- お役立ち記事
- Probabilistic graphical models and their application to causal inference
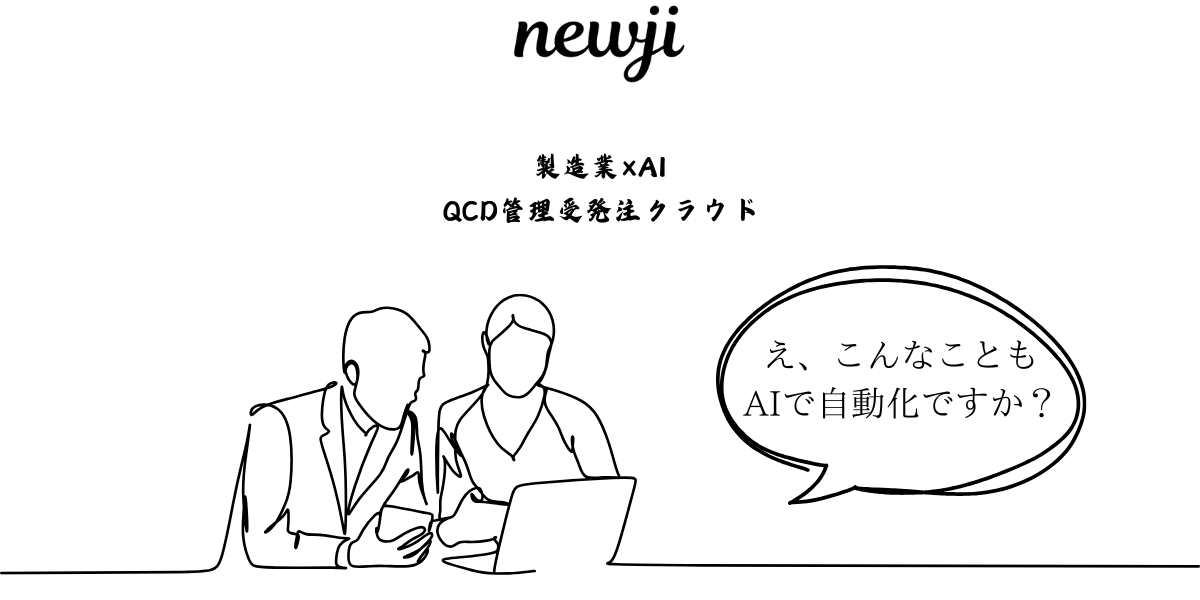
Probabilistic graphical models and their application to causal inference
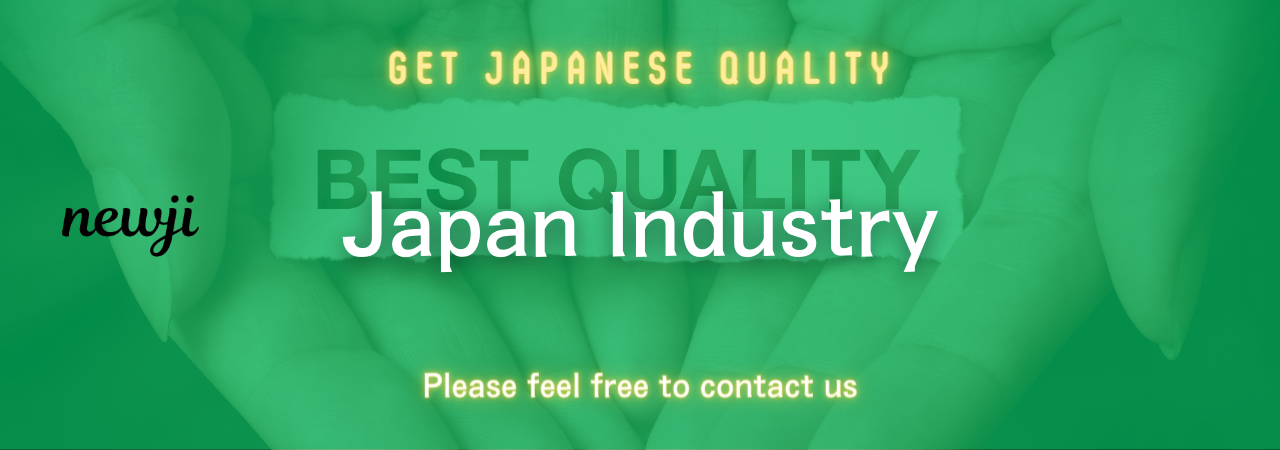
目次
Understanding Probabilistic Graphical Models
Probabilistic graphical models (PGMs) are a powerful tool in the field of statistics and machine learning.
They provide a way to represent complex distributions and uncertain systems.
At their core, PGMs use a graph-based representation that makes it easier to visualize the dependencies and relationships between random variables.
In essence, PGMs help us understand the relationship between different variables in a dataset.
They combine the principles of probability theory and graph theory into a single framework.
This allows us to model complex systems in a structured way, making them particularly useful for tasks like prediction, diagnosis, and decision-making under uncertainty.
