- お役立ち記事
- Predictive maintenance using edge AI
月間76,176名の
製造業ご担当者様が閲覧しています*
*2025年3月31日現在のGoogle Analyticsのデータより
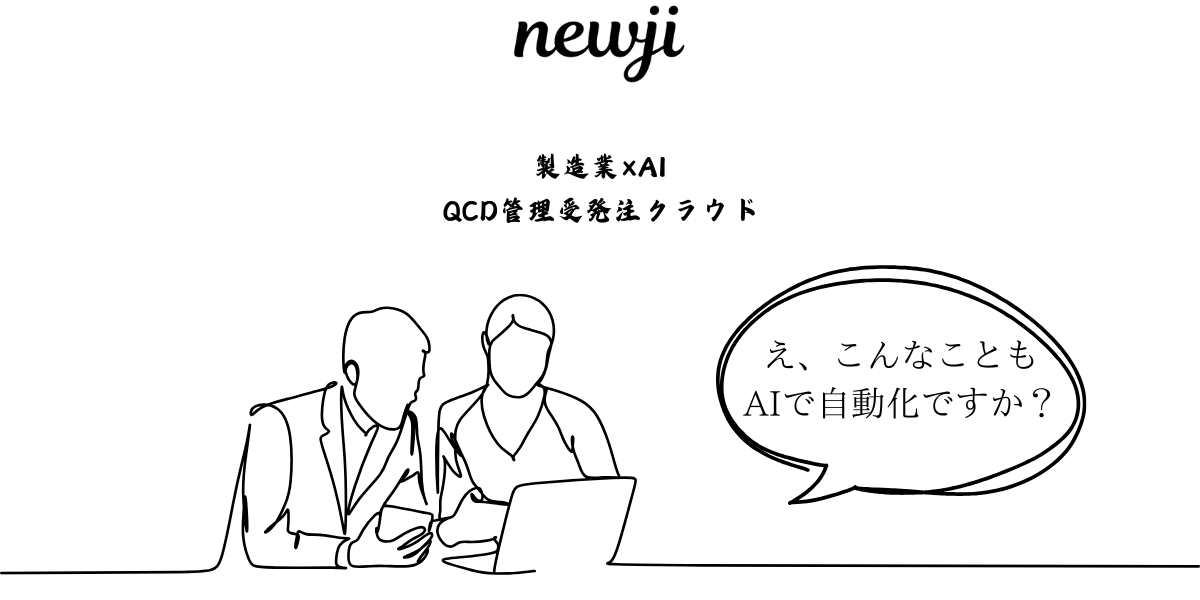
Predictive maintenance using edge AI
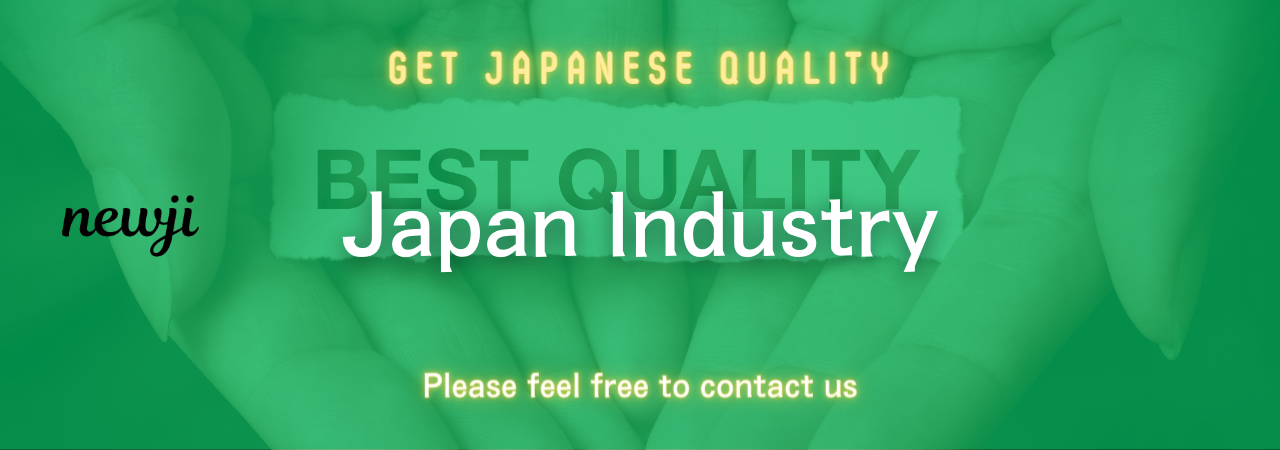
目次
Understanding Predictive Maintenance
Predictive maintenance is a proactive approach aimed at anticipating equipment failures before they occur.
This strategy relies on data analysis and advanced algorithms to predict when maintenance should be performed.
Traditionally, maintenance would follow a reactive or scheduled approach, with reactive focusing on fixing issues after they arise, and scheduled based on a fixed timeline.
Predictive maintenance stands out by allowing maintenance to be performed precisely when needed, reducing downtime and optimizing operational efficiency.
In recent years, edge AI has emerged as a groundbreaking technology that takes predictive maintenance to a whole new level.
Edge AI refers to computational intelligence placed at the “edge” of the network, closer to where data is generated.
This enables real-time data processing and analytics directly at the source.
