- お役立ち記事
- Process optimization using response surface methodology and its practical methods that should be addressed by the R&D department
Process optimization using response surface methodology and its practical methods that should be addressed by the R&D department
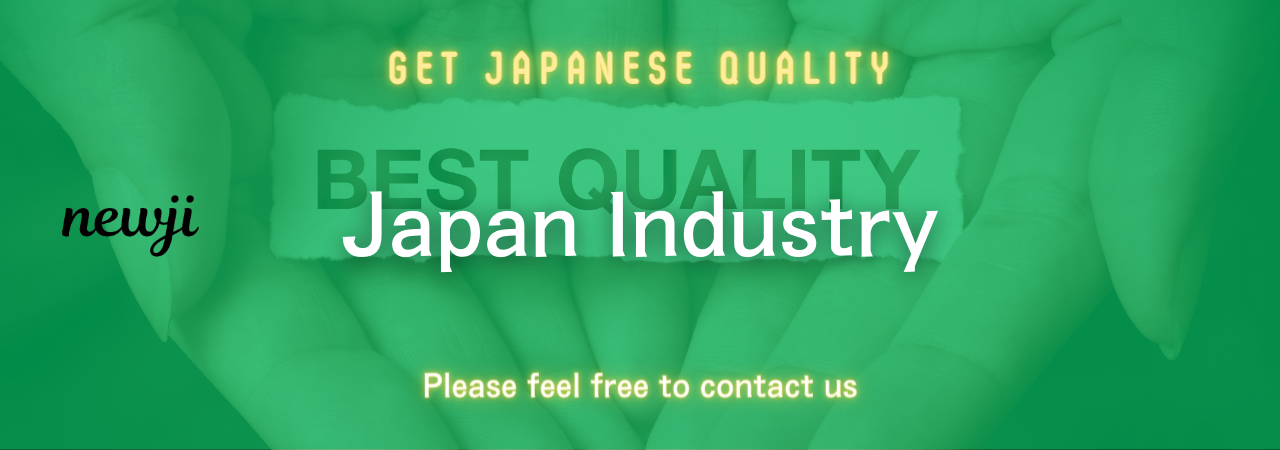
目次
Understanding Response Surface Methodology (RSM)
Response Surface Methodology (RSM) is a collection of mathematical and statistical techniques useful for modeling and analyzing problems where several variables influence a response of interest.
The primary objective is to optimize this response.
RSM is particularly useful in situations where a response is influenced by various variables, and the goal is to identify the optimal combination of these variables.
Industries ranging from manufacturing to pharmaceuticals often utilize RSM to enhance the efficiency and effectiveness of their processes.
By understanding how input variables interact with each other and affect the final output, organizations can streamline production processes and improve product quality.
Concepts of RSM
At the core of RSM lies the concept of the response surface, which is a graphical representation of a response to various factors.
This surface can help identify the optimal conditions for a desired response.
The mathematical models often used in RSM are quadratic polynomials that help describe the behavior of the response variable.
By fitting these models to experimental data, researchers can predict outcomes for different combinations of variables.
Central to RSM is the design of experiments (DOE), which helps ensure that the experiment is efficient, minimizes resource usage, and provides enough data for accurate analysis.
The Importance of Process Optimization
Process optimization is essential for R&D departments as it directly impacts operational efficiency, cost reduction, and product quality.
By refining manufacturing processes or chemical formulations, companies can enhance their competitiveness in the market.
Optimizing processes helps in reducing waste, improving the yield of products, and conserving resources.
This is crucial in today’s industry landscape, where sustainability and cost-efficiency are high priorities.
By fine-tuning the parameters affecting a process, organizations can ensure compliance with regulations, improve customer satisfaction, and boost profitability.
Practical Methods for Implementing RSM in R&D
Implementing RSM in an R&D department involves several practical steps.
Here are some methods that these departments should address:
Design of Experiments (DOE)
A well-structured DOE is vital for successful process optimization.
It involves planning experiments to collect data efficiently while ensuring that the results are statistically valid.
Factorial designs, such as full or fractional factorials, help in identifying the most significant factors and their interactions.
DOE is instrumental in setting the stage for RSM by defining the initial set of experiments needed to build response models.
Building and Testing Models
Once experiments are conducted, the next step is to develop mathematical models that describe the response surface.
These models, usually second-order polynomial equations, analyze and predict the behavior under different variable settings.
It is crucial to verify model assumptions and validate predictions through additional experiments or real-world data.
Validation checks the model’s accuracy and reliability in predicting responses.
Optimization Algorithms and Tools
Various software tools and algorithms are available to aid in the optimization of the response surface.
These tools help find the best conditions for the desired response by navigating the model’s surface effectively.
Software like Design-Expert, JMP, and Minitab are commonly used for modeling and optimization.
These tools provide user-friendly interfaces to design and analyze experiments, making implementation easier for R&D teams.
Challenges and Considerations
While RSM offers significant advantages, its implementation can pose challenges that must be considered carefully.
Complex Interactions and Noise
In real-world scenarios, processes often have complex interactions between variables.
Noise or experimental error can affect the accuracy of models, so robust experimental designs are necessary to mitigate these effects.
Resource and Time Constraints
Conducting a comprehensive set of experiments can be resource-intensive.
Balancing the need for detailed data collection with available time and budget is crucial for successful implementation.
Interdisciplinary Collaboration
Process optimization often requires collaboration across different disciplines (e.g., chemistry, engineering, statistics).
Ensuring effective communication and coordination among teams is critical to align objectives and share insights.
Conclusion
Response Surface Methodology is a powerful tool for optimizing processes and improving product quality in various industries.
By systematically exploring the relationships between variables, R&D departments can identify optimal conditions for desired responses.
Implementing RSM in practice involves careful planning, execution, and analysis.
Despite potential challenges, the benefits of process optimization are evident in terms of efficiency, cost reduction, and customer satisfaction.
Ultimately, by embracing RSM and process optimization, organizations can stay competitive and responsive to market demands, ensuring sustainable growth and success.
資料ダウンロード
QCD調達購買管理クラウド「newji」は、調達購買部門で必要なQCD管理全てを備えた、現場特化型兼クラウド型の今世紀最高の購買管理システムとなります。
ユーザー登録
調達購買業務の効率化だけでなく、システムを導入することで、コスト削減や製品・資材のステータス可視化のほか、属人化していた購買情報の共有化による内部不正防止や統制にも役立ちます。
NEWJI DX
製造業に特化したデジタルトランスフォーメーション(DX)の実現を目指す請負開発型のコンサルティングサービスです。AI、iPaaS、および先端の技術を駆使して、製造プロセスの効率化、業務効率化、チームワーク強化、コスト削減、品質向上を実現します。このサービスは、製造業の課題を深く理解し、それに対する最適なデジタルソリューションを提供することで、企業が持続的な成長とイノベーションを達成できるようサポートします。
オンライン講座
製造業、主に購買・調達部門にお勤めの方々に向けた情報を配信しております。
新任の方やベテランの方、管理職を対象とした幅広いコンテンツをご用意しております。
お問い合わせ
コストダウンが利益に直結する術だと理解していても、なかなか前に進めることができない状況。そんな時は、newjiのコストダウン自動化機能で大きく利益貢献しよう!
(Β版非公開)