- お役立ち記事
- Pattern recognition of sensor data using Python, scikit-learn, and numpy
Pattern recognition of sensor data using Python, scikit-learn, and numpy
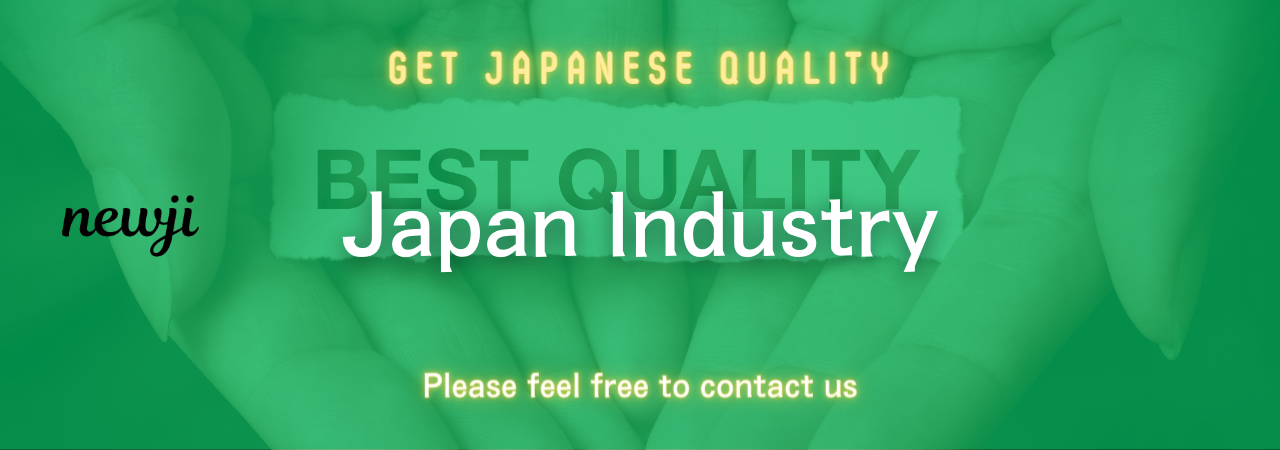
目次
Introduction to Pattern Recognition
Pattern recognition is an essential part of modern technology and plays a significant role in various applications, from identifying speech to recognizing images and detecting anomalies in data.
The goal is to classify input data into categories or identify a structure that can facilitate further data analysis.
When it comes to using sensory data, the task can often be quite complex, requiring the use of advanced algorithms and tools.
Python, along with libraries like scikit-learn and numpy, provides powerful tools for developers and data scientists to perform pattern recognition with ease and efficiency.
Understanding Sensor Data
Sensor data is gathered from devices that capture real-world data, such as accelerometers, temperature sensors, and image sensors.
This data is generally represented in the form of continuous numerical values, which are used to determine the state or behavior of the environment or object being monitored.
The sheer volume and complexity of sensor data necessitate the use of sophisticated computational techniques to extract meaningful patterns or trends.
Typical Applications
Some common applications of pattern recognition in sensor data include:
– Health Monitoring: Detecting abnormalities in heart rate and other vital signs.
– Environmental Monitoring: Identifying pollution levels or changes in climate data.
– Industrial Automation: Monitoring machinery for fault detection and preventive maintenance.
– Smart Homes: Automating devices based on occupancy detection and other parameters.
Python Libraries for Pattern Recognition
Python is a popular programming language for data scientists owing to its simplicity and the availability of specialized libraries like scikit-learn and numpy, which help facilitate pattern recognition.
Numpy
Numpy is vital for numerical computation in Python.
It provides support for large, multi-dimensional arrays and matrices, along with a collection of mathematical functions to operate on these arrays.
Its efficiency in processing large volumes of data makes it ideal for handling sensor data.
Scikit-learn
Scikit-learn is a robust library for machine learning that simplified the integration of predictive data analysis.
With comprehensive documentation and ease of use, it is often favored in academic and industrial applications.
