- お役立ち記事
- Possibility of speech synthesis technology using deep learning to predict voice from face
月間77,185名の
製造業ご担当者様が閲覧しています*
*2025年2月28日現在のGoogle Analyticsのデータより
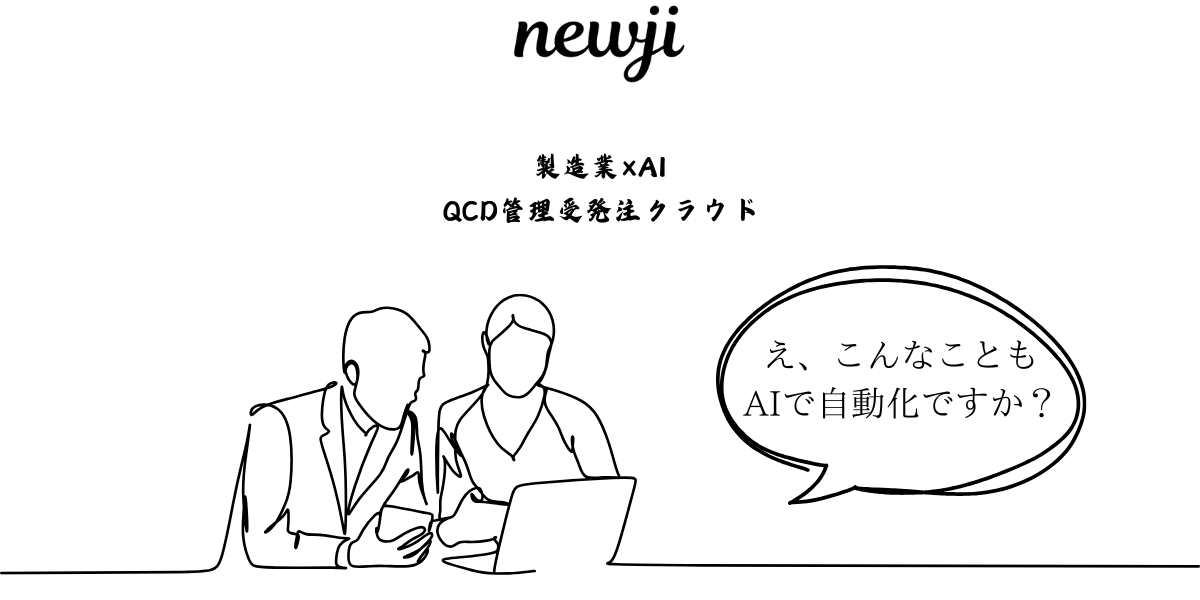
Possibility of speech synthesis technology using deep learning to predict voice from face
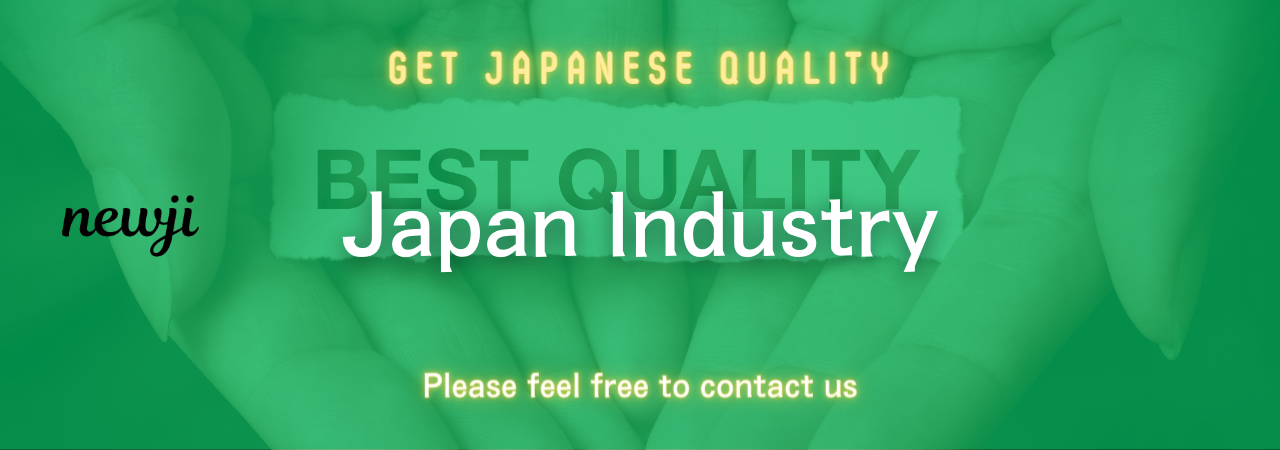
目次
Understanding Speech Synthesis Technology
Speech synthesis technology has made remarkable advancements over the years, and one of the most exciting developments in this field is the use of deep learning to predict voice from a person’s face.
This innovation has the potential to revolutionize how we interact with machines and understand speech patterns.
To grasp the possibilities, let’s explore what speech synthesis and deep learning are, and how they work together.