Types of Probabilistic Graphical Models
There are two main types of probabilistic graphical models: Bayesian Networks and Markov Networks.
While each type serves similar purposes, they differ in the way they represent dependencies.
Bayesian Networks
Bayesian Networks are directed acyclic graphs (DAGs).
Each node in the graph represents a random variable, and the edges depict the conditional dependencies between these variables.
The “directed” aspect refers to the edges having a specific direction, which indicates the influence of one variable over another.
A key feature of Bayesian Networks is their ability to represent causal relationships.
They can model the direction of influence between factors, such as “smoking causes lung cancer,” rather than a simple correlation.
This makes them particularly suitable for causal inference, as they can capture how changes in one variable might affect others in the system.
Markov Networks
Markov Networks, also known as Markov Random Fields, use undirected graphs.
In these models, the absence of a directed edge implies that no conditional dependency information is specified.
Instead, Markov Networks are used to capture the joint distribution over a set of variables where the focus is more on the pattern of independence than causality.
Markov Networks are often used in contexts where the relationships between variables are symmetric.
They are also popular in computer vision and language processing, where spatial and contextual patterns play a significant role.
Applications of Probabilistic Graphical Models in Causal Inference
Causal inference is about understanding the effect of one variable on another.
It helps in answering questions like “What would happen if we change the value of a particular variable?” or “What is the likely cause of an observed event?”
Why Use PGMs for Causal Inference?
PGMs are adept at handling causal inference due to their structured representation of relationships.
By modeling how variables influence each other, PGMs can simulate the potential outcomes of interventions in a system.
This makes them incredibly useful for understanding causal mechanisms in various domains, such as healthcare, economics, and social sciences.
Healthcare
In healthcare, PGMs can be used to model the progression of diseases and the impact of treatment interventions.
For example, a Bayesian Network could help determine the probability of an adverse reaction in patients undergoing a particular medication regimen by taking into account various biological and lifestyle factors.
This capability allows for better treatment planning and personalized medicine approaches.
Economics
In the field of economics, PGMs aid analysts in understanding complex market dynamics.
By modeling economic indicators and their interdependencies, Bayesian Networks can predict the impact of policy changes or external shocks on markets.
These insights can then inform better decision-making to manage risk and opportunity in economic planning.
Social Sciences
For social scientists, PGMs offer a way to explore relationships between behavioral patterns and societal factors.
Through causal models, researchers can study the impact of educational programs or policy interventions on social behavior and outcomes.
This deepens the understanding of how various social factors contribute to observed trends and helps devise effective social strategies.
Challenges and Considerations
While PGMs are incredibly powerful, they are not without challenges.
Constructing an accurate graphical model requires a deep understanding of the domain and careful consideration of the relationships between variables.
Computational Complexity
As the number of variables increases, the complexity of the model computation also rises.
Ensuring that the model remains efficient and manageable is a significant challenge, especially in large datasets typical of modern applications.
Data Quality
Probabilistic models rely heavily on the quality of the input data.
Poor-quality data can lead to erroneous conclusions.
It is essential to ensure that data is clean, accurate, and representative of the underlying phenomena.
Model Selection
Choosing the correct type of PGM and structure requires careful consideration of the specifics of the problem at hand.
Experts often use domain knowledge to guide the selection process, but missteps can lead to poor model performance.
Conclusion
Probabilistic graphical models play a crucial role in understanding and inferring causal relationships across diverse fields.
By providing a structured method to represent uncertainties and dependencies, PGMs empower decision-makers to explore and predict complex interactions within systems.
Despite their challenges, with proper construction and high-quality data, PGMs can unlock valuable insights and advance our knowledge in an increasingly data-driven world.
資料ダウンロード
QCD調達購買管理クラウド「newji」は、調達購買部門で必要なQCD管理全てを備えた、現場特化型兼クラウド型の今世紀最高の購買管理システムとなります。
ユーザー登録
調達購買業務の効率化だけでなく、システムを導入することで、コスト削減や製品・資材のステータス可視化のほか、属人化していた購買情報の共有化による内部不正防止や統制にも役立ちます。
NEWJI DX
製造業に特化したデジタルトランスフォーメーション(DX)の実現を目指す請負開発型のコンサルティングサービスです。AI、iPaaS、および先端の技術を駆使して、製造プロセスの効率化、業務効率化、チームワーク強化、コスト削減、品質向上を実現します。このサービスは、製造業の課題を深く理解し、それに対する最適なデジタルソリューションを提供することで、企業が持続的な成長とイノベーションを達成できるようサポートします。
オンライン講座
製造業、主に購買・調達部門にお勤めの方々に向けた情報を配信しております。
新任の方やベテランの方、管理職を対象とした幅広いコンテンツをご用意しております。
お問い合わせ
コストダウンが利益に直結する術だと理解していても、なかなか前に進めることができない状況。そんな時は、newjiのコストダウン自動化機能で大きく利益貢献しよう!
(Β版非公開)