- お役立ち記事
- Practical example of building an AI predictive model for purchasing department in material procurement
Practical example of building an AI predictive model for purchasing department in material procurement
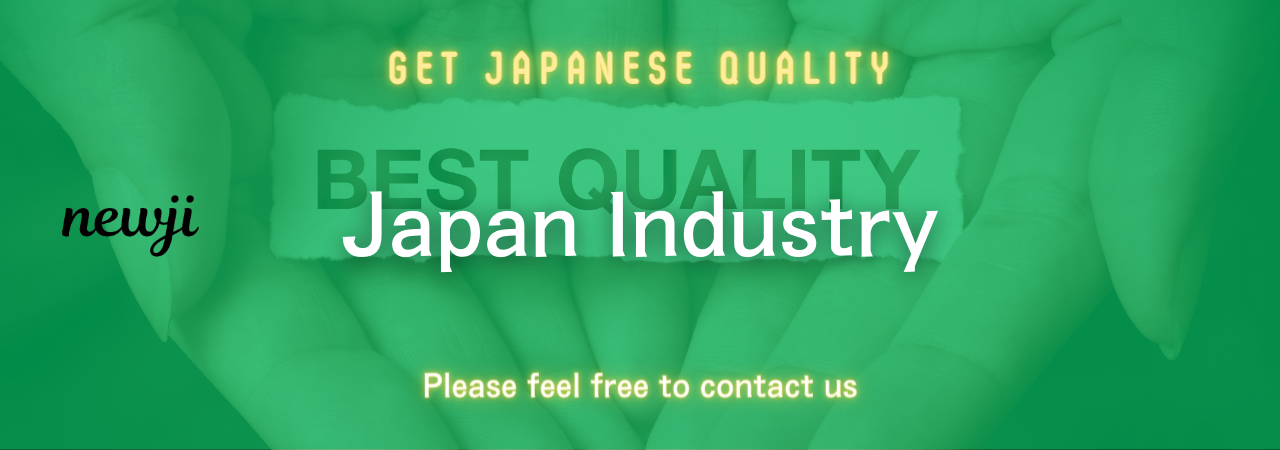
目次
Understanding AI Predictive Models
Predictive models are at the forefront of technological advancements, and when it comes to material procurement in the purchasing department, these models can be powerful tools in decision-making.
By leveraging historical data alongside AI algorithms, businesses can anticipate future needs, optimize costs, and manage resources more efficiently.
This practice not only reduces waste but also ensures that all supply chain components are functioning optimally.
In today’s competitive market, this can be a game-changer.
The Importance of AI in Material Procurement
AI predictive models provide invaluable insights that can be directly applied to procurement strategies.
They analyze vast data sets and predict potential market trends, highlight supplier reliability, and forecast material demand.
For purchasing departments, understanding these patterns means they can streamline procurement processes, reduce lead times, and negotiate better with suppliers.
By anticipating market demands and potential supply chain disruptions, AI ensures that necessary materials are always available when needed, without excess inventory costs.
Steps to Building an AI Predictive Model
Building an AI predictive model requires a structured approach.
Here’s a step-by-step process that purchasing departments can follow:
Step 1: Data Collection
The first step is gathering relevant data.
This information can come from various sources, such as historical purchase orders, supplier performance records, market trend reports, and inventory logs.
It’s crucial for the data to be accurate and comprehensive, as the quality of your data sets will directly impact the model’s effectiveness.
Step 2: Data Preprocessing
Once data is collected, the next phase is preprocessing.
During this stage, data is cleaned to remove any inconsistencies, duplicates, or irrelevant information.
Normalization and transformation may also be required to prepare the data for analysis.
The aim of preprocessing is to ensure that the AI model can efficiently process the data, leading to more accurate predictions.
Step 3: Selecting the Right Algorithm
Choosing the appropriate algorithm is critical for developing a successful model.
Common options include decision trees, linear regression, and neural networks, among others.
The choice of algorithm often depends on the specific needs of the procurement department and the nature of the datasets.
Experimenting with different algorithms during this stage can help find the one that delivers the best predictive accuracy.
