- お役立ち記事
- Quality and safety assurance technology and verification methods for AI-equipped systems and their important points
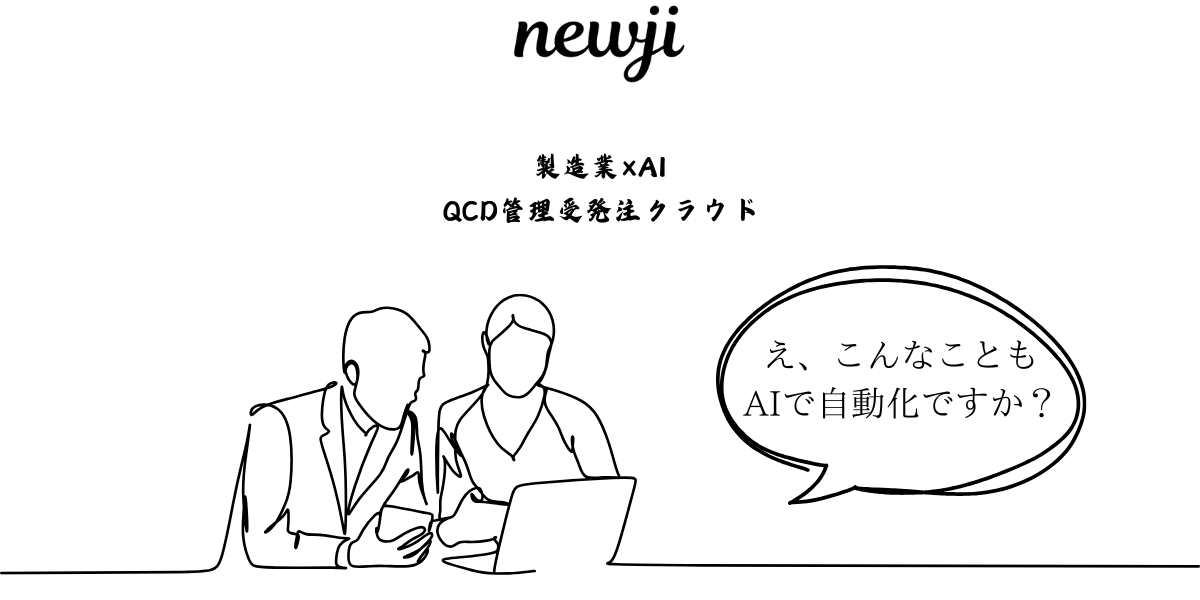
Quality and safety assurance technology and verification methods for AI-equipped systems and their important points
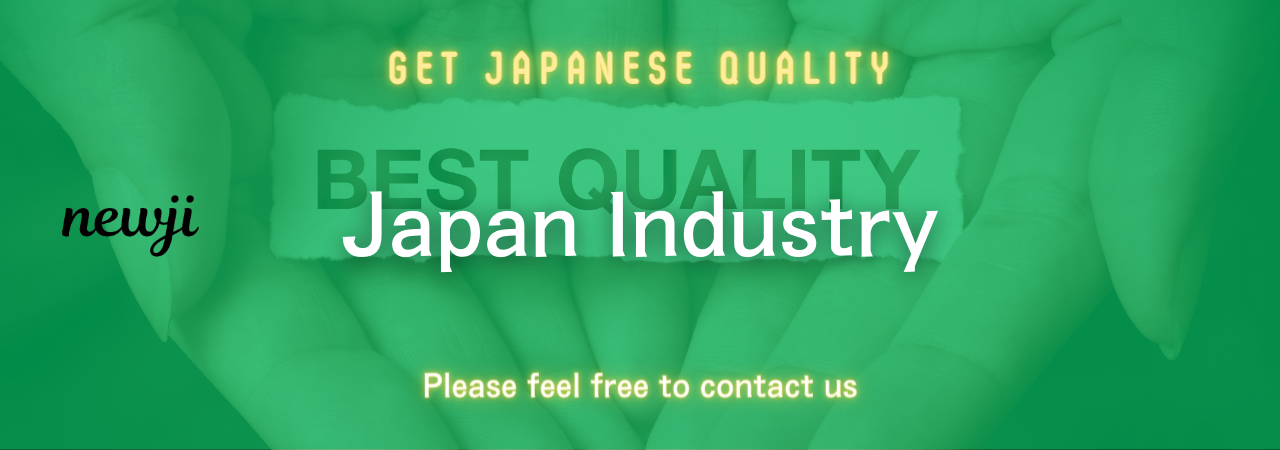
目次
Understanding AI-Equipped Systems
AI-equipped systems have become integral in today’s technological landscape, impacting various sectors such as healthcare, automotive, finance, and more.
These systems utilize artificial intelligence to process information, make decisions, and automate tasks, which can enhance efficiency, accuracy, and scalability.
However, as these systems become more embedded in critical operations, ensuring their quality and safety becomes a priority.
What is Quality Assurance in AI?
