- お役立ち記事
- Randomized block method and partitioning method, orthogonal array experimental design
この記事は、当社の提供するお役立ち記事の一部です。詳しくは公式サイトをご覧ください。
Randomized block method and partitioning method, orthogonal array experimental design
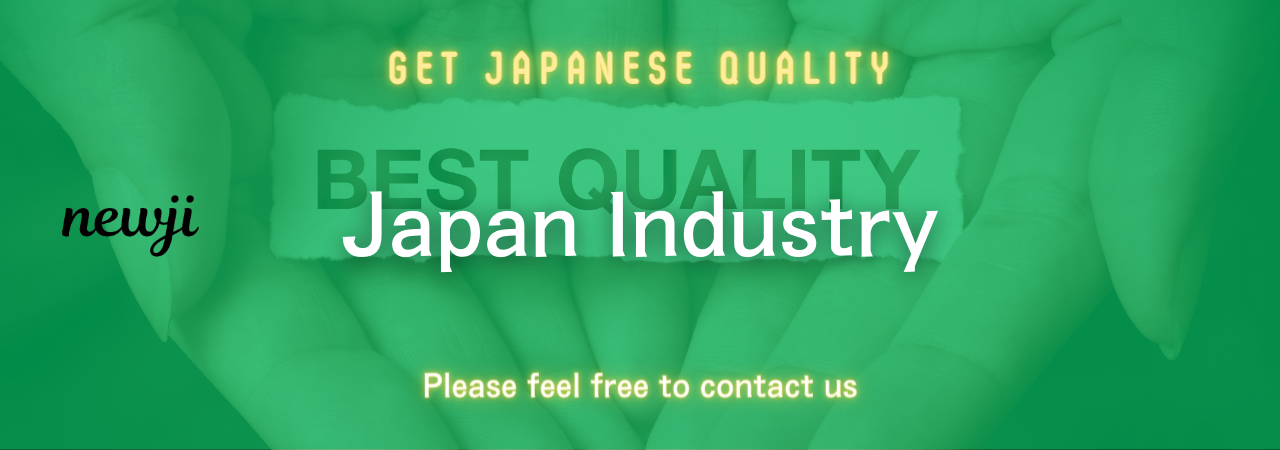
Understanding how to efficiently conduct experiments and analyze data is vital in various fields, from manufacturing to agricultural studies.
Two powerful tools used in experimental design are the Randomized Block Method and the Partitioning Method, together with Orthogonal Array Experimental Design.
These methodologies help researchers to minimize error, manage variability, and draw precise conclusions.
Let’s dive into each of these methods to better understand how they work and when to use them.
目次
What is the Randomized Block Method?
The Randomized Block Method is a statistical technique used in the design of experiments to account for variability among experimental units.
Generally, the idea is to group similar experimental units into blocks and then randomly assign different treatments to units within these blocks.
The main advantage of using the Randomized Block Method is that it effectively reduces the impact of confounding variables that might otherwise skew the results.
By controlling for these confounding variables, the variations that arise from within each block are attributed to the treatment rather than other external factors.
How Does the Randomized Block Method Work?
The process involves several steps:
1. **Identify Blocks:** Determine the factors in your study that are considered to be sources of variation.
These become your blocks.
2. **Assign Treatments Randomly:** Within each block, randomly assign the treatments to the experimental units.
3. **Conduct the Experiment:** Implement the study according to the plan, ensuring that the experimental conditions are consistent across all blocks.
4. **Analyze Results:** Compare the results between the treatment groups within each block to understand the effect of the treatment.
The Randomized Block Method is particularly useful in experiments where you expect certain variables to have a significant impact on the experimental outcome.
The Partitioning Method in Experimental Design
The Partitioning Method is another effective approach in the field of experimental design.
It involves dividing the experimental data into sections, or partitions, allowing for detailed analysis.
Why Use the Partitioning Method?
The Partitioning Method allows researchers to separate and analyze complex data scenarios.
