- お役立ち記事
- Quality and safety assurance technology for AI-equipped systems and its important points
月間77,185名の
製造業ご担当者様が閲覧しています*
*2025年2月28日現在のGoogle Analyticsのデータより
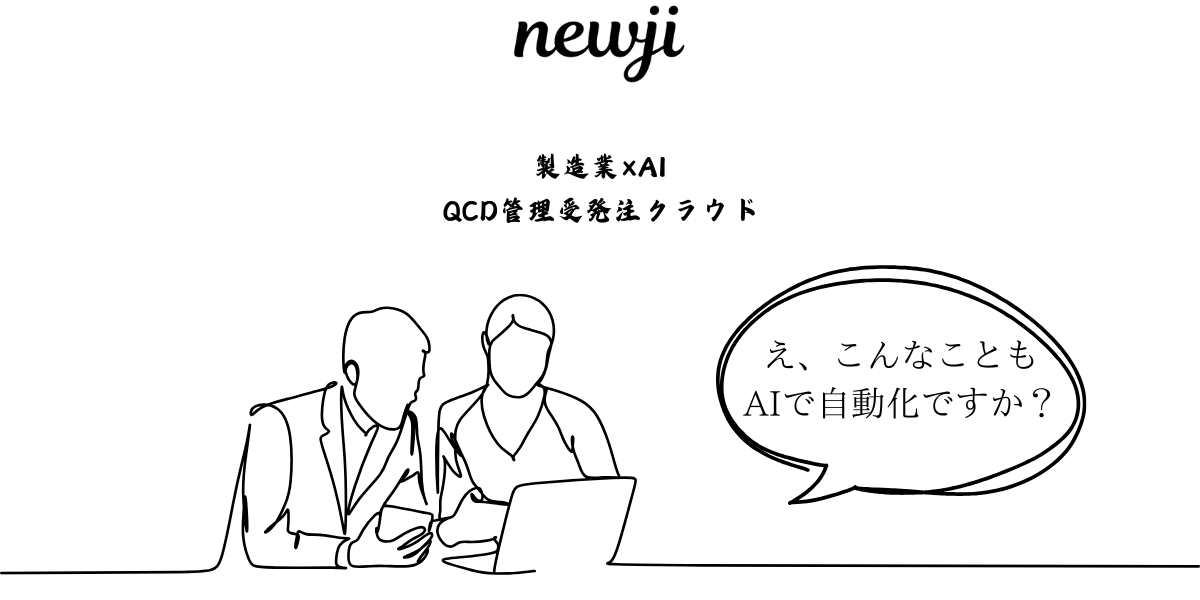
Quality and safety assurance technology for AI-equipped systems and its important points
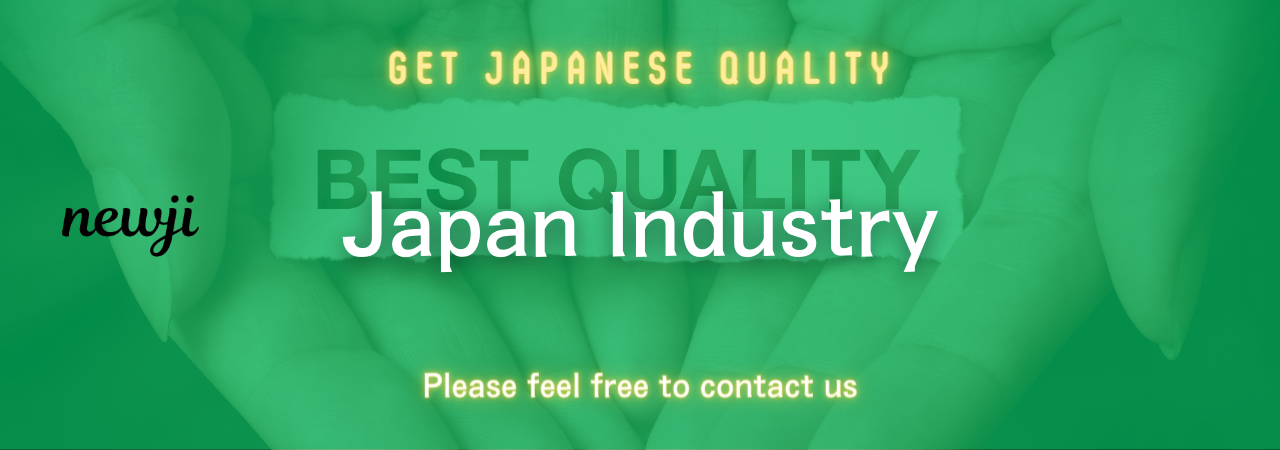
目次
Introduction to AI Quality and Safety Assurance
Artificial Intelligence (AI) has made significant strides in a short period, becoming an integral part of various systems we rely on daily.
From autonomous vehicles to healthcare diagnostics and smart personal assistants, AI equips systems with capabilities that were previously thought impossible.
However, as AI systems become more widespread, ensuring their quality and safety has become paramount.
Quality and safety assurance for AI-equipped systems is crucial not only for user satisfaction but also for avoiding potential risks and harm.
