- お役立ち記事
- Points to utilize failure data as reliability data
Points to utilize failure data as reliability data
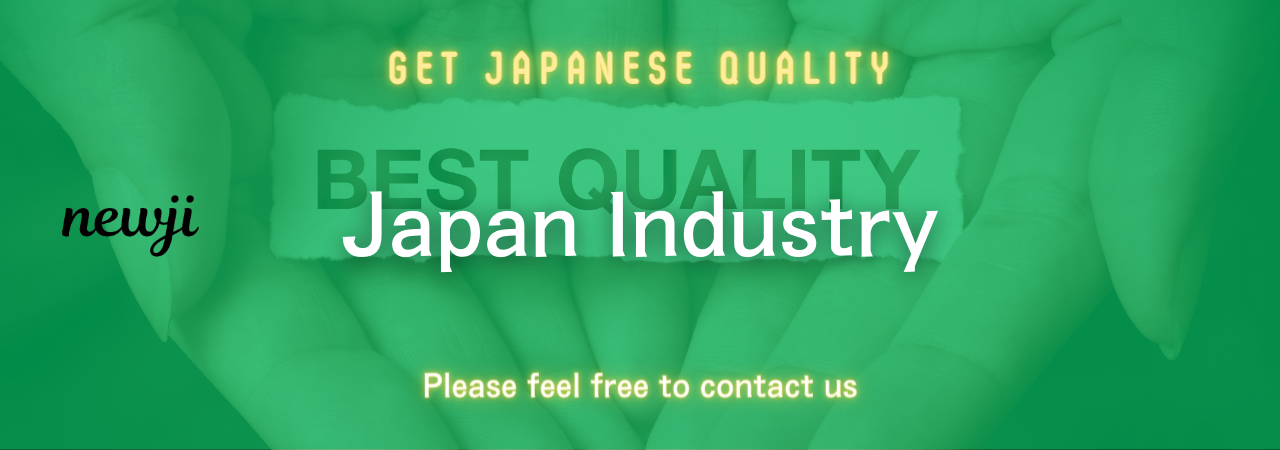
目次
Understanding Failure Data
Failure data refers to information collected from systems, processes, or products that have failed to perform as expected.
These failures can be technical malfunctions, software glitches, or any cessation of expected operations.
By analyzing this data, companies and researchers can glean insights into system weaknesses and potential areas for improvement.
However, using failure data as reliability data requires a strategic approach to ensure accurate and beneficial outcomes.
Why Use Failure Data?
Failure data offers a window into the real-world performance of systems under various conditions.
Every failure provides a lesson and an opportunity to correct past errors and enhance future performance.
Using failure data as reliability data helps organizations predict possible system failures, thereby allowing them to take proactive measures to prevent such issues.
By understanding the reasons for failures, companies can improve design, manufacturing, and operational processes, ultimately leading to more reliable products and services.
Collecting Comprehensive Failure Data
Before failure data can be effectively used for reliability assessment, it must be collected comprehensively and accurately.
This involves documenting every instance of failure, including minor ones, as they can collectively point to significant reliability issues.
Data collection should cover the specifics of the failure event: what happened, when it happened, under what conditions, and to what extent it affected the system.
Proper documentation ensures that no failure goes unnoticed and that patterns can be identified over time.
Using Automated Systems
Incorporating automated systems for data collection can significantly enhance the accuracy and efficiency of data gathering.
These systems can record events in real-time, reducing the chance of human error and providing immediate insights into any anomalies or failures.
By leveraging technology, companies can ensure that failure data is comprehensive and ready to be used for subsequent analysis.
Analyzing Failure Data for Reliability
Once the data has been collected, the next step is analysis.
This process involves examining the data to identify patterns and understand the root causes of failures.
Statistical methods such as trend analysis, root cause analysis, and failure mode effects analysis (FMEA) can be employed to gain a deeper understanding of the failure data.
These techniques help organizations determine the frequency and impact of different failures, allowing them to prioritize reliability improvements.
