careful consideration of several key points.
Accurate System Modeling
An accurate model is the foundation of any control strategy.
Understanding the system’s dynamics and identifying potential nonlinearities and sources of uncertainty are essential.
A well-calibrated model can significantly improve the performance of control strategies.
Real-Time Processing Capabilities
For adaptive and predictive control strategies, real-time processing capabilities are vital.
Systems must process data and adjust control parameters rapidly to respond effectively to changes in real-world conditions.
Integration of Multiple Strategies
In many cases, using a combination of control and stabilization strategies yields the best results.
For instance, integrating feedback control with adaptive or robust methods can enhance overall system performance.
Continuous Monitoring and Adjustment
Continuous monitoring is crucial for maintaining system stability.
Advanced sensors and data analysis tools can provide real-time insights into system performance, facilitating timely adjustments.
Conclusion
Suppression of nonlinearity and uncertainty, along with effective stabilization techniques, is crucial for the reliable performance of mechatronic systems.
As technology advances, more sophisticated and integrated approaches are being developed, offering greater resilience to the inherent challenges in mechatronic control.
By focusing on key aspects such as accurate modeling, real-time processing, and integrating multiple strategies, engineers can significantly enhance system performance and reliability.
This ongoing evolution in control strategies represents the pathway to smarter, more robust mechatronic systems.
資料ダウンロード
QCD管理受発注クラウド「newji」は、受発注部門で必要なQCD管理全てを備えた、現場特化型兼クラウド型の今世紀最高の受発注管理システムとなります。
ユーザー登録
受発注業務の効率化だけでなく、システムを導入することで、コスト削減や製品・資材のステータス可視化のほか、属人化していた受発注情報の共有化による内部不正防止や統制にも役立ちます。
NEWJI DX
製造業に特化したデジタルトランスフォーメーション(DX)の実現を目指す請負開発型のコンサルティングサービスです。AI、iPaaS、および先端の技術を駆使して、製造プロセスの効率化、業務効率化、チームワーク強化、コスト削減、品質向上を実現します。このサービスは、製造業の課題を深く理解し、それに対する最適なデジタルソリューションを提供することで、企業が持続的な成長とイノベーションを達成できるようサポートします。
製造業ニュース解説
製造業、主に購買・調達部門にお勤めの方々に向けた情報を配信しております。
新任の方やベテランの方、管理職を対象とした幅広いコンテンツをご用意しております。
お問い合わせ
コストダウンが利益に直結する術だと理解していても、なかなか前に進めることができない状況。そんな時は、newjiのコストダウン自動化機能で大きく利益貢献しよう!
(β版非公開)