In this article, we’ll delve into the essential components of quality and safety assurance for AI-equipped systems and highlight important considerations.
Understanding AI System Quality
The quality of an AI system can be determined by its performance, reliability, and robustness.
Performance encompasses various factors, including accuracy, speed, and efficiency.
A high-quality AI system should consistently perform tasks to meet or exceed predefined benchmarks.
Reliability refers to the AI system’s ability to maintain consistent performance over time under different conditions.
Robustness measures the system’s ability to handle unexpected inputs or scenarios without significant performance deterioration.
The importance of ensuring AI quality cannot be overstated.
Poor quality can lead to inaccurate predictions, system failures, and diminished trust.
Therefore, rigorous testing and validation processes are necessary to assess and uphold the quality of AI systems.
Testing and Validation Processes
1. **Data Quality**: The performance of AI systems is greatly dependent on the quality of data they are trained on.
Ensure that the data is accurate, diverse, and representative of real-world scenarios.
2. **Model Evaluation**: Utilize various metrics to evaluate the AI model’s performance, such as precision, recall, F1 score, and more.
Proper evaluation helps in identifying areas of improvement.
3. **Simulations and Real-world Testing**: Conduct simulations and real-world tests to assess the AI system’s performance under different conditions.
This helps in verifying that the system can handle various scenarios reliably.
Ensuring Safety in AI Systems
Safety assurance in AI systems involves minimizing risks and preventing harm that could result from system failures or errors.
AI systems, especially those in critical sectors like healthcare and transportation, must adhere to safety standards to protect users and the environment.
Key Safety Considerations
1. **Transparency and Explainability**: AI systems should be transparent, allowing users to understand how decisions are made.
Explainable AI helps in diagnosing system behavior and identifying any safety issues.
2. **Security Measures**: Implement robust security protocols to protect AI systems from cyber threats.
Unauthorized access or tampering can compromise safety.
3. **Fail-safe Mechanisms**: Design AI systems with fail-safe mechanisms to ensure minimal harm in case of malfunctions.
This involves creating backup procedures and manual overrides when necessary.
4. **Ethical Considerations**: Address ethical concerns by ensuring the AI system does not exhibit biased behavior or make unethical decisions.
Regular audits can help in maintaining ethical standards.
Regulations and Standards
Various organizations and governing bodies are working towards developing regulations and standards to ensure the quality and safety of AI systems.
Compliance with these regulations is not only a legal obligation but also a best practice for developing trustworthy AI solutions.
Some key standards include:
1. **ISO/IEC JTC 1/SC 42**: This standard focuses on the development of standards that pertain to AI, promoting consistency, and interoperability across AI systems.
2. **IEEE P7000**: The IEEE standards address ethical concerns, ensuring AI systems operate in a manner beneficial to humanity and the environment.
3. **GDPR Compliance**: For AI systems handling personal data, compliance with the General Data Protection Regulation (GDPR) is essential to safeguard user privacy.
Challenges in Assurance Processes
Assuring quality and safety in AI systems is challenging due to the complexity and dynamic nature of AI technologies.
Some of these challenges include:
1. **Evolving Algorithms**: AI algorithms continuously adapt, making it difficult to maintain consistent quality evaluations over time.
2. **Interpretability**: Many AI models, particularly deep learning models, act as “black boxes” with limited transparency, complicating the analysis of their behavior.
3. **Data Privacy**: Balancing quality assurance with data privacy regulations presents a challenge for developers, requiring careful handling of data throughout the testing process.
Conclusion
Quality and safety assurance for AI-equipped systems is a critical aspect of their development and deployment.
As AI technologies continue to evolve, establishing robust assurance processes is necessary to minimize risks and ensure dependable performance.
By understanding and implementing proper testing, validation, safety measures, and adhering to regulations, stakeholders can foster trust in AI systems.
Ultimately, the goal is to harness the power of AI while safeguarding the well-being of users and society at large.
資料ダウンロード
QCD管理受発注クラウド「newji」は、受発注部門で必要なQCD管理全てを備えた、現場特化型兼クラウド型の今世紀最高の受発注管理システムとなります。
ユーザー登録
受発注業務の効率化だけでなく、システムを導入することで、コスト削減や製品・資材のステータス可視化のほか、属人化していた受発注情報の共有化による内部不正防止や統制にも役立ちます。
NEWJI DX
製造業に特化したデジタルトランスフォーメーション(DX)の実現を目指す請負開発型のコンサルティングサービスです。AI、iPaaS、および先端の技術を駆使して、製造プロセスの効率化、業務効率化、チームワーク強化、コスト削減、品質向上を実現します。このサービスは、製造業の課題を深く理解し、それに対する最適なデジタルソリューションを提供することで、企業が持続的な成長とイノベーションを達成できるようサポートします。
製造業ニュース解説
製造業、主に購買・調達部門にお勤めの方々に向けた情報を配信しております。
新任の方やベテランの方、管理職を対象とした幅広いコンテンツをご用意しております。
お問い合わせ
コストダウンが利益に直結する術だと理解していても、なかなか前に進めることができない状況。そんな時は、newjiのコストダウン自動化機能で大きく利益貢献しよう!
(β版非公開)