- お役立ち記事
- Remaining life prediction method
月間76,176名の
製造業ご担当者様が閲覧しています*
*2025年3月31日現在のGoogle Analyticsのデータより
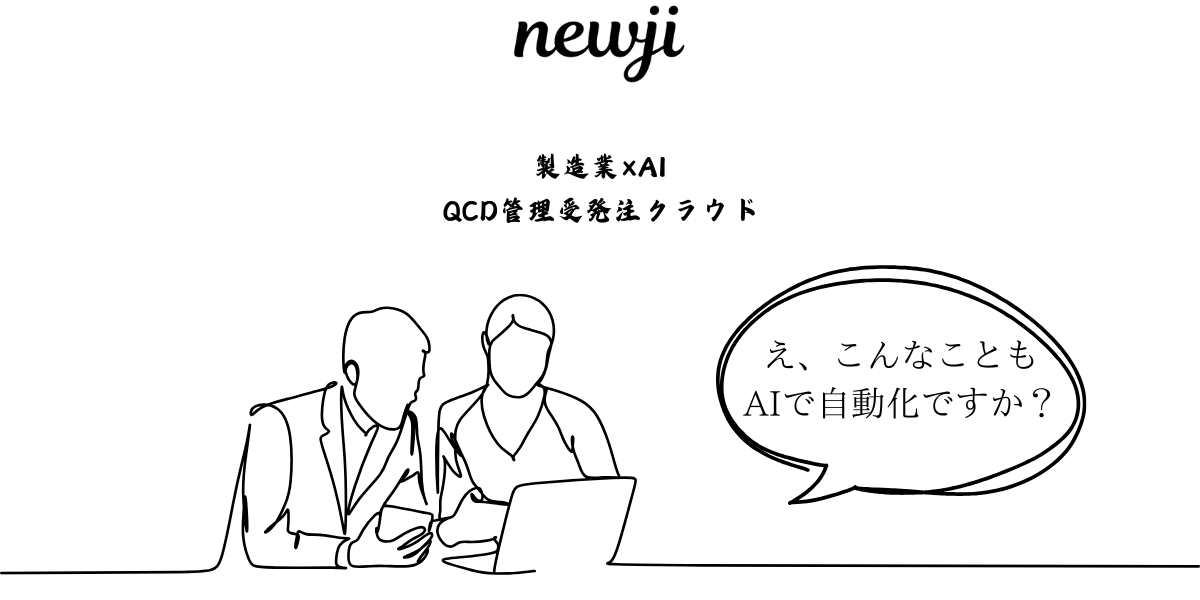
Remaining life prediction method
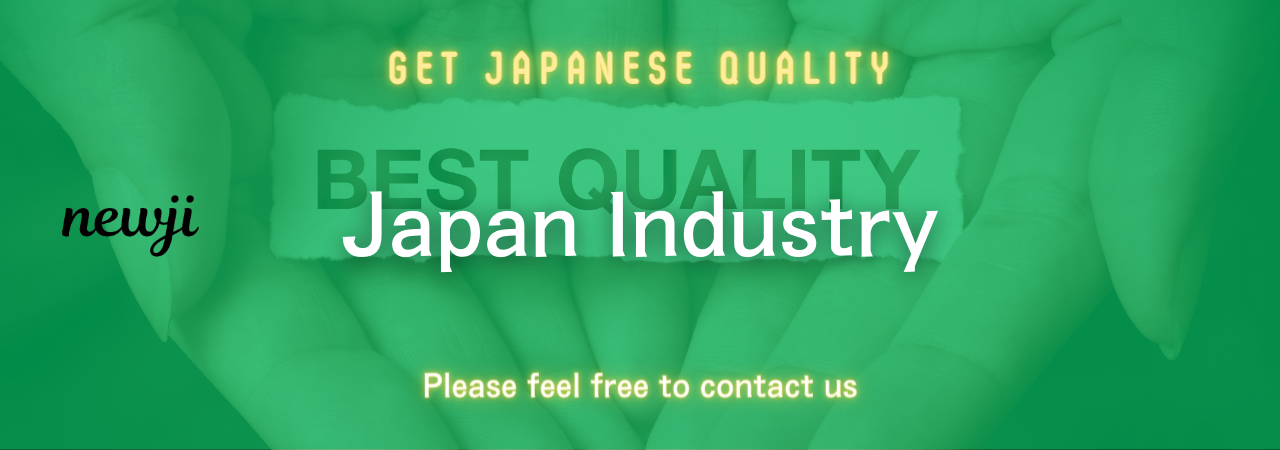
目次
Understanding Remaining Life Prediction
Predicting the remaining life of an object or system involves estimating how long it will continue to function before failure or significant degradation occurs.
This process is crucial across various industries, as it enables organizations to plan maintenance, allocate resources effectively, and ensure safety and reliability.
From aircraft engines to industrial machinery, being able to predict remaining life can save time, reduce costs, and prevent accidents.
The Importance of Remaining Life Prediction
Remaining life prediction is essential for maintaining the efficiency and safety of equipment and systems.
Industries such as aerospace, manufacturing, automotive, and energy rely heavily on accurate predictions to minimize downtime and optimize maintenance schedules.
By anticipating when a component may fail, companies can perform timely repairs or replacements, thereby avoiding unexpected breakdowns and ensuring continuous operation.
Moreover, remaining life prediction contributes to cost reduction.
Unscheduled maintenance is often more expensive than planned interventions.
