- お役立ち記事
- Prediction of future events from multidimensional time series data
月間76,176名の
製造業ご担当者様が閲覧しています*
*2025年3月31日現在のGoogle Analyticsのデータより
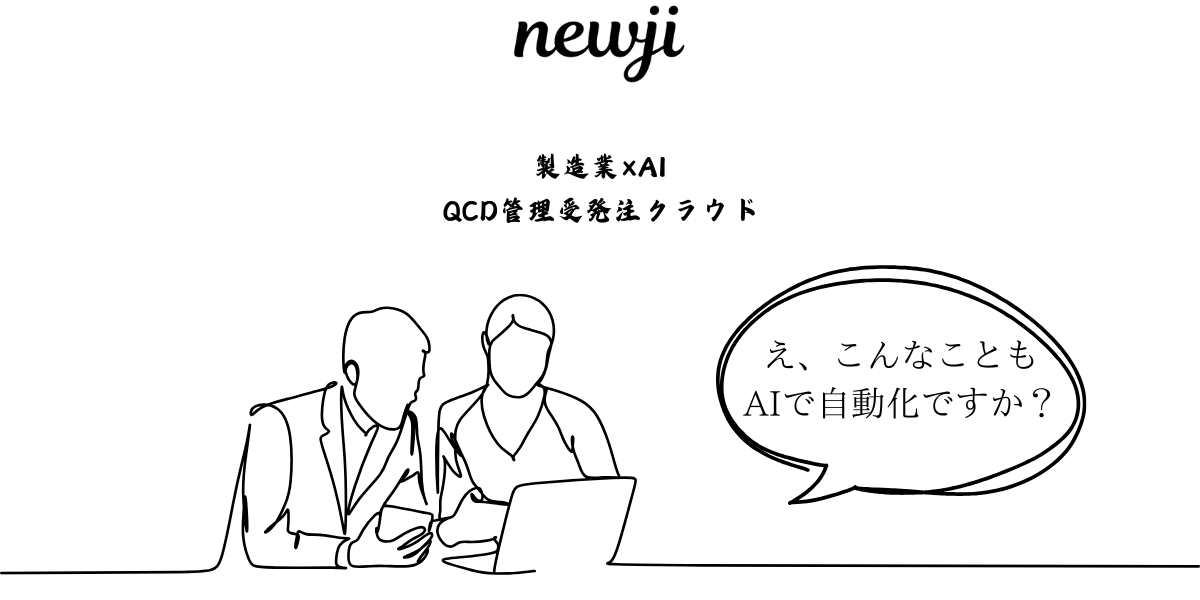
Prediction of future events from multidimensional time series data
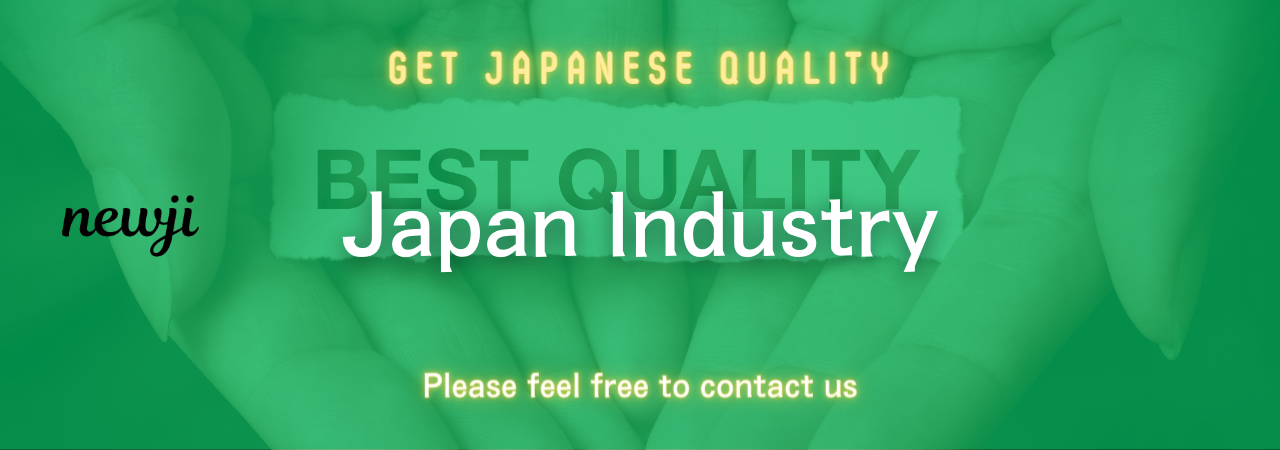
目次
Understanding Time Series Data
Time series data is an essential concept in understanding our world and predicting future events.
It consists of a sequence of data points collected or recorded at specific time intervals.
For example, daily temperature readings, stock market prices, and website traffic statistics are all forms of time series data.
By analyzing these data points, we can see patterns, trends, and cycles that help us understand how things have changed over time and make predictions about the future.
What are Multidimensional Time Series?
Multidimensional time series data is a more complex form of time series data, where we have multiple variables recorded at each time point.