It includes various algorithms for classification, regression, clustering, and dimensionality reduction, making it a valuable tool for pattern recognition.
Steps for Pattern Recognition in Sensor Data
To perform pattern recognition using Python and its libraries, a systematic approach is essential.
1. Data Collection
The first step involves gathering the sensor data that you want to analyze.
Sensors may output data in various formats, so converting the data into a suitable format, such as CSV or JSON, is often necessary before using it as input.
2. Data Preprocessing
Cleaning and transforming data is a critical step for accurate pattern recognition.
Handle missing data, remove noise, and standardize or normalize the input data.
Python libraries like pandas and numpy can help preprocess data by filling missing values, smoothing fluctuations, or scaling data.
3. Feature Selection and Extraction
Selecting the right features is crucial for building an effective model.
Feature selection reduces the dimensionality of the data by selecting only the most relevant features, while feature extraction creates new features by transforming the data.
Library tools like Principal Component Analysis (PCA) in scikit-learn can assist in these processes.
4. Model Training
Use the processed features to train a machine learning model.
Scikit-learn offers a variety of supervised and unsupervised learning algorithms suitable for pattern recognition, such as Support Vector Machines (SVM), Decision Trees, and K-Nearest Neighbors (KNN).
It is essential to choose an appropriate algorithm based on the nature of the data and the problem at hand.
5. Model Evaluation
After training, evaluate the model’s performance using techniques such as cross-validation and by calculating metrics like precision, recall, and accuracy.
Scikit-learn provides functions for splitting the data into training and test sets and for evaluating the performance of the model with different metrics.
6. Model Deployment
Once a satisfactory model is developed, it can be deployed to analyze real-time sensor data.
This may involve integrating it into a larger system, such as a web application or an IoT platform, to detect patterns as the new data comes in.
Practical Example
Suppose you want to recognize patterns in temperature sensor data to predict sudden climate changes.
First, collect the temperature data over time.
Preprocess this data by addressing any outliers, missing values, and normalizing the values.
Using numpy and pandas, analyze the data to understand the main trends.
Apply feature extraction techniques to derive additional useful features that might indicate temperature change patterns.
Choose an algorithm, such as a Decision Tree, using scikit-learn, and train it with your data.
Evaluate the decision tree’s effectiveness, and once satisfactory results are obtained, deploy the model to a monitoring system that alerts users to significant climate changes.
Conclusion
Pattern recognition in sensor data using Python, scikit-learn, and numpy provides a comprehensive solution for identifying patterns in complex data sets.
By following a structured approach to data collection, preprocessing, model training, and deployment, one can achieve efficient and accurate results.
Utilizing these powerful Python libraries, individuals and organizations can harness the potential of their sensor data to make informed decisions and automate processes based on pattern recognition insights.
資料ダウンロード
QCD調達購買管理クラウド「newji」は、調達購買部門で必要なQCD管理全てを備えた、現場特化型兼クラウド型の今世紀最高の購買管理システムとなります。
ユーザー登録
調達購買業務の効率化だけでなく、システムを導入することで、コスト削減や製品・資材のステータス可視化のほか、属人化していた購買情報の共有化による内部不正防止や統制にも役立ちます。
NEWJI DX
製造業に特化したデジタルトランスフォーメーション(DX)の実現を目指す請負開発型のコンサルティングサービスです。AI、iPaaS、および先端の技術を駆使して、製造プロセスの効率化、業務効率化、チームワーク強化、コスト削減、品質向上を実現します。このサービスは、製造業の課題を深く理解し、それに対する最適なデジタルソリューションを提供することで、企業が持続的な成長とイノベーションを達成できるようサポートします。
オンライン講座
製造業、主に購買・調達部門にお勤めの方々に向けた情報を配信しております。
新任の方やベテランの方、管理職を対象とした幅広いコンテンツをご用意しております。
お問い合わせ
コストダウンが利益に直結する術だと理解していても、なかなか前に進めることができない状況。そんな時は、newjiのコストダウン自動化機能で大きく利益貢献しよう!
(Β版非公開)