- お役立ち記事
- Quality deterioration sign detection technology and inspection data utilization method
月間77,185名の
製造業ご担当者様が閲覧しています*
*2025年2月28日現在のGoogle Analyticsのデータより
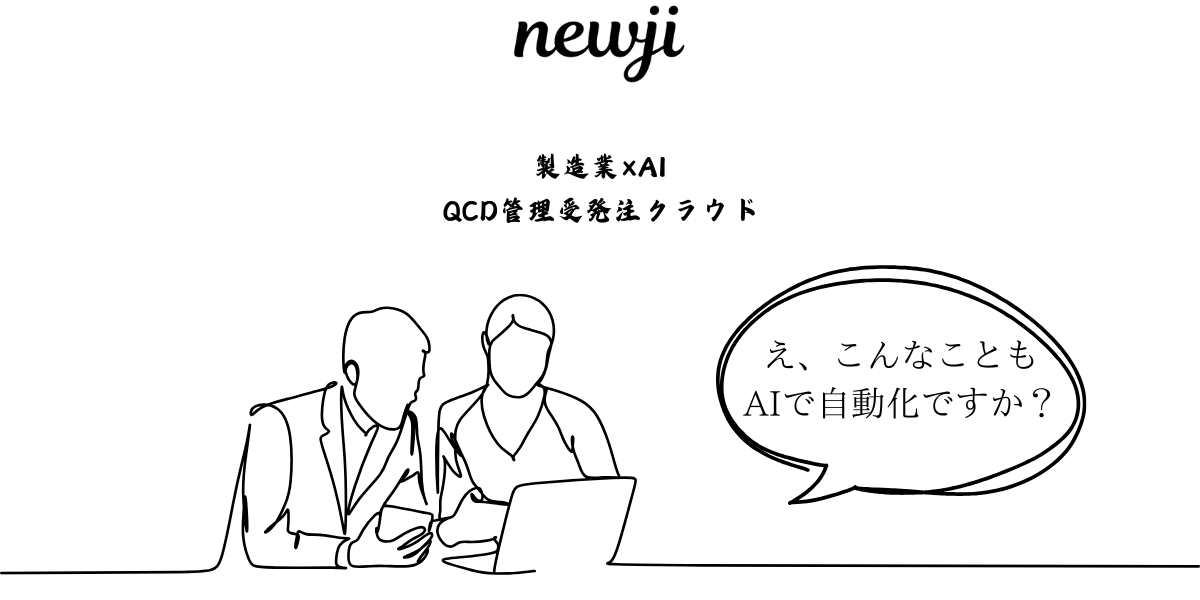
Quality deterioration sign detection technology and inspection data utilization method
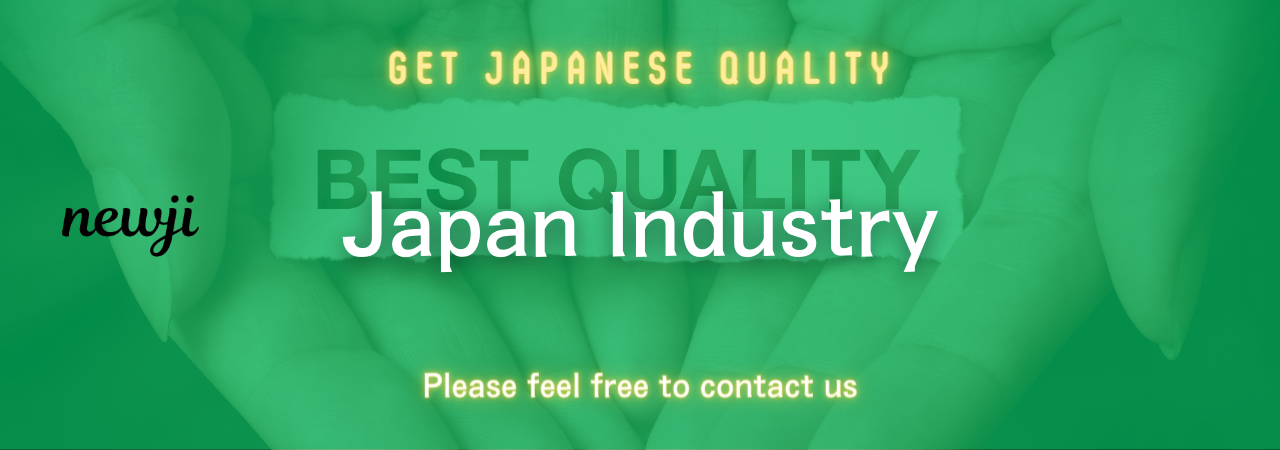
目次
Understanding Quality Deterioration Sign Detection Technology
Quality deterioration sign detection technology is an innovative approach used to identify potential defects or issues in products before they escalate into significant problems.
This technology leverages advanced tools and smart systems to monitor and analyze various parameters that signal a decline in product quality.
These parameters can range from vibration levels in machinery to temperature changes in storage environments.
As industries strive to deliver impeccable products, the implementation of such technology has become crucial.
Not only does it help in maintaining the quality standards, but it also plays a pivotal role in enhancing the overall efficiency of production processes.