Instead of tracking a single variable like temperature, multidimensional time series might simultaneously track temperature, humidity, and wind speed.
This added complexity allows for a more detailed and comprehensive analysis, as each variable may interact and influence the others.
The Importance of Multidimensional Data
Analyzing multidimensional time series data helps us capture the intricate relationships between different variables.
For example, in financial markets, prices, volumes, and volatility are all interconnected.
Understanding these relationships can provide a significant advantage in predicting future movements and developing strategies.
Techniques for Analyzing Time Series Data
Predicting future events from multidimensional time series data can be a challenging task.
However, several techniques are widely used to analyze and forecast from such data.
Statistical Models
Traditional statistical models, like ARIMA (AutoRegressive Integrated Moving Average), are often used for time series forecasting.
These models rely on patterns within the data, like trends and seasonality, to make predictions.
In multidimensional time series, vector autoregression (VAR) models are particularly useful, as they can handle multiple time-dependent variables simultaneously.
Machine Learning Approaches
Machine learning techniques have gained popularity in time series forecasting, especially with multidimensional data.
Algorithms like Support Vector Machines (SVM) and Random Forests can be employed to identify complex patterns.
Neural networks, particularly Long Short-Term Memory (LSTM) models, excel in capturing dependencies across time and multiple variables, making them ideal for multidimensional series forecasting.
Deep Learning Methods
Deep learning offers advanced techniques like convolutional neural networks (CNNs) and LSTMs.
These models can detect patterns in high-dimensional datasets, unveiling intricate structures that traditional models might miss.
Deep learning approaches can significantly improve prediction accuracy, especially when dealing with vast and complex datasets.
Applications of Multidimensional Time Series Predictions
Predicting future events using multidimensional time series data has a wide range of applications across various fields.
Weather Forecasting
Weather predictions are one of the most well-known applications of time series data.
Meteorologists use multidimensional time series data, including temperature, humidity, wind speed, and atmospheric pressure, to forecast weather conditions accurately.
These predictions are crucial for planning and safety purposes, from agriculture to aviation.
Financial Market Analysis
In finance, predicting stock prices, commodities, and currency exchange rates involves complex multidimensional time series analysis.
Traders and investors rely on these predictions to make informed decisions and optimize their portfolios.
Using methods like machine learning and deep learning can boost the efficiency and accuracy of such financial predictions.
Healthcare Monitoring
In healthcare, multidimensional time series data can track a patient’s vital signs over time, such as heart rate, blood pressure, and temperature.
Analyzing this data helps doctors make timely interventions and predict potential health risks.
This application is crucial for patients with chronic conditions requiring constant monitoring.
Challenges in Time Series Predictions
While predicting future events using multidimensional time series data holds great promise, it comes with its challenges.
High Dimensionality
Handling data with many dimensions can lead to computational complexity and the risk of overfitting, where a model becomes too adapted to the training data and less effective at predicting unseen data.
Data Quality
Poor-quality data, such as missing values or noise, can severely impact the accuracy of predictions.
Ensuring data quality is a critical step in the preprocessing phase.
Understanding Complexity
The relationships between variables in multidimensional time series can be highly complex, making it challenging to identify causal links.
Interpreting these relationships is often as important as the predictions.
The Future of Time Series Forecasting
Even with its challenges, multidimensional time series forecasting is an evolving field with growing importance in our data-driven world.
As technology advances, we can expect more sophisticated models and computational techniques that enable faster and more accurate predictions.
Artificial intelligence, with its integration of machine learning and deep learning, promises to lead significant breakthroughs in predicting future events from multidimensional time series data.
These advancements will open up new possibilities for innovation across various fields, from cutting-edge financial technologies to personalized healthcare solutions.
Fostering a continuous improvement mindset within this domain will broaden our understanding of complex systems, helping us make better-informed decisions and anticipate future developments with greater accuracy.
資料ダウンロード
QCD管理受発注クラウド「newji」は、受発注部門で必要なQCD管理全てを備えた、現場特化型兼クラウド型の今世紀最高の受発注管理システムとなります。
ユーザー登録
受発注業務の効率化だけでなく、システムを導入することで、コスト削減や製品・資材のステータス可視化のほか、属人化していた受発注情報の共有化による内部不正防止や統制にも役立ちます。
NEWJI DX
製造業に特化したデジタルトランスフォーメーション(DX)の実現を目指す請負開発型のコンサルティングサービスです。AI、iPaaS、および先端の技術を駆使して、製造プロセスの効率化、業務効率化、チームワーク強化、コスト削減、品質向上を実現します。このサービスは、製造業の課題を深く理解し、それに対する最適なデジタルソリューションを提供することで、企業が持続的な成長とイノベーションを達成できるようサポートします。
製造業ニュース解説
製造業、主に購買・調達部門にお勤めの方々に向けた情報を配信しております。
新任の方やベテランの方、管理職を対象とした幅広いコンテンツをご用意しております。
お問い合わせ
コストダウンが利益に直結する術だと理解していても、なかなか前に進めることができない状況。そんな時は、newjiのコストダウン自動化機能で大きく利益貢献しよう!
(β版非公開)