How Edge AI Enhances Predictive Maintenance
The integration of edge AI into predictive maintenance systems offers numerous advantages.
Firstly, it drastically reduces latency since data does not need to be transmitted to a centralized server for processing.
Instead, the data is analyzed right at the source, providing instant insights and facilitating timely decision-making.
Another advantage is the enhanced data security.
When data is processed at the edge, there is minimal need to send sensitive information over the network.
This local processing reduces the risk of data breaches and ensures the security of critical operational data.
Moreover, edge AI enables continuous monitoring and analysis of equipment.
This continuous insight allows maintenance teams to identify trends and patterns that could indicate potential failures.
By addressing these issues promptly, organizations can minimize unplanned downtime and extend the lifespan of their machinery.
Real-World Applications of Edge AI in Predictive Maintenance
A wide range of industries can benefit from predictive maintenance through edge AI.
In the manufacturing sector, machines are equipped with sensors that gather critical data on temperature, vibration, and noise levels.
Edge AI analyzes this data to detect any anomalies and predict equipment failures.
This proactive approach allows manufacturers to maintain smooth operations and reduce maintenance costs.
Similarly, in the energy sector, predictive maintenance helps prevent unplanned outages and optimizes equipment life cycles.
For example, wind turbines can be monitored using edge AI to analyze vibration data for early signs of wear.
Timely maintenance can then be conducted, preventing costly failures and ensuring a stable power supply.
In the field of transportation, edge AI can be applied in vehicles to monitor engine performance and other critical components.
This smart monitoring allows fleet operators to pre-emptively address issues, improving fleet reliability and reducing repair costs.
Integration of IoT and Edge AI
The Internet of Things (IoT) plays a crucial role in harnessing the power of predictive maintenance with edge AI.
IoT devices provide the data collection infrastructure necessary for edge AI to function effectively.
Sensors embedded in machinery or equipment continuously collect data, which can then be analyzed by edge AI systems.
The synergy between IoT and edge AI results in highly efficient predictive maintenance systems.
IoT provides the data, while edge AI processes and analyzes it rapidly to deliver actionable insights.
This combination allows for real-time monitoring and decision-making, improving overall operational efficiency.
Challenges in Implementing Edge AI for Predictive Maintenance
Despite its numerous benefits, implementing edge AI for predictive maintenance does come with challenges.
One significant challenge is the initial cost of deployment.
Equipping machines with the necessary sensors and edge AI hardware can be costly, though the long-term cost savings often justify the investment.
Data management is another challenge.
With sensors continuously collecting data, organizations need to have appropriate storage solutions and data management processes in place.
This ensures that the edge AI algorithms have access to quality data for accurate predictions.
Ensuring compatibility between existing systems and new edge AI technology can also be complex.
Organizations may face difficulties in integrating edge AI with their current infrastructure, necessitating careful planning and potentially further investments.
Future Prospects of Edge AI in Predictive Maintenance
The future of predictive maintenance with edge AI is promising.
As technology continues to advance, we can expect more efficient and cost-effective solutions.
Edge AI will become increasingly accessible to a broader range of industries, enabling smaller businesses to benefit from its capabilities.
Advancements in AI algorithms will likely lead to more accurate predictions and faster processing times.
This will further enhance the reliability of predictive maintenance systems, reducing downtime and increasing productivity.
Moreover, the ongoing development of 5G technology will benefit edge AI by providing faster and more reliable data transmission.
This will make it even more feasible for real-time data processing at the edge, cementing edge AI’s role in predictive maintenance strategies.
Conclusion
Predictive maintenance using edge AI represents a significant advancement in maintenance strategies.
By processing data at the edge, organizations can make timely, informed decisions that optimize equipment performance, reduce costs, and minimize downtime.
While challenges exist, the benefits of implementing edge AI for predictive maintenance far outweigh the drawbacks.
As technology progresses, we can expect even more effective solutions, shaping the future of maintenance practices across various industries.
Embracing predictive maintenance with edge AI is a strategic move that can lead to more reliable operations and a competitive advantage in today’s fast-paced, technology-driven world.
資料ダウンロード
QCD管理受発注クラウド「newji」は、受発注部門で必要なQCD管理全てを備えた、現場特化型兼クラウド型の今世紀最高の受発注管理システムとなります。
ユーザー登録
受発注業務の効率化だけでなく、システムを導入することで、コスト削減や製品・資材のステータス可視化のほか、属人化していた受発注情報の共有化による内部不正防止や統制にも役立ちます。
NEWJI DX
製造業に特化したデジタルトランスフォーメーション(DX)の実現を目指す請負開発型のコンサルティングサービスです。AI、iPaaS、および先端の技術を駆使して、製造プロセスの効率化、業務効率化、チームワーク強化、コスト削減、品質向上を実現します。このサービスは、製造業の課題を深く理解し、それに対する最適なデジタルソリューションを提供することで、企業が持続的な成長とイノベーションを達成できるようサポートします。
製造業ニュース解説
製造業、主に購買・調達部門にお勤めの方々に向けた情報を配信しております。
新任の方やベテランの方、管理職を対象とした幅広いコンテンツをご用意しております。
お問い合わせ
コストダウンが利益に直結する術だと理解していても、なかなか前に進めることができない状況。そんな時は、newjiのコストダウン自動化機能で大きく利益貢献しよう!
(β版非公開)