- お役立ち記事
- Quality and Safety Assurance for AI-Integrated Systems
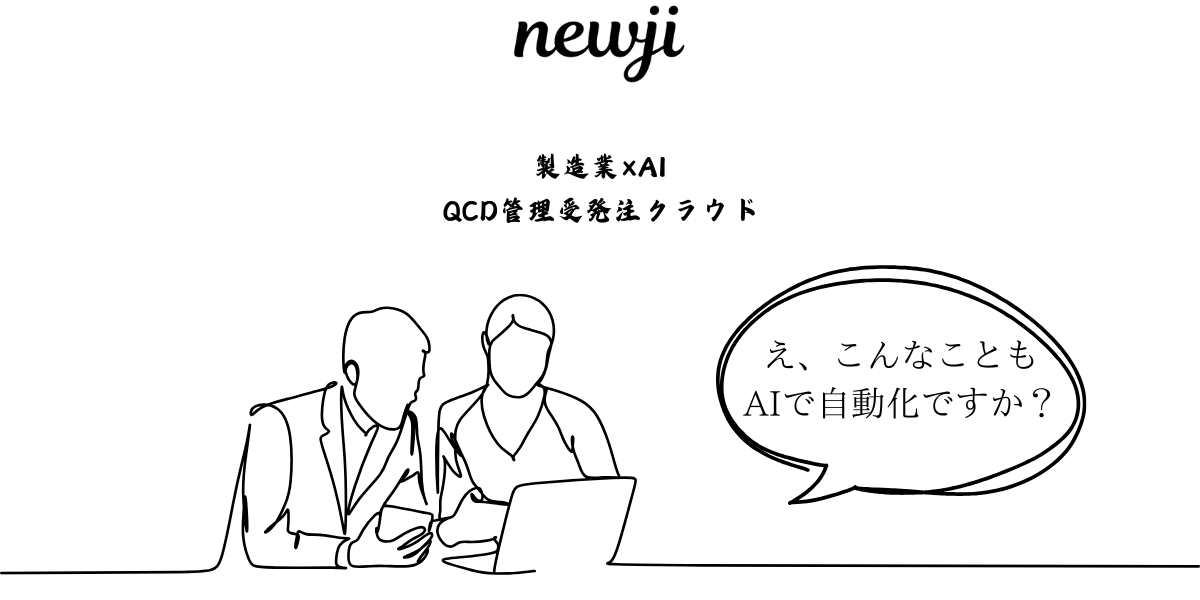
Quality and Safety Assurance for AI-Integrated Systems
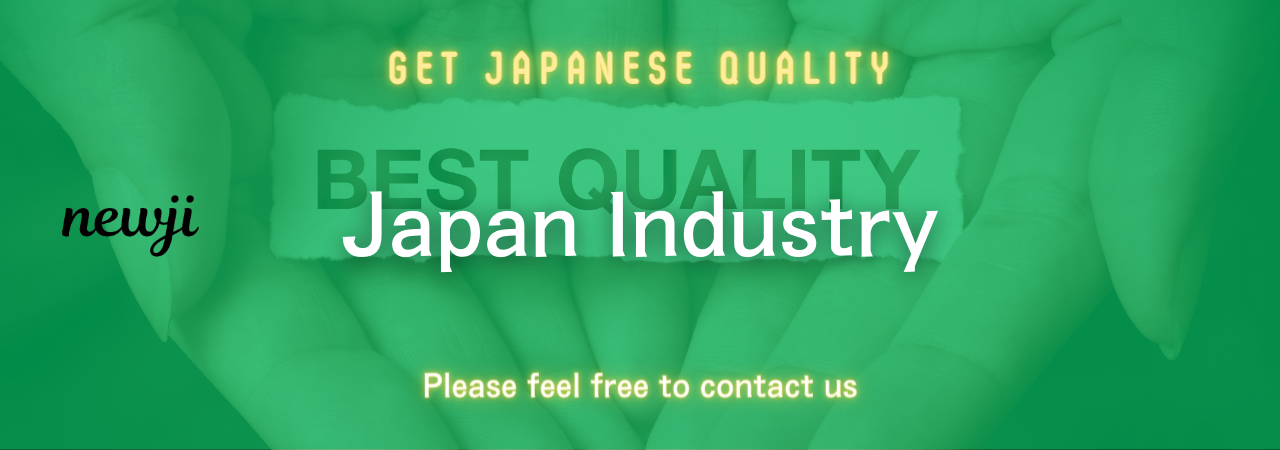
目次
Understanding AI-Integrated Systems
AI-integrated systems are becoming increasingly prevalent in our day-to-day lives, from smart home devices to autonomous vehicles and complex industrial applications.
These systems leverage artificial intelligence to enhance their functionality, responsiveness, and efficiency.
However, as their usage expands, ensuring their quality and safety becomes paramount.
AI technologies are inherently complex and can pose unique challenges when it comes to validation and reliability.
What are AI-Integrated Systems?
