- お役立ち記事
- Practical usage and implementation points for RAG construction and generation AI system
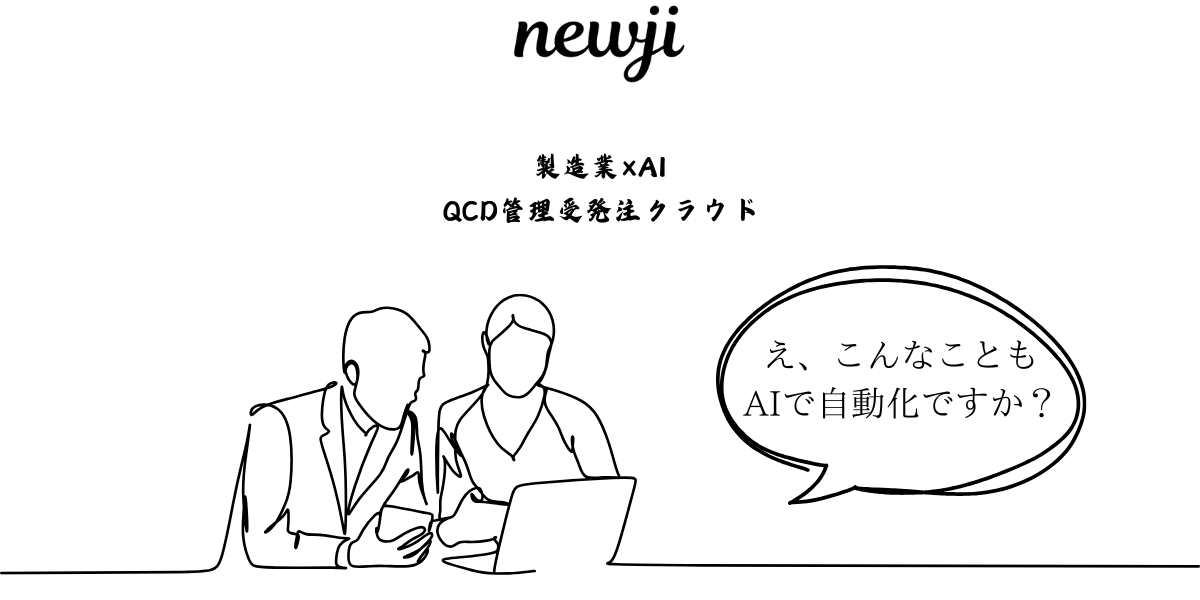
Practical usage and implementation points for RAG construction and generation AI system
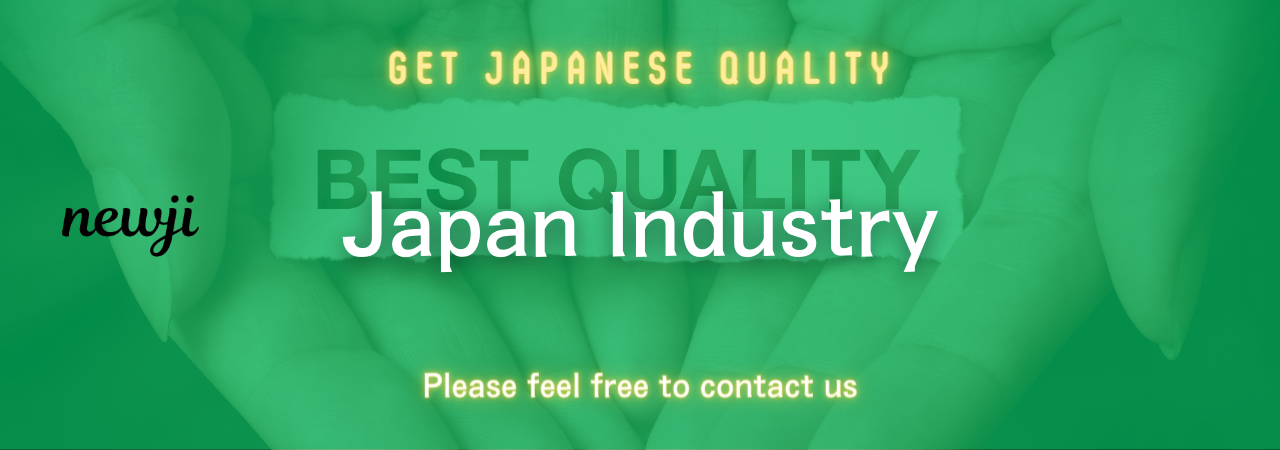
目次
Understanding RAG Construction
The rapid advancements in artificial intelligence have led to various forms of innovation across different industries.
One such innovation is the RAG (Retrieval-Augmented Generation) model, which combines retrieval-based methods with generative AI to provide more accurate and contextualized outputs.
But how exactly can one practically utilize this system, and what are the implementation points one should consider?
