- お役立ち記事
- Practical course on the basics of multivariate analysis and multiple regression analysis and key points for their use
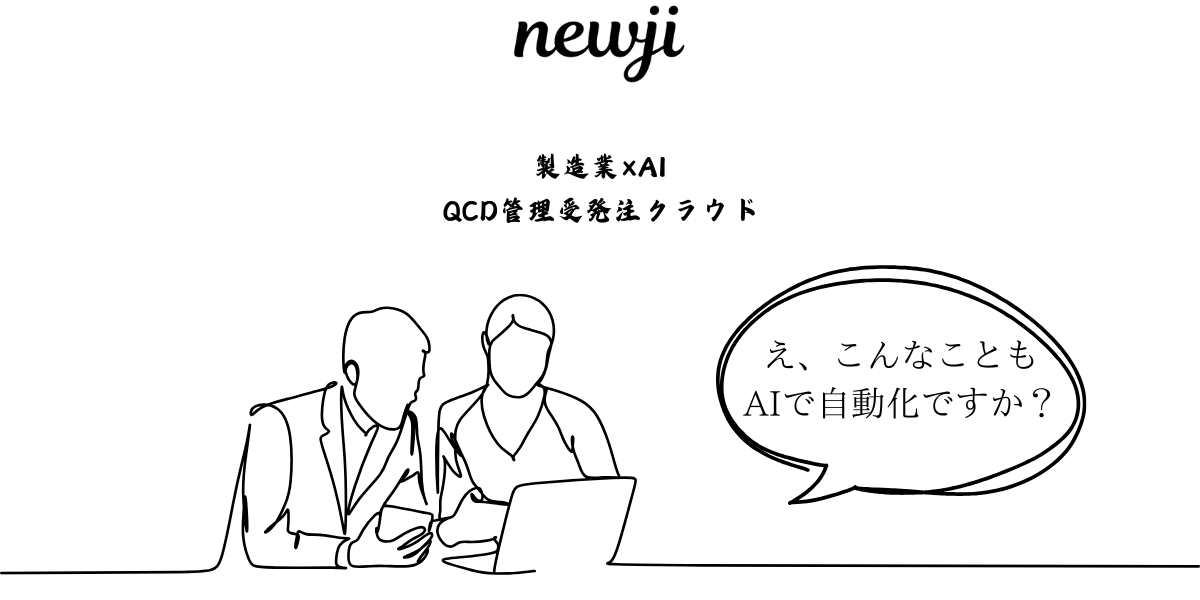
Practical course on the basics of multivariate analysis and multiple regression analysis and key points for their use
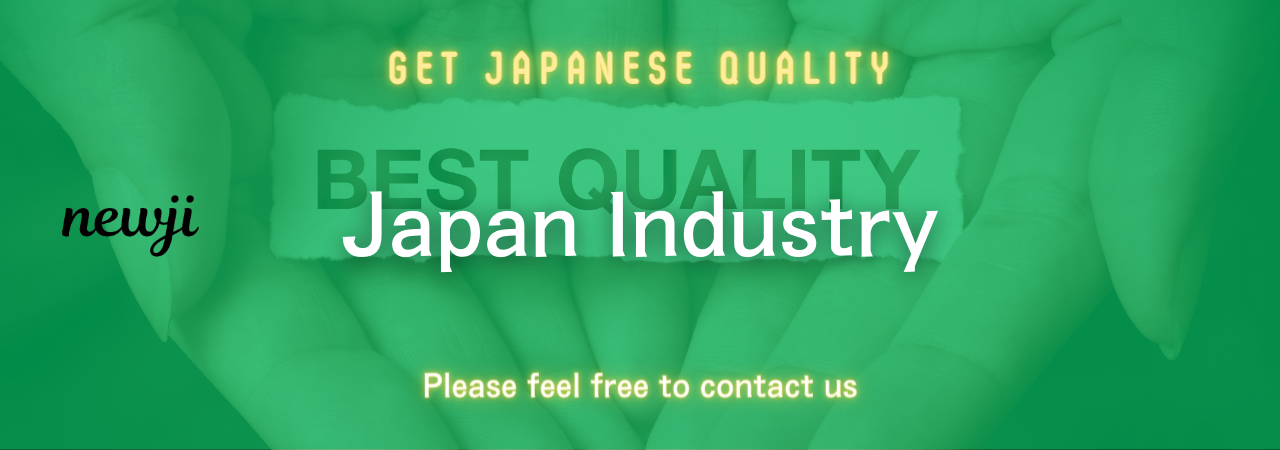
目次
Understanding Multivariate Analysis
Multivariate analysis is a statistical technique used to understand the relationships between multiple variables simultaneously.
It involves observation and analysis of more than one statistical outcome variable at a time.
This form of analysis is essential in various fields such as finance, social sciences, and marketing because it provides a more comprehensive view by considering the interaction between variables.
Multivariate analysis helps researchers and analysts derive insights that go beyond the limitations of univariate and bivariate analysis.
By incorporating multiple variables, this technique accounts for the complexity of real-world data, enabling more accurate predictions and better-informed decisions.
Essentially, it allows analysts to understand how variables relate to one another and how changes in one variable might affect others.