AI-integrated systems are designed to perform tasks by utilizing artificial intelligence algorithms.
Unlike traditional systems that follow fixed instructions, AI systems can learn, adapt, and make decisions based on data.
They can be used in a wide range of applications, including healthcare, transportation, finance, and more.
These systems can process and analyze vast amounts of data rapidly, providing insights and automating processes that were not possible before.
The Importance of Quality Assurance
Quality assurance in AI-integrated systems is critical for several reasons.
First, these systems often operate in environments where errors can lead to severe consequences, such as in autonomous driving or medical diagnosis.
Ensuring quality minimizes risks and maximizes performance, contributing to the safety and effectiveness of the system.
Moreover, a robust quality assurance process can foster trust among users and stakeholders, who rely on these technologies to deliver accurate and reliable results.
Challenges in AI Quality Assurance
AI systems are inherently dynamic due to their learning capabilities, which poses a significant challenge for traditional quality assurance methods.
Standard testing approaches may not account for the adaptive nature of AI, requiring new strategies to evaluate system performance accurately.
Moreover, the data-driven nature of AI systems means that their reliability heavily depends on the quality and breadth of the data they are trained on.
If the data is biased or incomplete, the system’s performance can be compromised.
Another challenge lies in understanding and interpreting AI decision-making processes.
Many AI systems, particularly those using neural networks, are often regarded as “black boxes,” making it difficult to explain how specific decisions are made.
This opaqueness can hinder efforts to audit, verify, and ensure the system meets quality and safety standards.
Ensuring Safety in AI-Integrated Systems
Safety is a top priority when integrating AI into systems, especially where there’s potential for human harm.
Achieving safety in AI systems involves a comprehensive approach that includes rigorous testing, continuous monitoring, and transparent evaluation processes.
Implementing Safety Protocols
Safety protocols for AI systems should be developed with an understanding of the specific environment and tasks the AI will undertake.
Developers must anticipate potential failure modes and implement fail-safes to mitigate risks.
This might involve having human oversight in critical operations or setting up automated alerts when the system operates outside acceptable parameters.
Regular system updates are crucial to maintain safety, as they allow developers to address newly discovered vulnerabilities and improve system robustness.
Moreover, it’s essential to establish guidelines for ethical AI use, ensuring that AI systems respect user autonomy and privacy.
Best Practices for Quality and Safety Assurance
To ensure high quality and safety standards, certain best practices should be followed in developing and maintaining AI-integrated systems.
Robust Testing and Validation
Testing AI systems under a variety of operating conditions is essential to understand their strengths and limitations.
It involves both static testing for system stability and dynamic testing that simulates real-world scenarios.
Validation processes should be transparent and reproducible, allowing for consistent evaluation of performance and decision logic.
Data Management and Governance
Quality data is the cornerstone of reliable AI systems.
To maintain data quality, organizations should implement strong data governance practices, which include data curation, validation, and ethical management.
It’s important to provide the system with diverse and representative datasets to avoid biased outcomes and inaccuracies.
Monitoring and Continuous Improvement
AI systems should be regularly monitored, and their performance metrics should be analyzed to identify areas for improvement.
Implementing a feedback loop where the system learns from its environment and prior experiences can significantly enhance its efficiency and effectiveness.
Continuous improvement methodologies ensure that systems evolve to meet changing requirements and enhance their safety over time.
Conclusion
The integration of AI into various systems presents a transformative potential but also comes with challenges regarding quality and safety.
By adopting comprehensive quality assurance strategies and safety protocols, organizations can mitigate potential risks and ensure that AI systems perform effectively and responsibly.
Ultimately, fostering an environment of trust and accountability in AI-integrated systems will pave the way for broader acceptance and utilization of these powerful technologies.
資料ダウンロード
QCD調達購買管理クラウド「newji」は、調達購買部門で必要なQCD管理全てを備えた、現場特化型兼クラウド型の今世紀最高の購買管理システムとなります。
ユーザー登録
調達購買業務の効率化だけでなく、システムを導入することで、コスト削減や製品・資材のステータス可視化のほか、属人化していた購買情報の共有化による内部不正防止や統制にも役立ちます。
NEWJI DX
製造業に特化したデジタルトランスフォーメーション(DX)の実現を目指す請負開発型のコンサルティングサービスです。AI、iPaaS、および先端の技術を駆使して、製造プロセスの効率化、業務効率化、チームワーク強化、コスト削減、品質向上を実現します。このサービスは、製造業の課題を深く理解し、それに対する最適なデジタルソリューションを提供することで、企業が持続的な成長とイノベーションを達成できるようサポートします。
オンライン講座
製造業、主に購買・調達部門にお勤めの方々に向けた情報を配信しております。
新任の方やベテランの方、管理職を対象とした幅広いコンテンツをご用意しております。
お問い合わせ
コストダウンが利益に直結する術だと理解していても、なかなか前に進めることができない状況。そんな時は、newjiのコストダウン自動化機能で大きく利益貢献しよう!
(Β版非公開)