By predicting remaining life, businesses can spread out maintenance activities, reducing peak demand on resources and personnel.
Methods for Predicting Remaining Life
There are multiple approaches to predicting the remaining life of a system or component.
These methods harness various types of data and analytical techniques to forecast potential failures.
Statistical Methods
Statistical methods involve analyzing historical failure data to predict the probability of future failures.
The Weibull analysis is a commonly used technique in this category.
It models the time to failure for a product, allowing users to calculate the likelihood of failure at different points in time.
Through statistical methods, one can estimate the reliability of components and determine their expected lifespan based on past performance.
Machine Learning and Artificial Intelligence
Recent advancements in technology have introduced machine learning and artificial intelligence (AI) into the realm of remaining life prediction.
These approaches leverage large datasets and sophisticated algorithms to detect patterns and predict failures with high accuracy.
Machine learning models can be trained on historical sensor data, operational conditions, and maintenance records.
These models learn from the data to predict remaining life, identifying potential issues long before they manifest as failures.
Moreover, AI can continuously update its predictions as new data becomes available, making it a dynamic and adaptable tool for remaining life prediction.
Physics-Based Models
Physics-based models rely on understanding the underlying physical mechanisms that lead to wear and failure.
These models use principles from physics and engineering to simulate how components degrade over time under specific conditions.
For example, in the case of mechanical components, analysts can use fatigue analysis to assess how materials react to repeated stress.
This approach provides detailed insights into the degradation process and helps predict the eventual failure point.
Physics-based models are especially useful for systems where failure processes are well understood and where empirical data may be limited.
Hybrid Models
Hybrid models combine elements of statistical, machine learning, and physics-based methods to provide a comprehensive prediction framework.
By integrating multiple approaches, hybrid models can leverage the strengths of each method and offer more robust and accurate predictions.
These models are particularly effective in complex systems where different components may follow different degradation patterns.
Hybrid models can adjust to varying conditions and incorporate real-time data to improve prediction reliability.
Applications and Benefits
The application of remaining life prediction extends across numerous sectors, each with its own set of benefits.
Manufacturing
In manufacturing, equipment downtime can lead to significant production losses.
By predicting remaining life, manufacturers can schedule maintenance during non-peak hours, thereby minimizing impact on production schedules.
Additionally, by ensuring that machinery is in optimal condition, manufacturers can maintain product quality and avoid defects that could arise from equipment failure.
Aerospace
In the aerospace industry, safety is paramount.
Predicting the remaining life of aircraft components ensures that they are replaced or repaired before they pose safety risks.
With accurate predictions, airlines can optimize the service life of parts, balancing safety with operational costs.
This allows for better fleet management and adherence to regulatory compliance.
Energy
Energy providers, whether in oil and gas or renewable sectors, utilize remaining life prediction to ensure the reliability of critical infrastructure.
By forecasting equipment failures, energy companies can reduce the risk of outages and increase the efficiency of power generation.
Furthermore, by optimizing maintenance schedules, energy providers can reduce operational costs and improve the sustainability of their operations.
Transportation and Automotive
In transportation and automotive, remaining life prediction supports the longevity and reliability of vehicles, from commercial fleets to personal cars.
This is crucial for minimizing breakdowns, reducing repair costs, and extending the service life of vehicles.
With reliable predictions, transportation companies can optimize their fleets, enhance safety, and improve customer satisfaction.
Challenges and Considerations
While remaining life prediction offers numerous benefits, it is not without challenges.
Data Quality and Availability
The accuracy of predictions heavily depends on the quality and quantity of data available.
Ensuring reliable and sufficient data for analysis can be challenging, particularly in legacy systems or environments where comprehensive data collection is not standard practice.
Complexity of Systems
Complex systems with many interacting components may require sophisticated models to predict remaining life accurately.
The interdependencies between components can complicate prediction efforts and require advanced modeling techniques.
Changing Operating Conditions
Systems often operate under varying conditions, which can impact the degradation process.
Models must account for these variations to provide reliable predictions across different scenarios.
By understanding and addressing these challenges, industries can maximize the effectiveness of remaining life prediction and harness its full potential for enhancing safety, efficiency, and cost-effectiveness.
資料ダウンロード
QCD管理受発注クラウド「newji」は、受発注部門で必要なQCD管理全てを備えた、現場特化型兼クラウド型の今世紀最高の受発注管理システムとなります。
ユーザー登録
受発注業務の効率化だけでなく、システムを導入することで、コスト削減や製品・資材のステータス可視化のほか、属人化していた受発注情報の共有化による内部不正防止や統制にも役立ちます。
NEWJI DX
製造業に特化したデジタルトランスフォーメーション(DX)の実現を目指す請負開発型のコンサルティングサービスです。AI、iPaaS、および先端の技術を駆使して、製造プロセスの効率化、業務効率化、チームワーク強化、コスト削減、品質向上を実現します。このサービスは、製造業の課題を深く理解し、それに対する最適なデジタルソリューションを提供することで、企業が持続的な成長とイノベーションを達成できるようサポートします。
製造業ニュース解説
製造業、主に購買・調達部門にお勤めの方々に向けた情報を配信しております。
新任の方やベテランの方、管理職を対象とした幅広いコンテンツをご用意しております。
お問い合わせ
コストダウンが利益に直結する術だと理解していても、なかなか前に進めることができない状況。そんな時は、newjiのコストダウン自動化機能で大きく利益貢献しよう!
(β版非公開)