Quality assurance (QA) for AI systems involves practices and methodologies aimed at ensuring the system performs as intended without degradation or failure.
For AI to be reliable, it must not only deliver accurate results but also maintain stable performance under different conditions and datasets.
QA in AI spans several domains:
– **Data Quality:** Ensuring that the data used for training AI models is accurate, comprehensive, and bias-free.
– **Model Performance:** Verifying that the model is performing accurately and consistently.
– **System Integration:** Confirming that the AI system works seamlessly with other system components.
Safety Assurance for AI Systems
Safety assurance ensures that AI systems operate without causing harm, adhering to regulatory and safety standards.
For AI-equipped systems, safety assurance encompasses several elements:
– **Risk Assessment:** Identifying potential risks posed by AI operations and determining their impact.
– **Fail-Safe Mechanisms:** Implementing processes to manage and mitigate failures.
– **Compliance:** Ensuring that AI systems comply with existing legal and regulatory frameworks.
Verification Methods for AI Systems
Verification is a critical process in assuring the quality and safety of AI systems. It involves confirming that a system meets predefined specifications and behaves as expected. Here are some common verification methods for AI systems:
Testing and Validation
Testing involves executing the AI model on a dataset to assess its performance, accuracy, and reliability.
Validation ensures that the AI system’s outputs align with real-world expectations. Different testing approaches include:
– **Unit Testing:** Checking individual components of the AI system for accuracy.
– **Integration Testing:** Evaluating how different components function together.
– **System Testing:** Assessing the entire system’s operation in a real- or near-real-world scenario.
Formal Verification
Formal verification uses mathematical techniques to prove or disprove the correctness of algorithms underpinning AI systems.
This method is particularly useful for safety-critical applications like autonomous vehicles or medical devices, where precision and certainty are paramount.
Continuous Monitoring and Feedback
AI systems need constant monitoring to ensure they continue to perform accurately and safely over time.
This method involves:
– **Real-Time Monitoring:** Tracking system outputs continuously to detect anomalies.
– **User Feedback:** Incorporating end-user feedback to improve system reliability and performance.
– **Regular Updates:** Updating models and algorithms to adapt to new data and scenarios.
Important Points in Ensuring Quality and Safety
To ensure quality and safety in AI-equipped systems, several important points must be considered:
Understand the Domain
Understanding the domain where AI is applied is fundamental.
It informs data selection, model design, and performance expectations.
Knowledge of the domain helps anticipate system behavior in varied scenarios.
Data Management
High-quality AI systems begin with high-quality data.
Data should be carefully managed to eliminate biases and inaccuracies.
This involves establishing transparent processes for data collection, annotation, and pre-processing.
Model Transparency and Interpretability
AI models should be transparent and interpretable to ensure stakeholders understand how decisions are made.
Understanding model operations helps diagnose and correct errors and assure users of the system’s reliability.
Collaboration and Multi-Disciplinary Teams
Building AI systems often requires collaboration across various disciplines.
Bringing together experts from different fields ensures a holistic approach to system design, development, and verification.
Compliance and Ethics
AI systems must comply with legal and ethical standards to maintain public trust.
Adhering to regulations and considering ethical implications are crucial for the acceptance and success of AI-equipped systems.
Continuous Improvement
AI systems require ongoing analysis and improvement.
Implementing a framework for regular system evaluation and updates ensures the system remains relevant and reliable over time.
Conclusion
AI-equipped systems hold tremendous potential but also come with significant responsibility.
Ensuring their quality and safety requires a thorough understanding of the technology, rigorous verification methods, and a commitment to continuous improvement.
By focusing on critical points such as domain knowledge, data management, model transparency, and ethical compliance, developers can build AI systems that not only perform efficiently but also earn users’ trust and confidence in their operation.
資料ダウンロード
QCD調達購買管理クラウド「newji」は、調達購買部門で必要なQCD管理全てを備えた、現場特化型兼クラウド型の今世紀最高の購買管理システムとなります。
ユーザー登録
調達購買業務の効率化だけでなく、システムを導入することで、コスト削減や製品・資材のステータス可視化のほか、属人化していた購買情報の共有化による内部不正防止や統制にも役立ちます。
NEWJI DX
製造業に特化したデジタルトランスフォーメーション(DX)の実現を目指す請負開発型のコンサルティングサービスです。AI、iPaaS、および先端の技術を駆使して、製造プロセスの効率化、業務効率化、チームワーク強化、コスト削減、品質向上を実現します。このサービスは、製造業の課題を深く理解し、それに対する最適なデジタルソリューションを提供することで、企業が持続的な成長とイノベーションを達成できるようサポートします。
オンライン講座
製造業、主に購買・調達部門にお勤めの方々に向けた情報を配信しております。
新任の方やベテランの方、管理職を対象とした幅広いコンテンツをご用意しております。
お問い合わせ
コストダウンが利益に直結する術だと理解していても、なかなか前に進めることができない状況。そんな時は、newjiのコストダウン自動化機能で大きく利益貢献しよう!
(Β版非公開)