- お役立ち記事
- Possibilities and Challenges of Utilizing Big Data in Manufacturing Quality Control
Possibilities and Challenges of Utilizing Big Data in Manufacturing Quality Control
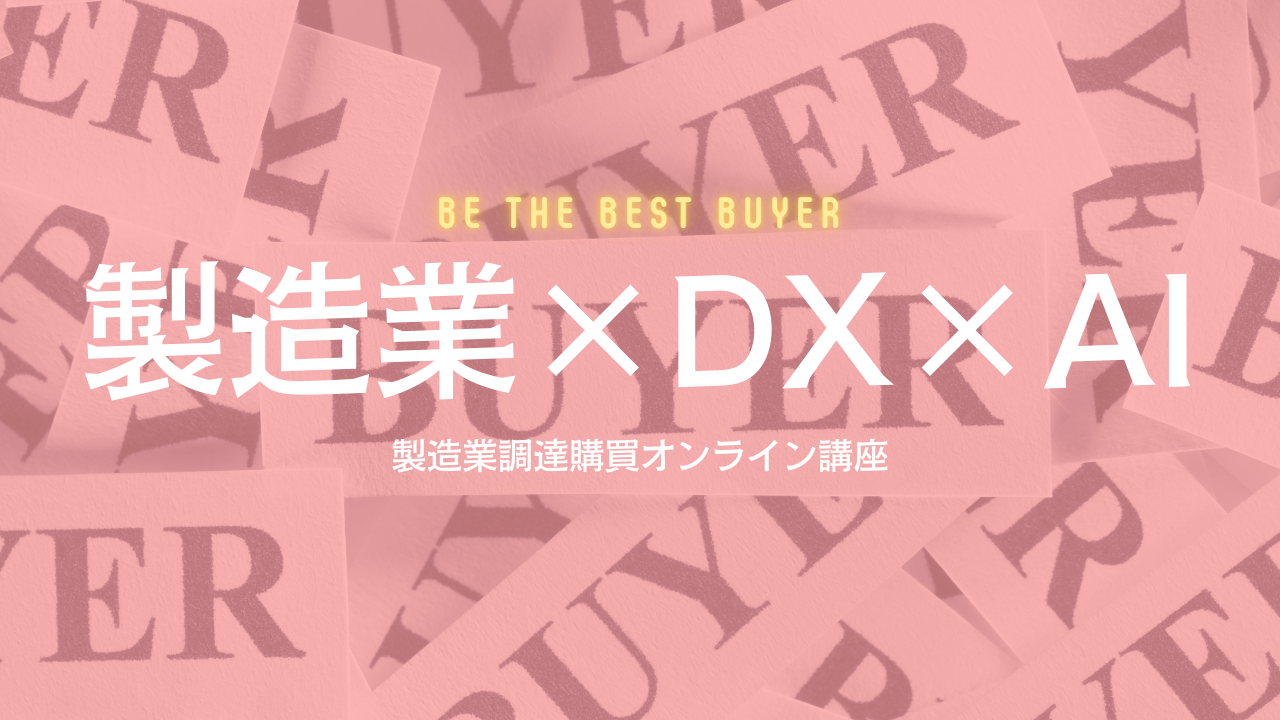
Big data and advanced analytics are revolutionizing quality control for manufacturers around the world. Vast amounts of information can now be collected from products, processes and customers to gain valuable insights and continuously improve. However, leveraging big data for quality also presents new technical, organizational and social challenges.
目次
Quality is Paramount in Manufacturing
Getting quality right is absolutely critical for manufacturers. Customers rightfully demand defect-free products that perform as expected. Issues can lead to recalls, replacement costs, lost sales and damaged brand reputation. Traditional quality control methods, like inspecting small samples, are no longer sufficient as product varieties and volumes increase. Manufacturers need a comprehensive and preventative approach.
This is where big data comes in. By capturing and analyzing data from every stage of the manufacturing process and product usage, patterns can be identified to address issues before they impact customers. Equipment sensors, internet-connected devices, customer feedback and warranty records all generate gigantic volumes of data each day. With the right tools and strategies, this wealth of information opens new possibilities for proactive quality control.