By breaking the data into smaller, manageable partitions, it simplifies the process of detecting patterns and interactions within the data that might not be visible at a higher, aggregate level.
This technique is especially handy in situations where data are inherently heterogeneous.
For instance, in clinical trials, different patient groups may respond differently to treatments based on age, gender, or genetic factors.
Steps in the Partitioning Method
The basic steps usually include:
1. **Segment the Data:** Identify distinct characteristics or factors in your data that are worth isolating.
These become your partitions.
2. **Analyze Partitions Independently:** Conduct separate analyses for each partition.
This allows for comparisons and the identification of trends specific to each subset.
3. **Integrate Insights:** Combine findings from individual partitions to make broader inferences about the entire dataset.
Understanding Orthogonal Array Experimental Design
Orthogonal Array Experimental Design (OAED) is a systematic way to study multiple variables simultaneously.
This technique is particularly useful for optimizing processes and improving quality.
What are Orthogonal Arrays?
Orthogonal Arrays are structured matrices used in experimental designs to test combinations of factors and their levels in a balanced way.
They allow researchers to understand relationships between variables efficiently and effectively, using fewer experiments than would be required with other methods.
Advantages of Orthogonal Array Experimental Design
1. **Reduced Experimentation:** By carefully structuring the experiment, you need fewer trials, saving both time and resources.
2. **Balanced Testing:** Provides a balanced way to investigate the effects of multiple factors.
3. **Ease in Interpretation:** Simplifies the analysis of interaction effects between multiple factors.
Implementing an Orthogonal Array Design
To apply OAED, follow these steps:
1. **Select Factors and Levels:** Choose the factors you want to study and decide how many levels (variations) each factor will have.
2. **Choose an Appropriate Array:** Based on the number of factors and levels, select an orthogonal array that suits your study.
3. **Assign Factor Levels to the Array:** Map the chosen factors and levels onto the array to plan your experiments.
4. **Conduct the Experiments:** Execute the experiments as per the design plan, ensuring accuracy and consistency.
5. **Analyze the Results:** Use statistical tools to analyze the data, identifying the influence of each factor on the response variable.
When to Use These Methods?
Each of these experimental design methods has its place depending on the context of the study:
– **Randomized Block Method** is ideal when you have identified sources of variability you want to control for.
– **Partitioning Method** is suited for analyzing complex datasets with heterogeneous characteristics.
– **Orthogonal Array Experimental Design** is perfect when multiple factors are at play, and you need a cost-effective way to study them.
Understanding when and how to use these methods can significantly enhance the efficiency and effectiveness of experimental research.
By applying the appropriate method, you can ensure that your experiments are well-designed, the data collected are accurate, and the results are reliable.
This knowledge is fundamental in advancing research, solving real-world problems, and making informed decisions based on empirical evidence.
資料ダウンロード
QCD調達購買管理クラウド「newji」は、調達購買部門で必要なQCD管理全てを備えた、現場特化型兼クラウド型の今世紀最高の購買管理システムとなります。
ユーザー登録
調達購買業務の効率化だけでなく、システムを導入することで、コスト削減や製品・資材のステータス可視化のほか、属人化していた購買情報の共有化による内部不正防止や統制にも役立ちます。
NEWJI DX
製造業に特化したデジタルトランスフォーメーション(DX)の実現を目指す請負開発型のコンサルティングサービスです。AI、iPaaS、および先端の技術を駆使して、製造プロセスの効率化、業務効率化、チームワーク強化、コスト削減、品質向上を実現します。このサービスは、製造業の課題を深く理解し、それに対する最適なデジタルソリューションを提供することで、企業が持続的な成長とイノベーションを達成できるようサポートします。
オンライン講座
製造業、主に購買・調達部門にお勤めの方々に向けた情報を配信しております。
新任の方やベテランの方、管理職を対象とした幅広いコンテンツをご用意しております。
お問い合わせ
コストダウンが利益に直結する術だと理解していても、なかなか前に進めることができない状況。そんな時は、newjiのコストダウン自動化機能で大きく利益貢献しよう!
(Β版非公開)