Identifying Trends and Patterns
Through proper analysis, failure data can reveal trends and patterns that may not be apparent at first glance.
For instance, certain failures may only occur under specific environmental conditions or when used in certain ways.
By recognizing these patterns, organizations can address the underlying issues more effectively.
Understanding trends also aids in forecasting potential failures, enabling preemptive action to be taken.
Implementing Improvements Based on Data
Once insights have been drawn from the failure data analysis, the next step is to implement changes aimed at improving reliability.
This could involve redesigning components, changing materials, optimizing manufacturing processes, or even altering how products are used.
The ultimate goal is to minimize the risk of future failures by removing identified weaknesses and improving the overall robustness of the system.
Feedback Loops for Continuous Improvement
Creating a feedback loop where improvements are continuously tested and refined is crucial for maintaining reliability.
By consistently monitoring the effects of implemented changes, organizations can ascertain whether their reliability improvements are effective or if further adjustments are necessary.
This ongoing process ensures that systems are as robust and reliable as possible, adapting to new challenges and evolving demands.
Challenges in Utilizing Failure Data
Despite its benefits, using failure data effectively can present several challenges.
These include the potential for incomplete data collection, difficulty in identifying root causes, and the risk of overcorrecting based on anomalous data.
Moreover, integrating failure data analysis into regular operational processes can be resource-intensive, requiring dedicated personnel and technologies.
Ensuring Data Quality
To overcome these challenges, it is essential to prioritize data quality.
This means ensuring that data collection processes are thorough and accurate, covering all potential points of failure.
Quality control measures, such as regular audits and validation checks, can help maintain high standards of data integrity.
The Role of Technology in Handling Failure Data
Technological advancements play a vital role in enhancing the utilization of failure data.
Tools like machine learning algorithms and artificial intelligence can automate the analysis process, providing faster and more accurate reliability insights.
These technologies can process vast amounts of data, identify subtle patterns, and suggest improvements that might not be evident through manual analysis.
Embracing Digital Twins
Digital twins, which are virtual models of physical systems, can be particularly useful in simulating failures and testing solutions.
By replicating real-world conditions, digital twins allow organizations to experiment with different scenarios without the risk of real-world consequences.
This proactive use of technology ensures that companies can respond swiftly and effectively to potential reliability issues.
Conclusion
In conclusion, utilizing failure data as reliability data is a powerful strategy for organizations aiming to improve their systems’ robustness and reduce risk.
By collecting comprehensive data, performing detailed analyses, and implementing strategic improvements, companies can transform failures into opportunities for growth and innovation.
Despite the challenges, the integration of reliable data collection and analysis methods, supported by advanced technologies, ensures that reliability insights are both actionable and effective.
By embracing this approach, organizations can not only meet but exceed their reliability objectives, delivering higher quality and more dependable products and services.
資料ダウンロード
QCD調達購買管理クラウド「newji」は、調達購買部門で必要なQCD管理全てを備えた、現場特化型兼クラウド型の今世紀最高の購買管理システムとなります。
ユーザー登録
調達購買業務の効率化だけでなく、システムを導入することで、コスト削減や製品・資材のステータス可視化のほか、属人化していた購買情報の共有化による内部不正防止や統制にも役立ちます。
NEWJI DX
製造業に特化したデジタルトランスフォーメーション(DX)の実現を目指す請負開発型のコンサルティングサービスです。AI、iPaaS、および先端の技術を駆使して、製造プロセスの効率化、業務効率化、チームワーク強化、コスト削減、品質向上を実現します。このサービスは、製造業の課題を深く理解し、それに対する最適なデジタルソリューションを提供することで、企業が持続的な成長とイノベーションを達成できるようサポートします。
オンライン講座
製造業、主に購買・調達部門にお勤めの方々に向けた情報を配信しております。
新任の方やベテランの方、管理職を対象とした幅広いコンテンツをご用意しております。
お問い合わせ
コストダウンが利益に直結する術だと理解していても、なかなか前に進めることができない状況。そんな時は、newjiのコストダウン自動化機能で大きく利益貢献しよう!
(Β版非公開)