- お役立ち記事
- “Productivity data analysis” from a management perspective—how to formulate improvement measures based on data
“Productivity data analysis” from a management perspective—how to formulate improvement measures based on data
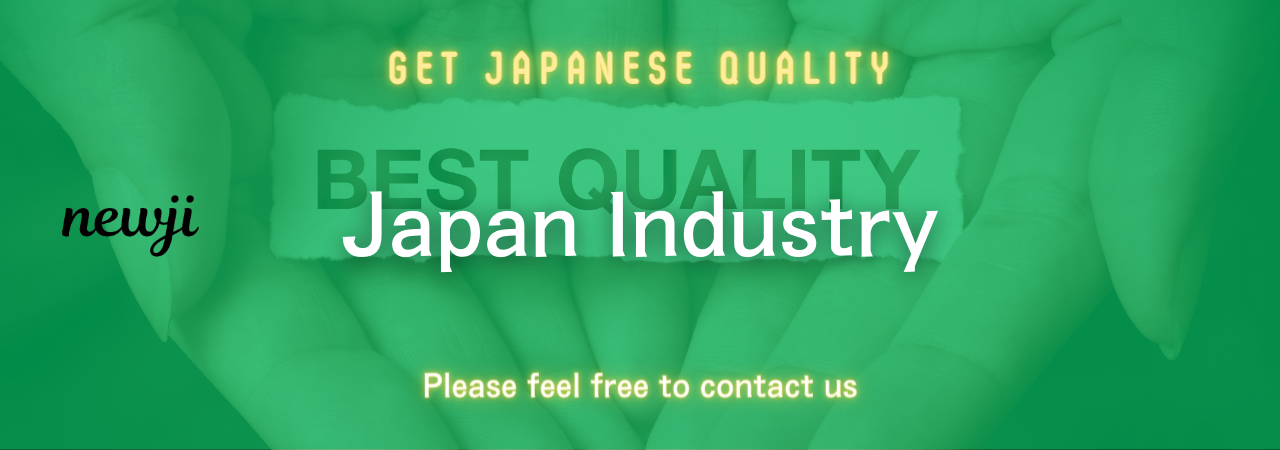
目次
Understanding Productivity Data Analysis
In today’s fast-paced business environment, productivity is more important than ever.
Companies constantly seek ways to improve their efficiency and effectiveness.
Productivity data analysis offers invaluable insights into how a company can enhance its operations.
By understanding these insights and learning how to apply them, management can make informed decisions that boost productivity levels.
Productivity data analysis involves examining the metrics and data related to an organization’s output.
This process helps management to identify patterns, bottlenecks, and opportunities for improvement.
A thorough analysis allows companies to pinpoint areas where their resources may be underutilized.
Thus, they can formulate strategies to address these issues.
The Importance of Data-Driven Decisions
For management, relying on gut instincts or traditional decision-making methods is not enough.
Data-driven decisions provide a solid foundation to support managerial actions.
By evaluating productivity data, managers can develop a clear understanding of current performance levels.
They can determine what is working and what needs to change.
Informed decisions based on data are generally more effective than those based on assumptions.
Organizations that prioritize data-driven decision-making often outpace those that do not.
This is because they can adapt quickly to changing market conditions and internal challenges.
Collecting and Analyzing Productivity Data
To start with productivity data analysis, the first step is to collect relevant data.
Data can come from different departments such as sales, manufacturing, customer service, etc.
Key performance indicators (KPIs) associated with these departments provide a basis for analysis.
Analyzing the collected data involves using statistical tools and software.
These tools help in recognizing trends, identifying outliers, and making forecasts.
Modern data analytics solutions allow management to visualize data through charts and graphs, facilitating easier interpretation.
Performance metrics like output per hour, error rates, and turnaround time are common productivity measures.
These metrics give insights into how resources are being utilized.
However, to get a complete picture, qualitative data, such as employee feedback, should also be considered.
Identifying Key Areas for Improvement
The analysis stage is about identifying strengths and weaknesses within the current processes.
For instance, a high error rate might point to the need for better training or equipment.
