- お役立ち記事
- Points of application to abnormality detection using sensor data acquisition/collection technology and artificial intelligence/AI
月間76,176名の
製造業ご担当者様が閲覧しています*
*2025年3月31日現在のGoogle Analyticsのデータより
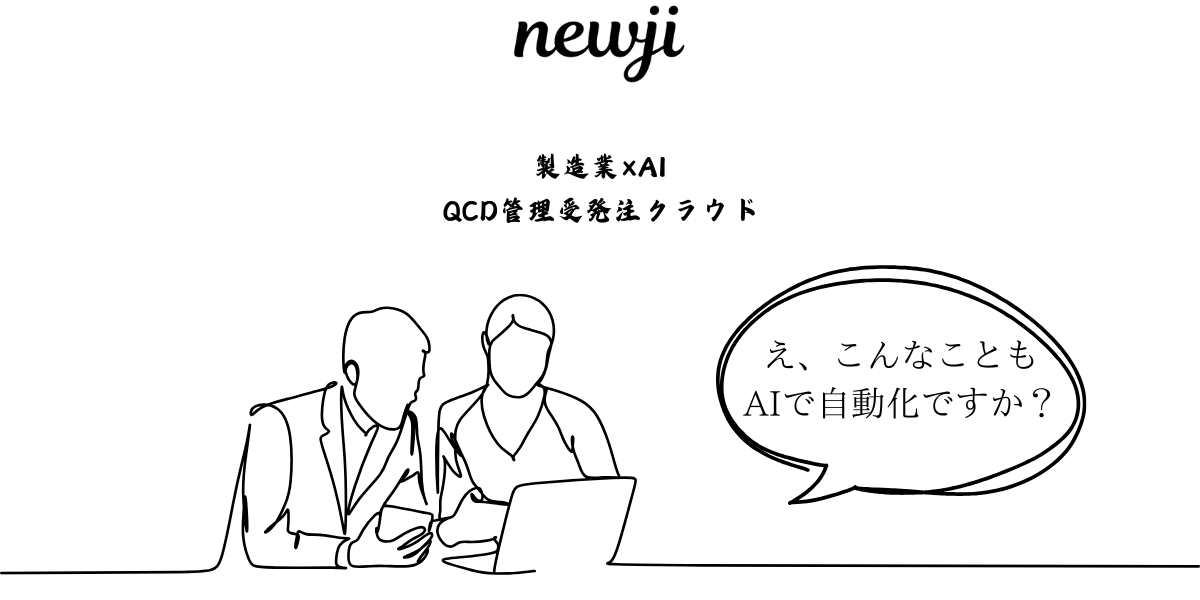
Points of application to abnormality detection using sensor data acquisition/collection technology and artificial intelligence/AI
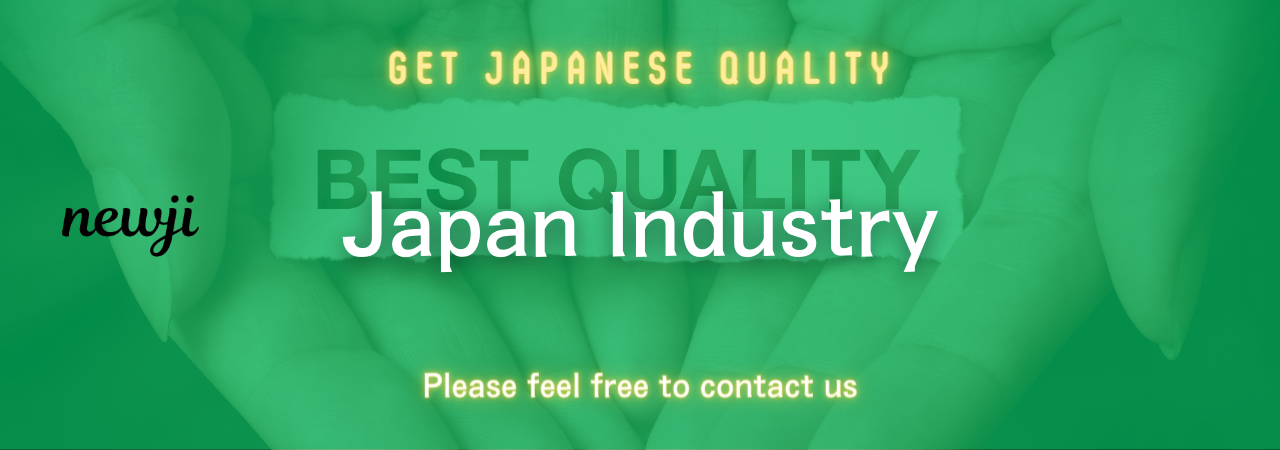
目次
Understanding Abnormality Detection
Abnormality detection, often referred to as anomaly detection, involves identifying patterns in a dataset that do not conform to expected behavior.
These anomalies can manifest in various forms, such as outliers in a cluster of homogeneous data or unexpected patterns in time series data.
In many industries, detecting anomalies is crucial because they can indicate significant, often critical issues.
For instance, anomalies might signify fraudulent activities in financial systems, or signal potential equipment failure in industrial settings.
The Role of Sensor Data Acquisition
Sensor data acquisition plays a pivotal role in abnormality detection.
