- お役立ち記事
- Relationship between Bayesian statistics and MCMC
Relationship between Bayesian statistics and MCMC
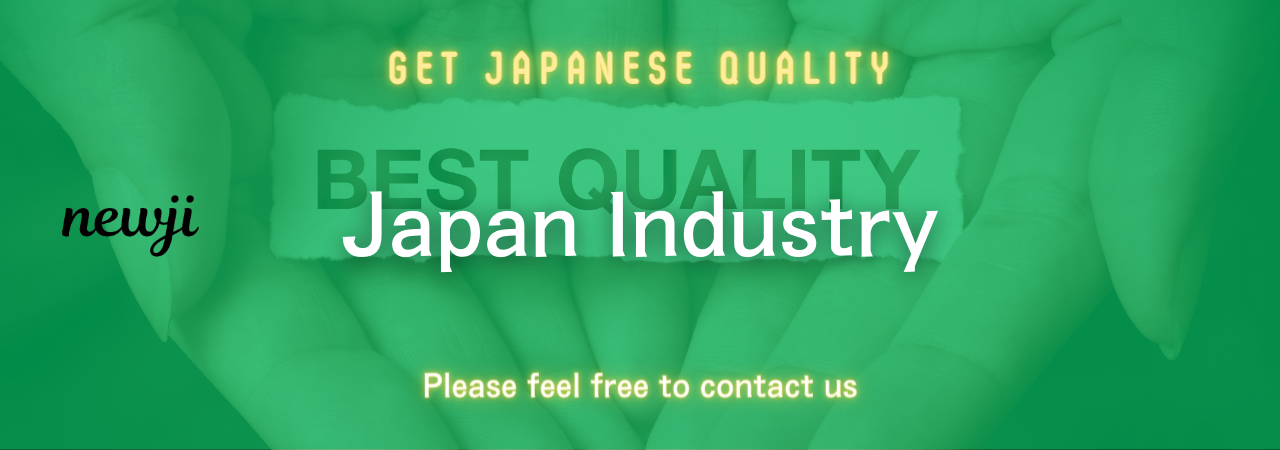
目次
Understanding Bayesian Statistics
Bayesian statistics is an approach to statistical inference that differs from the classical or frequentist approach.
At its core, Bayesian statistics is about updating probabilities as more evidence or information becomes available.
This method uses what is known as Bayes’ Theorem, which serves as the mathematical foundation.
Bayesian statistics takes into account both prior beliefs and new data.
The prior belief is quantified as a probability distribution before new evidence is considered.
When new data is introduced, this prior is updated to form a posterior distribution, which represents the updated belief about the model parameters or hypotheses.
For example, consider a simple scenario where we want to determine the probability of rain tomorrow.
Initially, you might believe there’s a 30% chance based on past weather patterns (prior probability).
However, if new weather data indicates a low-pressure system moving in, you might adjust that probability to a higher number (posterior probability).
Key Components of Bayesian Statistics
In Bayesian statistics, three essential components work together:
1. **Prior Distribution**: This represents your initial beliefs about a parameter before observing any data.
It could be based on previous studies, expert opinion, or even assumed to be uniform if no prior information is available.
2. **Likelihood**: This is the probability of observing the data given a specific hypothesis or parameter value.
It is the same as in frequentist statistics and usually derived from a statistical model.
3. **Posterior Distribution**: This is the updated belief after considering the evidence (data).
It is calculated using Bayes’ Theorem, combining the prior and the likelihood of the observed data.
Bayes’ Theorem can be mathematically represented as:
\[ \text{Posterior} \propto \text{Likelihood} \times \text{Prior} \]
Here, “proportional to” (\(\propto\)) indicates that the posterior distribution is scaled by the likelihood and prior.
What is MCMC?
Markov Chain Monte Carlo (MCMC) is a class of algorithms used to sample from probability distributions based on constructing a Markov chain.
This is particularly useful when dealing with complex or high-dimensional probability distributions where direct sampling is not feasible.
MCMC methods allow us to approximate the posterior distribution in Bayesian statistics.
They are essential tools when dealing with Bayesian inference, as calculating the posterior distribution analytically is often impossible in real-world problems.
