- お役立ち記事
- Revolutionizing Japanese Manufacturing: Harnessing Machine Learning Experimental Design with Active Learning Approaches
月間76,176名の
製造業ご担当者様が閲覧しています*
*2025年3月31日現在のGoogle Analyticsのデータより
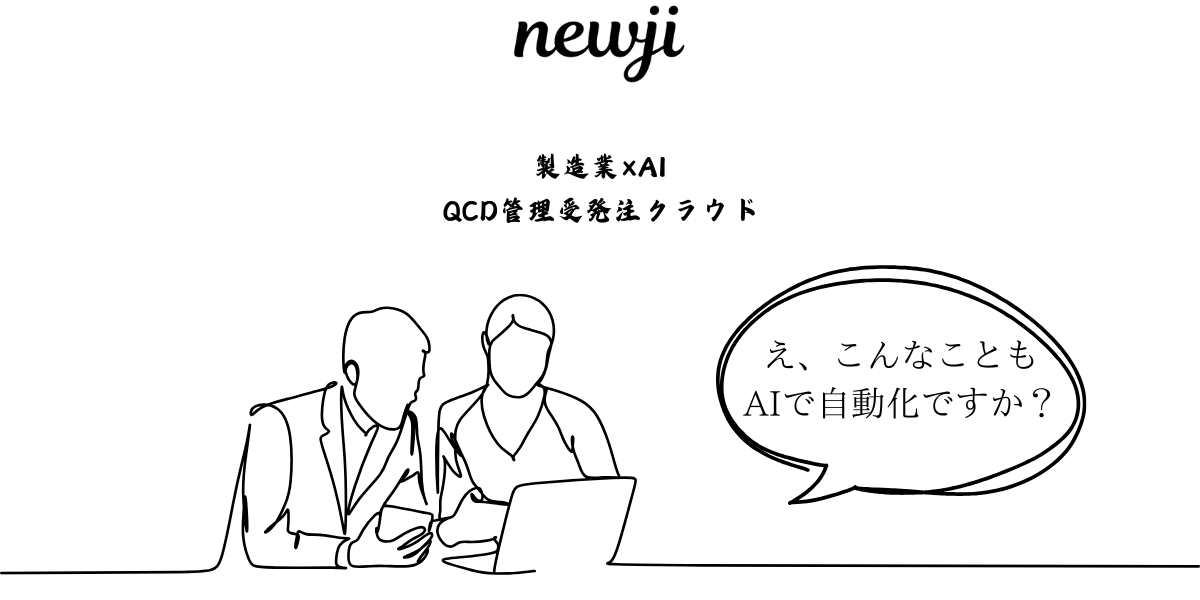
Revolutionizing Japanese Manufacturing: Harnessing Machine Learning Experimental Design with Active Learning Approaches
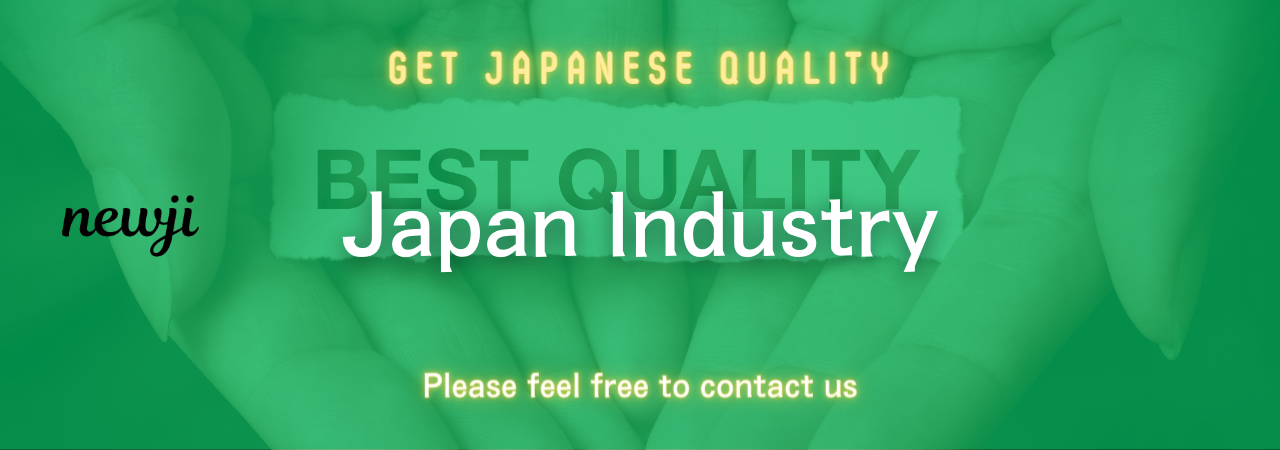
目次
Introduction to Machine Learning in Japanese Manufacturing
Japanese manufacturing has long been synonymous with quality and efficiency.
In recent years, the integration of machine learning (ML) into manufacturing processes has marked a significant shift towards Industry 4.0.
This transformation leverages data-driven insights to optimize operations, enhance product quality, and drive innovation.