With advancements in technology, a wide array of sensors is being utilized across different industries to collect data in real-time.
These sensors range from temperature and pressure sensors in industrial environments to biometric sensors in healthcare settings.
The first step in abnormality detection involves gathering an accurate and comprehensive set of data from these sensors.
Accurate data acquisition is essential because the quality of the collected data directly impacts the effectiveness of the anomaly detection process.
The data acquired from sensors is typically continuous and high-dimensional, presenting challenges in storage and processing.
However, once collected, this data can provide invaluable insights when processed and analyzed correctly.
Artificial Intelligence and Machine Learning
Artificial Intelligence (AI) and Machine Learning (ML) are transforming the field of abnormality detection.
These technologies are capable of learning from vast amounts of data, enabling the identification of patterns and deviations that might be imperceptible to human analysts.
AI-driven systems can be trained on historical data to understand what normal behavior looks like.
Once trained, these systems can continuously monitor incoming data for any anomalies.
Machine learning algorithms, such as neural networks, support vector machines, and clustering algorithms, are commonly used in this regard.
Neural networks, particularly deep learning models, have shown significant promise in detecting complex patterns in high-dimensional data.
They can be configured to automatically extract features from raw sensor data, improving the detection accuracy without the need for manual feature engineering.
Applications in Various Industries
Abnormality detection using sensor data and AI can be applied to a multitude of industries, each with its own unique challenges and benefits.
Manufacturing
In manufacturing, sensors are used extensively to monitor machinery and production lines.
Detecting anomalies in this environment can prevent costly equipment failures and downtime.
By analyzing sensor data, AI systems can predict when a piece of equipment might fail, allowing for proactive maintenance.
This application not only enhances production efficiency but also extends the life of machinery.
Healthcare
In healthcare, wearable devices and biometric sensors generate a vast amount of data related to a patient’s vital signs and activities.
Abnormality detection can alert healthcare professionals to irregularities in a patient’s health, enabling timely intervention.
For example, detecting anomalies in heart rate or oxygen levels can be critical for patients with chronic conditions.
Finance
In the finance industry, anomalies might indicate fraudulent transactions or data breaches.
AI systems can analyze transaction data in real-time to detect unusual spending patterns or unauthorized access.
This application can significantly enhance the security and trustworthiness of financial services.
Challenges and Considerations
While the combination of sensor data acquisition and AI presents a powerful tool for abnormality detection, it also comes with several challenges.
Data Quality and Volume
One of the primary challenges is ensuring the quality and volume of data.
Sensors must be calibrated and maintained to provide accurate data.
Moreover, the vast data generated needs efficient storage and processing capabilities.
Algorithm Selection and Training
Choosing the right machine learning algorithm is crucial, as different algorithms have varying strengths and are suited to different types of data.
The algorithm must be trained with a representative dataset to ensure it can accurately differentiate between normal and abnormal data.
Interpretability
The interpretability of AI models is crucial, especially where the results of anomaly detection influence critical decisions.
Stakeholders need to understand why a certain data point was flagged as anomalous.
Developing systems that provide a rationale for their decisions enhances trust and allows for better decision-making.
Future Trends
As technology continues to evolve, we can expect several advancements in this field.
Integration with IoT
The proliferation of the Internet of Things (IoT) will increase the number of sensors and the volume of data available for analysis.
This will enhance the capability to monitor and detect anomalies across various environments in real-time.
Advanced Algorithms
We will likely see the development of more sophisticated algorithms that can handle more complex datasets and provide more accurate anomaly detection.
These may include advancements in deep learning and reinforcement learning.
Edge Computing
With edge computing, data processing is performed closer to where it is generated.
This reduces latency and bandwidth issues, enabling faster detection and response to anomalies, particularly important in time-sensitive applications like autonomous driving or disaster response.
In conclusion, the integration of sensor data acquisition with AI holds immense potential for transforming how abnormalities are detected across various industries.
By continuing to overcome current challenges and embracing emerging technologies, the effectiveness and reliability of anomaly detection will only continue to improve.
資料ダウンロード
QCD管理受発注クラウド「newji」は、受発注部門で必要なQCD管理全てを備えた、現場特化型兼クラウド型の今世紀最高の受発注管理システムとなります。
ユーザー登録
受発注業務の効率化だけでなく、システムを導入することで、コスト削減や製品・資材のステータス可視化のほか、属人化していた受発注情報の共有化による内部不正防止や統制にも役立ちます。
NEWJI DX
製造業に特化したデジタルトランスフォーメーション(DX)の実現を目指す請負開発型のコンサルティングサービスです。AI、iPaaS、および先端の技術を駆使して、製造プロセスの効率化、業務効率化、チームワーク強化、コスト削減、品質向上を実現します。このサービスは、製造業の課題を深く理解し、それに対する最適なデジタルソリューションを提供することで、企業が持続的な成長とイノベーションを達成できるようサポートします。
製造業ニュース解説
製造業、主に購買・調達部門にお勤めの方々に向けた情報を配信しております。
新任の方やベテランの方、管理職を対象とした幅広いコンテンツをご用意しております。
お問い合わせ
コストダウンが利益に直結する術だと理解していても、なかなか前に進めることができない状況。そんな時は、newjiのコストダウン自動化機能で大きく利益貢献しよう!
(β版非公開)