- お役立ち記事
- Practical Guide to Effective Data Analysis Techniques for Advancing DX
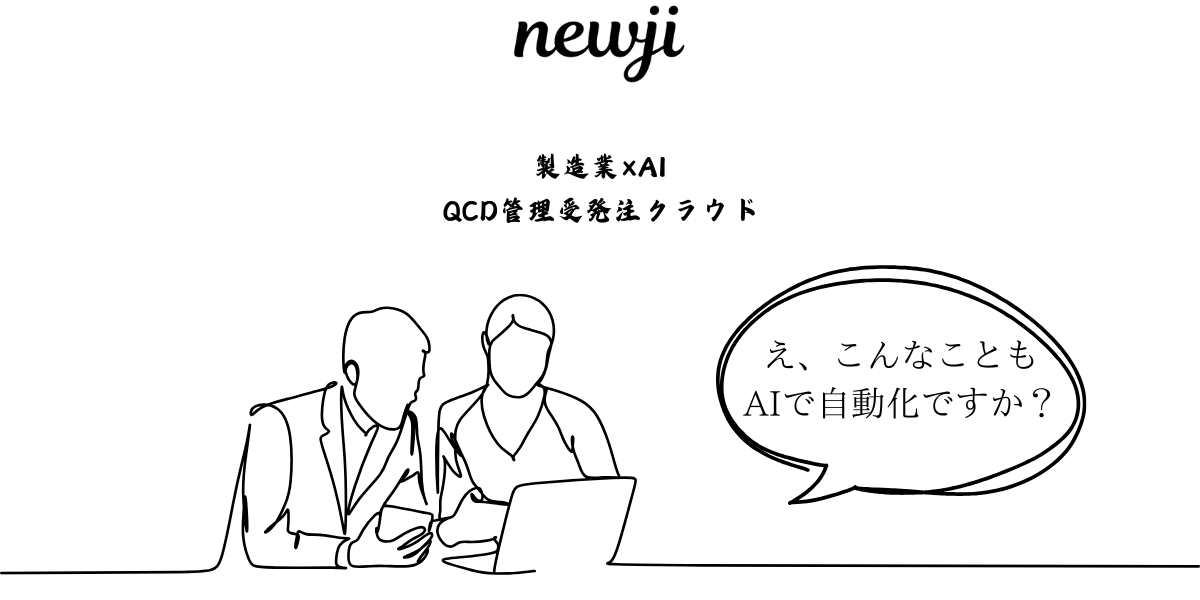
Practical Guide to Effective Data Analysis Techniques for Advancing DX
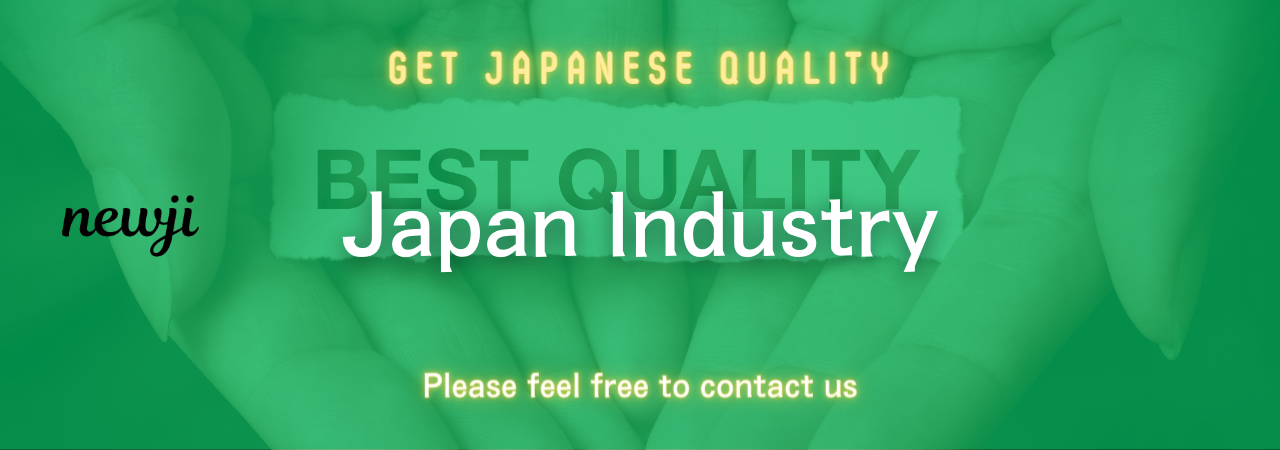
目次
Understanding Data Analysis in the Context of Digital Transformation
Data analysis plays a significant role in advancing digital transformation (DX).
It involves the process of inspecting, cleansing, transforming, and modeling data to discover useful information that can support decision-making.
In the context of DX, effective data analysis techniques enable organizations to leverage data for enhancing business operations, customer experiences, and creating new business models.
Today, businesses generate massive amounts of data from various digital touchpoints.
Harnessing this data is crucial for gaining insights needed to drive meaningful changes within an organization.
This guide will delve into effective data analysis techniques that can propel an organization’s digital transformation journey.
Data Collection: Laying the Groundwork
Before diving into analysis, it’s imperative to have a robust data collection strategy in place.
Data collection is the first step in data analysis and serves as the foundation for all subsequent processes.
Organizations need to gather data from different sources such as customer interactions, social media platforms, and IoT devices.
Ensuring that data collected is clean, accurate, and relevant is vital.
This involves using tools and technologies to eliminate duplicates, fill in missing information, and correct errors.
Clean data is easier to work with and leads to more reliable outcomes during the analysis phase.