One effective method is to carry out a SWOT analysis (Strengths, Weaknesses, Opportunities, Threats).
This framework helps in understanding internal and external factors affecting productivity.
Managers can focus on converting weaknesses into strengths and threats into opportunities.
Recognizing patterns in the productivity data can lead to innovation.
For instance, identifying a slow production line can pave the way for automation.
Similarly, understanding customer service data might lead to improved customer interaction protocols.
Creating a Data-Informed Strategy
Once the areas for improvement are clear, management can create strategies to address them.
This may involve setting up new processes, investing in technology, or reallocating resources.
Setting Specific, Measurable, Achievable, Relevant, and Time-bound (SMART) goals can be beneficial.
For example, if data shows that manufacturing output needs improvement, a SMART goal could be to increase output by 15% within the next six months.
It is also crucial to involve all stakeholders in the formulation phase.
Employees at all levels should understand what the data indicates and how proposed changes will benefit them and the organization.
Involving them helps in gaining their buy-in, which is vital for successful implementation.
Implementing and Monitoring Improvement Measures
After strategy formulation, the next step is implementation.
This phase requires thorough planning and communication to ensure everyone is on the same page.
The implementation should be phased to minimize disruptions.
Once the improvement measures are in place, ongoing monitoring is necessary.
Metrics should be tracked to measure progress against the set goals.
Feedback loops should be established to allow for continuous refinement of strategies.
Regular data review sessions help in keeping the momentum on improvement initiatives.
Adjustments should be made based on real-time data to maintain or increase productivity levels.
The Role of Technology in Enhancing Productivity
Technology plays a crucial role in productivity data analysis.
With advanced analytics tools, businesses can automate data collection and processing.
Software solutions enable quicker insights into large datasets, thus speeding up decision-making.
Predictive analytics is a powerful technology that can foresee potential productivity issues before they occur.
This proactive approach helps in preempting problems and mitigating risks.
Furthermore, collaboration tools such as project management software streamline workflows.
They ensure that all team members are productive and aligned with organizational goals.
Challenges and Solutions in Productivity Data Analysis
Despite its benefits, productivity data analysis presents challenges such as data overload, interpretation difficulties, and privacy concerns.
Overcoming these challenges requires a strategic approach.
To address data overload, it is essential to focus on the data that matters.
Management should identify key metrics that directly impact productivity and prioritize them.
To improve interpretation, training staff on data analytics tools is vital.
As for privacy concerns, strict data governance policies should be in place to protect sensitive information while facilitating analysis.
In conclusion, effective productivity data analysis is pivotal for organizational success.
By collecting, analyzing, and applying data-driven insights, management can significantly enhance productivity.
Though challenges exist, with the right tools and strategies, organizations can harness the power of data to drive meaningful improvements.
資料ダウンロード
QCD調達購買管理クラウド「newji」は、調達購買部門で必要なQCD管理全てを備えた、現場特化型兼クラウド型の今世紀最高の購買管理システムとなります。
ユーザー登録
調達購買業務の効率化だけでなく、システムを導入することで、コスト削減や製品・資材のステータス可視化のほか、属人化していた購買情報の共有化による内部不正防止や統制にも役立ちます。
NEWJI DX
製造業に特化したデジタルトランスフォーメーション(DX)の実現を目指す請負開発型のコンサルティングサービスです。AI、iPaaS、および先端の技術を駆使して、製造プロセスの効率化、業務効率化、チームワーク強化、コスト削減、品質向上を実現します。このサービスは、製造業の課題を深く理解し、それに対する最適なデジタルソリューションを提供することで、企業が持続的な成長とイノベーションを達成できるようサポートします。
オンライン講座
製造業、主に購買・調達部門にお勤めの方々に向けた情報を配信しております。
新任の方やベテランの方、管理職を対象とした幅広いコンテンツをご用意しております。
お問い合わせ
コストダウンが利益に直結する術だと理解していても、なかなか前に進めることができない状況。そんな時は、newjiのコストダウン自動化機能で大きく利益貢献しよう!
(Β版非公開)