Step 4: Training the Model
With the data prepared and an algorithm selected, the next step involves training the model.
This process requires feeding the algorithm with historical data, allowing it to recognize patterns and relationships within the data.
The algorithm uses these patterns to make predictions about future procurement needs.
It’s important to periodically test and validate the model to ensure its predictions remain accurate over time.
Step 5: Implementing the Model
Once trained and tested, the AI model can be implemented into the procurement process.
Integration with existing procurement systems allows for real-time data updates and seamless operations.
The model can now aid in decision-making, predict future material requirements, and streamline ordering processes.
Step 6: Continuous Monitoring and Improvement
After implementation, constant monitoring and updating are essential.
The purchasing department should regularly assess the model’s accuracy and incorporate any changes in supplier performance or market data.
Continuous refinement ensures that the model stays relevant and maintains its predictive capabilities in an ever-changing market.
Benefits of AI Predictive Models for Purchasing Departments
Integrating AI predictive models into the procurement process offers numerous advantages for purchasing departments.
Improved Forecast Accuracy
With data-driven insights, purchasing teams can make more informed and accurate forecasts regarding material requirements.
This leads to optimized inventory levels, reduced obsolescence, and lower carrying costs.
Enhanced Supplier Management
By analyzing historical supplier performance data, AI models can help identify the most reliable and cost-effective suppliers.
This capability allows purchasing departments to negotiate better terms and establish stronger supplier relationships.
Risk Mitigation
Predictive models can identify and alert teams to potential supply chain disruptions, enabling them to take proactive measures.
This foresight ensures that necessary materials are always available, minimizing the risk of project delays or stoppages.
Cost Savings
By optimizing order quantities and improving supplier negotiations, departments can significantly reduce their overall procurement costs.
Additionally, AI-driven insights can lead to better purchasing decisions, avoiding costly overstocking or shortages.
Challenges in Implementing AI Models
While the benefits are clear, implementing AI predictive models in material procurement also presents challenges.
Data Quality and Integrity
The accuracy of predictive models depends heavily on the quality of data fed into them.
Ensuring data integrity and reliability is a significant initial hurdle that departments must overcome.
Integration with Existing Systems
Seamless integration of new AI technologies with existing procurement software can be complex and may require significant IT resources.
Ensuring that all systems communicate effectively is crucial for successful implementation.
Change Management
Introducing AI models requires changes in established processes and practices.
Training staff and obtaining buy-in from all stakeholders are essential to overcome resistance and ensure smooth adoption.
In conclusion, building an AI predictive model for the purchasing department offers significant opportunities to improve efficiency and reduce costs in material procurement.
With careful planning and execution, these technologies can transform procurement strategies, delivering better outcomes for businesses.
By investing in AI models and overcoming implementation challenges, companies can gain a competitive advantage in an evolving market.
資料ダウンロード
QCD調達購買管理クラウド「newji」は、調達購買部門で必要なQCD管理全てを備えた、現場特化型兼クラウド型の今世紀最高の購買管理システムとなります。
ユーザー登録
調達購買業務の効率化だけでなく、システムを導入することで、コスト削減や製品・資材のステータス可視化のほか、属人化していた購買情報の共有化による内部不正防止や統制にも役立ちます。
NEWJI DX
製造業に特化したデジタルトランスフォーメーション(DX)の実現を目指す請負開発型のコンサルティングサービスです。AI、iPaaS、および先端の技術を駆使して、製造プロセスの効率化、業務効率化、チームワーク強化、コスト削減、品質向上を実現します。このサービスは、製造業の課題を深く理解し、それに対する最適なデジタルソリューションを提供することで、企業が持続的な成長とイノベーションを達成できるようサポートします。
オンライン講座
製造業、主に購買・調達部門にお勤めの方々に向けた情報を配信しております。
新任の方やベテランの方、管理職を対象とした幅広いコンテンツをご用意しております。
お問い合わせ
コストダウンが利益に直結する術だと理解していても、なかなか前に進めることができない状況。そんな時は、newjiのコストダウン自動化機能で大きく利益貢献しよう!
(Β版非公開)