Key Elements of MCMC
1. **Markov Chains**: A sequence of random samples where each sample depends only on the previous one.
The ‘memoryless’ property ensures that the chain converges to the target distribution over time.
2. **Monte Carlo Integration**: An approach that uses repeated random sampling to compute numerical estimates.
In the context of MCMC, it helps approximate distribution-related quantities, such as means or variances.
3. **Convergence**: The process where the chain’s samples approximate the target distribution.
Achieving convergence is crucial for obtaining reliable results from MCMC simulations.
The Relationship between Bayesian Statistics and MCMC
The connection between Bayesian statistics and MCMC lies in the challenge of obtaining the posterior distribution.
Bayesian models often involve complex multivariate distributions, making it difficult to calculate posterior conclusions analytically.
This is where MCMC becomes indispensable.
MCMC algorithms facilitate practical computation by generating samples from the posterior distribution.
These samples allow us to estimate various statistical measures such as means, variances, or quantiles even when the posterior is complex or high-dimensional.
Common MCMC Algorithms in Bayesian Inference
There are several popular MCMC algorithms, each with its unique features and applications:
1. **Metropolis-Hastings Algorithm**: This is one of the simplest and most general MCMC methods.
It generates a sequence of samples by proposing a new candidate from a proposal distribution and then accepting or rejecting the candidate based on a specific criterion.
2. **Gibbs Sampling**: Particularly effective when dealing with multivariate distributions, Gibbs sampling iteratively updates each variable conditional on the others.
It’s often used when the full conditional distributions are easier to sample from than the joint distribution.
3. **Hamiltonian Monte Carlo (HMC)**: Utilizes gradients to inform the proposal step, leading to more efficient exploration of the parameter space.
HMC is especially beneficial for high-dimensional problems or models with strong correlations among parameters.
Practical Applications of MCMC in Bayesian Statistics
MCMC methods are widely used across various fields due to their flexibility and robustness in handling complex models:
– **Medical Research**: Estimating effective treatment response by modeling population-level data with patient-level variations.
– **Economics**: Predicting market trends or consumer behavior using hierarchical Bayesian models.
– **Genetics**: Identifying the likelihood of disease-associated genes by integrating various types of genetic data.
– **Machine Learning**: In Bayesian neural networks, MCMC aids in sampling the weight distributions, enhancing model predictions and uncertainty estimation.
Conclusion
Bayesian statistics offers an intuitive and flexible framework for statistical inference, continually evolving with the data it observes.
However, its complexities often necessitate computational tools like MCMC to bring theoretical models to practical application.
MCMC algorithms, by effectively sampling from intricate distributions, make Bayesian inference feasible in real-world scenarios.
The synergy between Bayesian statistics and MCMC drives modern data analysis, enabling more informed decision-making across numerous domains.
資料ダウンロード
QCD調達購買管理クラウド「newji」は、調達購買部門で必要なQCD管理全てを備えた、現場特化型兼クラウド型の今世紀最高の購買管理システムとなります。
ユーザー登録
調達購買業務の効率化だけでなく、システムを導入することで、コスト削減や製品・資材のステータス可視化のほか、属人化していた購買情報の共有化による内部不正防止や統制にも役立ちます。
NEWJI DX
製造業に特化したデジタルトランスフォーメーション(DX)の実現を目指す請負開発型のコンサルティングサービスです。AI、iPaaS、および先端の技術を駆使して、製造プロセスの効率化、業務効率化、チームワーク強化、コスト削減、品質向上を実現します。このサービスは、製造業の課題を深く理解し、それに対する最適なデジタルソリューションを提供することで、企業が持続的な成長とイノベーションを達成できるようサポートします。
オンライン講座
製造業、主に購買・調達部門にお勤めの方々に向けた情報を配信しております。
新任の方やベテランの方、管理職を対象とした幅広いコンテンツをご用意しております。
お問い合わせ
コストダウンが利益に直結する術だと理解していても、なかなか前に進めることができない状況。そんな時は、newjiのコストダウン自動化機能で大きく利益貢献しよう!
(Β版非公開)