Types of Multivariate Analysis
There are several types of multivariate analysis, each suited for different kinds of data and research questions.
Some of the most commonly used types include:
1. Principal Component Analysis (PCA)
PCA is a technique used for dimensionality reduction.
It transforms a large set of variables into a smaller one that still contains most of the original information.
This reduction helps simplify data visualization and interpretation.
2. Factor Analysis
Similar to PCA, factor analysis is used for data reduction and structure detection.
It identifies underlying factors that explain the pattern of correlations within a set of observed variables.
3. Cluster Analysis
Cluster analysis is used to identify natural groupings in data.
By grouping similar data points together, it helps reveal internal patterns, such as customer segments or common characteristics.
4. Discriminant Analysis
Discriminant analysis is used to classify a set of observations into predefined classes.
It aids in identifying which variables discriminate between the different classes.
Basics of Multiple Regression Analysis
Multiple regression analysis is a statistical method used to model the relationship between a dependent variable and two or more independent variables.
This method extends simple linear regression, where only one independent variable is considered.
The fundamental purpose of multiple regression is to predict the value of the dependent variable based on the values of the independent variables.
This technique also helps in understanding the relative importance of each independent variable in the prediction.
Building a Regression Model
When constructing a regression model, the first step is to define the dependent and independent variables.
The dependent variable is the outcome you want to predict, while the independent variables are the predictors.
Next, data collection is crucial for building a robust model.
Accurate data that reflects the real-world scenario you want to analyze will lead to a reliable regression model.
After collecting data, it’s important to check for any outliers or anomalies that could affect the model’s accuracy.
Data cleaning ensures that the model’s predictions are not skewed by irregular data points.
Once the data is prepared, the regression model is fitted, meaning the statistical software calculates the relationship between the independent and dependent variables.
This involves estimating coefficients that quantify the impact of each independent variable.
Interpreting Regression Results
Interpreting the results of multiple regression involves understanding several key outputs.
The coefficients indicate the direction and magnitude of each variable’s impact on the dependent variable.
The p-value assesses the statistical significance of each coefficient.
A low p-value suggests that there is a statistically significant association between the independent and dependent variable.
The R-squared value provides a measure of how well the regression model fits the data.
A higher R-squared value indicates a better fit, meaning the model explains a greater proportion of the variance in the dependent variable.
Key Points for Applying Multivariate and Multiple Regression Analysis
1. Understand the Data
Before performing any analysis, it is crucial to have a deep understanding of the data at hand.
This involves knowing your variables, the type of data collected, and possible correlations and associations within the data set.
2. Assumptions Check
Both multivariate and multiple regression analysis rely on certain assumptions.
These include linearity, independence, homoscedasticity, and normality.
Testing these assumptions before analysis ensures the validity and reliability of the results.
3. Appropriate Selection of Variables
Choosing the right variables is essential for a meaningful analysis.
Irrelevant or redundant variables can lead to inaccurate models and misleading conclusions.
Use domain knowledge and statistical tests to select variables that genuinely impact the dependent variable.
4. Awareness of Multicollinearity
Multicollinearity occurs when independent variables are highly correlated with each other.
It can lead to unstable estimates and inflated standard errors.
It’s important to check for multicollinearity and address it through techniques like variable elimination or combining correlated variables.
5. Validation and Testing
After building the model, it’s crucial to validate it using techniques like cross-validation.
This kind of testing helps confirm that the model performs well on unseen data and not just the data it was trained on.
6. Interpretation and Application
Finally, effectively interpreting the results and applying them to decision-making is vital.
Ensure that stakeholders understand the insights derived from the analysis, as well as their implications for strategy and operations.
In conclusion, mastering multivariate and multiple regression analysis involves understanding the range of techniques available and their appropriate use cases.
By carefully preparing data, selecting variables, and validating models, analysts can extract valuable insights that drive better decision-making and strategic planning.
資料ダウンロード
QCD調達購買管理クラウド「newji」は、調達購買部門で必要なQCD管理全てを備えた、現場特化型兼クラウド型の今世紀最高の購買管理システムとなります。
ユーザー登録
調達購買業務の効率化だけでなく、システムを導入することで、コスト削減や製品・資材のステータス可視化のほか、属人化していた購買情報の共有化による内部不正防止や統制にも役立ちます。
NEWJI DX
製造業に特化したデジタルトランスフォーメーション(DX)の実現を目指す請負開発型のコンサルティングサービスです。AI、iPaaS、および先端の技術を駆使して、製造プロセスの効率化、業務効率化、チームワーク強化、コスト削減、品質向上を実現します。このサービスは、製造業の課題を深く理解し、それに対する最適なデジタルソリューションを提供することで、企業が持続的な成長とイノベーションを達成できるようサポートします。
オンライン講座
製造業、主に購買・調達部門にお勤めの方々に向けた情報を配信しております。
新任の方やベテランの方、管理職を対象とした幅広いコンテンツをご用意しております。
お問い合わせ
コストダウンが利益に直結する術だと理解していても、なかなか前に進めることができない状況。そんな時は、newjiのコストダウン自動化機能で大きく利益貢献しよう!
(Β版非公開)