Speech synthesis, simply put, is the artificial production of human speech.
It involves creating a voice from text input, allowing computers and other devices to ‘speak.’
Traditionally, speech synthesis was done using rule-based methods, where phonetic rules were manually designed to convert text to speech.
However, with the advent of deep learning, these methods have become more sophisticated and natural-sounding.
Deep learning is a subset of machine learning, focusing on neural networks with multiple layers that allow computers to learn from vast amounts of data.
It mimics the human brain’s way of processing information, enabling machines to recognize patterns and make decisions.
In the context of speech synthesis, deep learning algorithms are trained on large datasets of recorded speech and images of faces to generate realistic-sounding voices.
How Deep Learning Predicts Voice from a Face
The process of predicting voice from a face using deep learning involves analyzing the visual cues from a person’s face, such as lip movements, facial expressions, and even subtle muscle twitches.
These cues contain valuable information regarding how a person speaks and their speech patterns.
By using a deep learning model, computers can correlate these visual features with speech data, effectively predicting how a person’s voice would sound.
The core of this technology is the use of neural networks that include convolutional layers to extract features from images of faces and recurrent layers to model the temporal aspects of speech.
Training a model to accurately predict voice involves feeding it pairs of facial images and the corresponding audio tracks.
Through millions of iterations, the model learns to associate certain facial movements and characteristics with specific sounds.
Applications of Predicting Voice from Face
The ability to accurately synthesize speech from facial images has far-reaching applications across various fields.
Let’s explore some of these exciting possibilities:
1. Communication for People with Disabilities
Individuals with speech impairments or conditions that limit their ability to vocalize can greatly benefit from this technology.
By using a camera to capture their facial movements, a synthesized voice can be generated, allowing them to communicate more effectively.
2. Enhancements in Virtual Reality and Gaming
In virtual environments, creating realistic characters with synchronized speech enhances the immersive experience.
Deep learning can enable virtual avatars to speak using voice predictions based on their designed facial features.
3. Security and Authentication
Voice prediction technology can be integrated into security systems to verify identities or detect deepfakes.
By comparing predicted voices to an established baseline, systems can identify inconsistencies or fraudulent activities.
4. Film and Animation
Filmmakers and animators can use this technology to synchronize dialogue in different languages.
It can also be used to adjust character speech during post-production without needing to re-record audio tracks.
Challenges and Considerations
While the potential of predicting voice from a face is promising, it also presents several challenges and considerations that need to be addressed.
1. Privacy Concerns
As with any technology involving biological characteristics, privacy is a major concern.
The use of personal facial and voice data must be handled with utmost care to prevent unauthorized use and ensure individuals’ privacy.
2. Accuracy and Bias
Deep learning models can sometimes inherit biases present in their training data.
If the training dataset is not sufficiently diverse, the model might not perform well across different ethnicities or genders, affecting the accuracy of voice predictions.
3. Ethical Implications
The ethical implications of generating synthetic voices need serious consideration.
Misuse of technology could lead to deceptive practices, such as creating misleading audio content that could harm individuals or groups.
The Future of Voice Synthesis and Deep Learning
The field of speech synthesis using deep learning to predict voice from a face is undoubtedly exciting and filled with potential.
As researchers continue to refine these models, we can expect even more accurate and nuanced voice predictions.
The future may see seamless integration of these technologies into everyday life, making communication more accessible and authentic.
Improvements in hardware, data collection, and model efficiency will drive further advancements in this space.
In conclusion, the combination of speech synthesis and facial recognition powered by deep learning is opening up new avenues for communication technology.
As we continue to navigate the challenges and capitalize on the possibilities, this innovation promises to enhance the way we interact with machines and each other.
資料ダウンロード
QCD管理受発注クラウド「newji」は、受発注部門で必要なQCD管理全てを備えた、現場特化型兼クラウド型の今世紀最高の受発注管理システムとなります。
ユーザー登録
受発注業務の効率化だけでなく、システムを導入することで、コスト削減や製品・資材のステータス可視化のほか、属人化していた受発注情報の共有化による内部不正防止や統制にも役立ちます。
NEWJI DX
製造業に特化したデジタルトランスフォーメーション(DX)の実現を目指す請負開発型のコンサルティングサービスです。AI、iPaaS、および先端の技術を駆使して、製造プロセスの効率化、業務効率化、チームワーク強化、コスト削減、品質向上を実現します。このサービスは、製造業の課題を深く理解し、それに対する最適なデジタルソリューションを提供することで、企業が持続的な成長とイノベーションを達成できるようサポートします。
製造業ニュース解説
製造業、主に購買・調達部門にお勤めの方々に向けた情報を配信しております。
新任の方やベテランの方、管理職を対象とした幅広いコンテンツをご用意しております。
お問い合わせ
コストダウンが利益に直結する術だと理解していても、なかなか前に進めることができない状況。そんな時は、newjiのコストダウン自動化機能で大きく利益貢献しよう!
(β版非公開)