How Quality Deterioration Sign Detection Works
At the core of quality deterioration sign detection technology is the ability to predict when and where a product might exhibit signs of wear or malfunction.
This is typically achieved through the integration of sensors and IoT (Internet of Things) devices within the production line.
These sensors collect real-time data which is then analyzed using sophisticated algorithms and machine learning models.
For example, in a manufacturing plant, sensors might detect abnormal vibrations in a piece of machinery.
This data is processed to determine if the vibration patterns could lead to machine failure if left unchecked.
By identifying such signs early, manufacturers can conduct maintenance or repairs as needed, thus avoiding costly downtimes.
The Benefits of Early Detection
One of the most significant advantages of using quality deterioration sign detection technology is the ability to prevent defects before they occur.
This proactive approach ensures that products meet all quality specifications, resulting in greater customer satisfaction and loyalty.
Additionally, early detection helps in minimizing waste and reducing operational costs.
When issues are identified and addressed promptly, it eliminates the need for extensive repairs or complete overhauls, which can be expensive.
Moreover, early identification supports better resource management.
By understanding when and why certain components might fail, businesses can optimize their supply chain operations, ensuring that replacements or upgrades are made efficiently.
Utilizing Inspection Data
Utilization of inspection data refers to the strategic use of data gathered during the inspection processes to make informed decisions and improvements.
Inspection data consists of valuable information about the product’s condition, performance history, and any anomalies that might have been detected.
To fully harness the power of this data, companies are adopting advanced analytics and AI-driven tools.
These tools can process large volumes of data quickly and identify patterns that might not be obvious otherwise.
Implementing Data-Driven Decisions
Once the inspection data is analyzed, the insights gained can lead to the refinement of production techniques and quality assurance processes.
For instance, if the data reveals a consistent defect in a particular batch, it may prompt changes in the manufacturing process to rectify the issue.
Moreover, data-driven decisions can help in personalizing maintenance schedules.
By predicting when a machine might need servicing based on its past performance data, companies can schedule maintenance activities at optimal times, enhancing production uptime.
Furthermore, the utilization of inspection data plays an integral role in facilitating a feedback loop.
This means the data gathered from quality inspections can be fed back into the design and development process, guiding the creation of more robust and reliable products.
The Role of Machine Learning and AI
Machine learning (ML) and artificial intelligence (AI) are central to the advancement of quality deterioration sign detection technology and the sophisticated use of inspection data.
ML algorithms can learn from historic and real-time data to make predictions about future quality issues.
For example, these algorithms can be trained on datasets from previous inspections to identify correlations between specific variables and product failures.
Similarly, AI systems can automate the decision-making process by providing actionable insights without the need for extensive human intervention.
They can trigger automatic alerts and corrective measures when certain thresholds are breached, thus ensuring swift action.
Enhancing Quality Control Protocols
Utilizing AI and ML, companies can enhance their quality control protocols, making them more predictive than reactive.
Instead of relying solely on end-product inspections, organizations can shift towards a model that emphasizes continuous monitoring and adjustment.
AI-driven insights contribute to the creation of smarter manufacturing environments where systems evolve with emerging patterns and past errors, further refining the quality assurance process.
Challenges and Future Directions
Despite the many advantages, implementing quality deterioration sign detection technology does come with challenges.
One significant hurdle is the integration of these advanced systems into existing workflows without disrupting operations.
Moreover, ensuring data accuracy and security are paramount, as faulty data can lead to incorrect predictions.
However, the future of this technology is promising, with continuous improvements in sensor technologies and data analytics tools.
As these technologies evolve, they will become more accessible and cost-effective, making them a standard part of quality control and assurance across various industries.
Innovations such as edge computing and cloud analytics are set to further enhance the speed and efficiency with which quality data is processed.
In conclusion, quality deterioration sign detection technology and the strategic use of inspection data represent a new frontier in maintaining product excellence.
By integrating these technologies into everyday operations, businesses can remain competitive, delivering superior products while optimizing their resources and processes.
資料ダウンロード
QCD管理受発注クラウド「newji」は、受発注部門で必要なQCD管理全てを備えた、現場特化型兼クラウド型の今世紀最高の受発注管理システムとなります。
ユーザー登録
受発注業務の効率化だけでなく、システムを導入することで、コスト削減や製品・資材のステータス可視化のほか、属人化していた受発注情報の共有化による内部不正防止や統制にも役立ちます。
NEWJI DX
製造業に特化したデジタルトランスフォーメーション(DX)の実現を目指す請負開発型のコンサルティングサービスです。AI、iPaaS、および先端の技術を駆使して、製造プロセスの効率化、業務効率化、チームワーク強化、コスト削減、品質向上を実現します。このサービスは、製造業の課題を深く理解し、それに対する最適なデジタルソリューションを提供することで、企業が持続的な成長とイノベーションを達成できるようサポートします。
製造業ニュース解説
製造業、主に購買・調達部門にお勤めの方々に向けた情報を配信しております。
新任の方やベテランの方、管理職を対象とした幅広いコンテンツをご用意しております。
お問い合わせ
コストダウンが利益に直結する術だと理解していても、なかなか前に進めることができない状況。そんな時は、newjiのコストダウン自動化機能で大きく利益貢献しよう!
(β版非公開)