Collecting Comprehensive Manufacturing Data
The first step is collecting comprehensive operational and performance data. Sensor technology now allows manufacturers to monitor thousands of data points from machinery, tooling and production lines in real-time. Customers also generate substantial feedback through online reviews, surveys and connected product usage reports.
Bringing all this disparate data together into centralized repositories is a major technical challenge on its own. Manufacturers must decide what types of data provide the most value, from which sources, and store it in structured databases. Unstructured data like text reviews require natural language processing. Data standards and consistent formats are also essential to enable future analysis across systems.
Advanced Analytics for Deeper Insights
Once the appropriate data is centralized, advanced analytics can be applied. Approaches like machine learning, predictive modeling, big data mining and visualization techniques extract deeper insights. Patterns may reveal machinery issues impacting tolerances, areas prone to rework, or common product complaints. Suddenly weak spots within processes or design flaws become visible that were previously hidden.
Analytics also enable predictive quality by identifying early warning signs. Deviations detected can trigger investigations before serious problems emerge, allowing issues to be addressed proactively through process corrections or design adjustments. Recalls can even potentially be prevented with predictive predictions of field failures. As algorithms learn over time with more data, their accuracy and foresight capabilities improve.
Organizational Adaptation is Required
While technology enables new possibilities, organizational change is also crucial for manufacturers to extract full value from big data quality efforts. Silos between engineering, production, customer service and other functions must be broken down. Cross-functional “centres of excellence” bringing various expertise together optimizes insights.
Privacy, security and data governance also emerge as ongoing challenges as more customer and operational data are centralized. While quality goals require open data access, regulations demand controls. Manufacturers must thoughtfully navigate these social aspects of big data utilization. Overall, an analytical culture where data-driven decision making is pervasive at all levels delivers the greatest returns.
Remaining Barriers and The Road Ahead
Of course, the technical, economic and cultural shift required does not happen overnight. Budget and resource constraints, lack of analytics skills, and incompatible legacy systems present barriers. Data quality issues from inconsistent definitions, missing values or biases can also undermine results. Change management entails overcoming skepticism towards a proactive, collaborative big data driven approach.
Yet leaders who forge ahead gain tremendous competitive advantages through quality improvements and innovation capabilities not previously imagined. As analytics technologies continue advancing rapidly, what was considered aspirational even a few years ago will soon become commonplace best practices. For manufacturers committed to using data proactively, the future looks bright to deliver the consistent, high quality experiences customers demand while driving down costs through predictive maintenance and defect prevention. The opportunities of big data are waiting to be seized.
In summary, big data and advanced analytics open up exciting new avenues for proactive quality control if manufacturers can successfully collect comprehensive operational and customer information, apply the right techniques to extract actionable insights, and implement changes across their organizations. While challenges exist, those who adapt quickly to leverage the possibilities of big data will gain major competitive advantages in delivering defect-free products and services. With commitment to analytics-driven transformation, any issues can be addressed over time to realize this vision.
資料ダウンロード
QCD調達購買管理クラウド「newji」は、調達購買部門で必要なQCD管理全てを備えた、現場特化型兼クラウド型の今世紀最高の購買管理システムとなります。
ユーザー登録
調達購買業務の効率化だけでなく、システムを導入することで、コスト削減や製品・資材のステータス可視化のほか、属人化していた購買情報の共有化による内部不正防止や統制にも役立ちます。
NEWJI DX
製造業に特化したデジタルトランスフォーメーション(DX)の実現を目指す請負開発型のコンサルティングサービスです。AI、iPaaS、および先端の技術を駆使して、製造プロセスの効率化、業務効率化、チームワーク強化、コスト削減、品質向上を実現します。このサービスは、製造業の課題を深く理解し、それに対する最適なデジタルソリューションを提供することで、企業が持続的な成長とイノベーションを達成できるようサポートします。
オンライン講座
製造業、主に購買・調達部門にお勤めの方々に向けた情報を配信しております。
新任の方やベテランの方、管理職を対象とした幅広いコンテンツをご用意しております。
お問い合わせ
コストダウンが利益に直結する術だと理解していても、なかなか前に進めることができない状況。そんな時は、newjiのコストダウン自動化機能で大きく利益貢献しよう!
(Β版非公開)