First off, understanding the RAG system is crucial.
RAG involves two main components: a retriever and a generator.
The retriever is responsible for fetching relevant pieces of information from a large dataset, while the generator crafts coherent and contextually appropriate responses based on the retrieved data.
This dynamic integration allows the AI to produce more informative and relevant responses compared to traditional AI models that rely solely on pre-learned data.
Practical Uses of RAG AI System
RAG models hold great potential in various fields.
One of the most resonant uses is in customer support systems.
When customers pose queries, the RAG model can search for relevant information across a company’s database and generate clear and precise answers.
This reduces response times and improves customer satisfaction.
In the field of content creation, RAG models can assist writers by providing suggestions based on a vast range of materials.
For instance, when writing articles, the system can retrieve relevant statistics, quotes, and previous research that can be seamlessly integrated into the content.
This augmentation enriches the content while saving time.
In educational settings, RAG systems can act as tutors, assisting students with their studies.
They are able to provide detailed answers to complex questions by retrieving valid educational resources and crafting them into understandable explanations.
This personalized help can boost learning efficiency.
Medical researchers can also leverage RAG models to source relevant scientific papers or studies quickly.
This can streamline the research process by enabling quicker access to summative information, which could potentially accelerate findings and developments.
Key Implementation Points for RAG AI Systems
Although implementing a RAG model sounds enticing, there are specific points to consider for effective deployment.
Data Source Selection
The backbone of the RAG model is the quality of the data it retrieves from.
Therefore, it’s essential to choose datasets that are accurate, update-to-date, and relevant to your domain.
Incorporating data scrupulously will ensure the system’s outputs are reliable and verifiable.
Fine-Tuning
Fine-tuning the retriever and generator components is crucial to optimize performance.
It involves adjusting parameters such that the system can prioritize contextually relevant information.
This can be achieved through a supervised training process which involves using a subset of your data to train the system.
Customizing for Context
While a RAG system is versatile, it’s paramount to customize its use to suit context-specific needs.
A medical RAG system should differ from one used in retail, both in terms of dataset and response style.
Tailoring its retrieval and generative mechanisms ensures that outputs are pertinent and worthy of the specific industry’s standards.
Challenges and Considerations
No technology comes without its challenges.
With RAG systems, one major consideration is maintaining data privacy and security.
With the model handling potentially sensitive information, it is crucial to implement robust security protocols to prevent unauthorized access.
Another challenge is to manage the computational load, as RAG systems can demand high processing power, especially when engaging with vast amounts of data.
This necessitates investments in solid infrastructure that can handle the rigorous processes without lagging.
Moreover, bias in data retrieval can pose an issue.
If the data sources have inherent biases, these can propagate into the RAG system’s outputs.
Thus, incorporating safeguards against biases through diverse datasets is vital.
Finally, it’s worth mentioning the importance of continuously updating and maintaining the system.
As information evolves, so should the datasets feeding into the RAG model.
Keeping the system relevant and accurate requires regular updates and checks.
Conclusion
Retrieval-Augmented Generation AI systems represent a leap forward in harnessing the full potential of AI-driven solutions.
By tactically combining retrieval and generative capabilities, RAG models can significantly enrich user experiences across various fields.
For successful implementation, stakeholders must consider data selection, fine-tuning, and contextual customization while being mindful of challenges like data bias, security, and computational demands.
With careful planning and execution, RAG AI systems can transform industries and the way information is consumed and applied.
In a world increasingly reliant on AI, understanding and leveraging technologies like RAG can set a foundation for more intelligent and responsive systems.
資料ダウンロード
QCD調達購買管理クラウド「newji」は、調達購買部門で必要なQCD管理全てを備えた、現場特化型兼クラウド型の今世紀最高の購買管理システムとなります。
ユーザー登録
調達購買業務の効率化だけでなく、システムを導入することで、コスト削減や製品・資材のステータス可視化のほか、属人化していた購買情報の共有化による内部不正防止や統制にも役立ちます。
NEWJI DX
製造業に特化したデジタルトランスフォーメーション(DX)の実現を目指す請負開発型のコンサルティングサービスです。AI、iPaaS、および先端の技術を駆使して、製造プロセスの効率化、業務効率化、チームワーク強化、コスト削減、品質向上を実現します。このサービスは、製造業の課題を深く理解し、それに対する最適なデジタルソリューションを提供することで、企業が持続的な成長とイノベーションを達成できるようサポートします。
オンライン講座
製造業、主に購買・調達部門にお勤めの方々に向けた情報を配信しております。
新任の方やベテランの方、管理職を対象とした幅広いコンテンツをご用意しております。
お問い合わせ
コストダウンが利益に直結する術だと理解していても、なかなか前に進めることができない状況。そんな時は、newjiのコストダウン自動化機能で大きく利益貢献しよう!
(Β版非公開)