Data Cleansing: Preparing for Insightful Analysis
Data cleansing is an essential process that ensures the integrity and quality of the data before analysis.
This step involves identifying and rectifying errors or inconsistencies in your data set.
Effective data cleansing techniques include:
– Removing duplicate entries to avoid biased results.
– Correcting inconsistencies like misspelled names or incorrect dates.
– Eliminating irrelevant data that does not contribute to the analysis objectives.
By maintaining high standards of data quality, businesses can draw more precise conclusions and make confident decisions based on their analyses.
Data Integration: Unifying Data Sources
Most organizations deal with data from multiple sources, which can vary in format and structure.
Data integration involves unifying these disparate data sources to create a comprehensive dataset suitable for analysis.
Having a centralized data repository, such as a data warehouse, can help streamline data integration efforts.
Data integration not only simplifies the analysis process but also provides a holistic view of the organization, allowing for more informed decision-making.
Adopting Advanced Data Integration Tools
Modern data integration tools utilize machine learning and artificial intelligence to automate and optimize the data unification process.
These tools help businesses maintain consistent, up-to-date datasets that can easily adapt to new data sources.
Exploratory Data Analysis (EDA): Discovering Patterns
EDA is a crucial phase in data analysis, allowing analysts to explore data sets and summarize their main characteristics using visual methods.
The goal is to uncover patterns, spot anomalies, and test hypotheses.
Techniques involved in EDA include:
– Visualizations like histograms, scatter plots, and box plots to represent data graphically.
– Summary statistics to provide a quick overview, such as mean, median, and mode.
– Identifying relationships between variables using correlation matrices.
Through EDA, organizations can gain a preliminary understanding of the data, guiding further analysis steps.
Predictive Analytics: Foreseeing Future Trends
Predictive analytics involves using statistical algorithms and machine learning techniques to analyze historical data and make predictions about future outcomes.
This type of analysis is invaluable for anticipating market trends, customer behavior, and potential risks.
Common predictive analytics techniques include:
– Regression analysis to model and analyze the relationships between variables.
– Time series analysis for forecasting future values based on past observations.
– Classification algorithms for categorizing data into predefined labels.
Implementing predictive analytics enables businesses to take preemptive actions based on data-driven insights.
Prescriptive Analytics: Recommending Actions
While predictive analytics focuses on predicting future trends, prescriptive analytics goes a step further by recommending actions to achieve desired outcomes.
This advanced form of analytics leverages optimization and simulation to suggest actions that could lead to favorable results.
Prescriptive analytics techniques include:
– Decision trees for evaluating possible action paths and their outcomes.
– Optimization models to determine the best solution from a set of alternatives.
– Scenario analysis for understanding the impact of various scenarios on business objectives.
Incorporating prescriptive analytics into the digital transformation strategy can lead to more effective and strategic decision-making.
Data Visualization: Making Data Intuitive
One of the most powerful ways to communicate complex data insights is through data visualization.
Effective visualizations translate data into a format that is easy for stakeholders to understand and interpret.
Common tools for data visualization include interactive dashboards, graphs, and heatmaps.
These visual elements not only enhance data interpretation but also facilitate quick decision-making.
Choosing the Right Visualization Techniques
The effectiveness of data visualization largely depends on selecting the right technique for the data being presented.
– Line charts are suitable for showing data trends over time.
– Pie charts are effective for illustrating proportions.
– Bar graphs are useful for comparing quantities.
By choosing the appropriate visualization technique, organizations can effectively convey stories within their data.
Measuring the Impact of Data Analysis on DX
To ensure that data analysis efforts contribute to digital transformation success, it’s crucial to measure their impact.
Key performance indicators (KPIs) should be established to track improvements and outcomes driven by data insights.
KPIs may include increased customer satisfaction scores, higher operational efficiency, or revenue growth.
Regularly assessing these metrics helps organizations understand the effectiveness of their data analysis techniques in driving DX.
Conclusion
In the digital age, data is a valuable asset that, when analyzed effectively, can drive significant advancements in digital transformation.
By employing techniques such as data cleansing, integration, exploratory analysis, and predictive analytics, organizations can unlock the potential of their data and turn it into actionable insights.
Implementing these practices not only enhances business operations and customer experience but also positions businesses ahead in the digital transformation journey.
As organizations continue to embrace digital transformation, robust data analysis techniques will remain pivotal to their success.
資料ダウンロード
QCD調達購買管理クラウド「newji」は、調達購買部門で必要なQCD管理全てを備えた、現場特化型兼クラウド型の今世紀最高の購買管理システムとなります。
ユーザー登録
調達購買業務の効率化だけでなく、システムを導入することで、コスト削減や製品・資材のステータス可視化のほか、属人化していた購買情報の共有化による内部不正防止や統制にも役立ちます。
NEWJI DX
製造業に特化したデジタルトランスフォーメーション(DX)の実現を目指す請負開発型のコンサルティングサービスです。AI、iPaaS、および先端の技術を駆使して、製造プロセスの効率化、業務効率化、チームワーク強化、コスト削減、品質向上を実現します。このサービスは、製造業の課題を深く理解し、それに対する最適なデジタルソリューションを提供することで、企業が持続的な成長とイノベーションを達成できるようサポートします。
オンライン講座
製造業、主に購買・調達部門にお勤めの方々に向けた情報を配信しております。
新任の方やベテランの方、管理職を対象とした幅広いコンテンツをご用意しております。
お問い合わせ
コストダウンが利益に直結する術だと理解していても、なかなか前に進めることができない状況。そんな時は、newjiのコストダウン自動化機能で大きく利益貢献しよう!
(Β版非公開)