Machine learning experimental design, coupled with active learning approaches, is at the forefront of this revolution.
This article explores how these technologies are reshaping Japanese manufacturing, particularly from the perspectives of procurement and purchasing.
Understanding Machine Learning Experimental Design
Machine learning experimental design involves creating structured experiments to train algorithms effectively.
It focuses on selecting the right variables, determining the appropriate data collection methods, and establishing protocols to ensure reliable outcomes.
In manufacturing, this can translate to optimizing production lines, predictive maintenance, and quality control.
Active Learning Approaches in Manufacturing
Active learning is a subset of machine learning where the algorithm selectively queries the most informative data points.
This approach is particularly beneficial in environments where labeled data is scarce or expensive to obtain.
In Japanese manufacturing, active learning can enhance process efficiency by focusing on critical areas that require immediate attention, thereby reducing downtime and improving overall productivity.
Advantages of Implementing ML Experimental Design
The incorporation of ML experimental design in manufacturing offers numerous benefits:
Enhanced Decision-Making
Data-driven insights enable more accurate and timely decisions.
Procurement departments can forecast demand trends, optimize inventory levels, and negotiate better terms with suppliers based on predictive analytics.
Improved Quality Control
Machine learning models can detect defects and inconsistencies in real-time, ensuring that products meet stringent quality standards.
This proactive approach minimizes waste and reduces the costs associated with rework and returns.
Operational Efficiency
Automating routine tasks and optimizing workflows through ML leads to significant time and cost savings.
Manufacturers can streamline their supply chains, reduce lead times, and enhance overall operational performance.
Challenges and Disadvantages
While the benefits are substantial, implementing ML experimental design presents several challenges:
Data Quality and Availability
Effective machine learning models require high-quality, comprehensive data.
Inconsistent data collection practices and data silos can hinder the performance of ML algorithms.
Resistance to Change
Introducing new technologies can face resistance from employees accustomed to traditional methods.
Overcoming this requires comprehensive training and a cultural shift towards embracing innovation.
High Implementation Costs
The initial investment in ML technologies, including hardware, software, and skilled personnel, can be significant.
Balancing these costs with the long-term benefits is crucial for sustainable adoption.
Supplier Negotiation Techniques in the Age of ML
Machine learning is transforming how manufacturers interact with suppliers:
Data-Driven Negotiations
Procurement teams can leverage data analytics to assess supplier performance, pricing trends, and market conditions.
This information empowers them to negotiate more effectively, ensuring favorable terms and reducing procurement costs.
Predictive Supplier Risk Management
ML models can predict potential risks associated with suppliers, such as delays, quality issues, or financial instability.
By anticipating these risks, manufacturers can develop contingency plans and maintain a resilient supply chain.
Enhanced Collaboration
Sharing data insights with suppliers fosters a collaborative environment focused on mutual growth and continuous improvement.
This partnership can lead to innovations in product development and process optimization.
Market Conditions and Trends
The global manufacturing landscape is evolving rapidly, influenced by technological advancements and shifting market dynamics:
Globalization and Supply Chain Complexity
As supply chains become more global, manufacturers face increased complexity in managing suppliers from diverse geographical locations.
Machine learning aids in navigating this complexity by providing real-time visibility and optimization capabilities.
Sustainability and Environmental Concerns
There is a growing emphasis on sustainable manufacturing practices.
ML can help identify opportunities to reduce waste, improve energy efficiency, and minimize the environmental footprint of manufacturing operations.
Customization and Flexibility
Consumers demand more personalized products, necessitating flexible manufacturing processes.
Machine learning facilitates the customization of production lines to accommodate varied product specifications without sacrificing efficiency.
Best Practices for Implementing ML in Procurement and Purchasing
To successfully integrate machine learning into procurement and purchasing, manufacturers should consider the following best practices:
Invest in Quality Data Infrastructure
Establish robust data collection and management systems to ensure the availability of high-quality data.
This foundation is essential for building effective machine learning models.
Foster a Culture of Innovation
Encourage employees to embrace new technologies and methodologies.
Provide training and support to facilitate the transition and promote continuous learning.
Collaborate with Technology Partners
Partner with technology providers and research institutions to stay abreast of the latest advancements.
Collaborations can accelerate the implementation process and provide access to specialized expertise.
Start with Pilot Projects
Begin with small-scale pilot projects to test the feasibility and impact of machine learning applications.
Evaluate the outcomes and scale successful initiatives across the organization.
Ensure Compliance and Data Security
Prioritize data privacy and comply with relevant regulations.
Implement robust cybersecurity measures to protect sensitive information from breaches and unauthorized access.
Case Studies: Successful ML Integration in Japanese Manufacturing
Examining real-world examples provides valuable insights into the practical application of machine learning in manufacturing:
Automotive Industry
A leading Japanese automotive manufacturer implemented ML algorithms to optimize its assembly line.
By predicting maintenance needs and scheduling repairs proactively, the company reduced downtime by 15% and improved overall production efficiency.
Electronics Manufacturing
An electronics component supplier utilized active learning approaches to enhance quality control.
The ML models accurately identified defects in real-time, resulting in a 20% decrease in product returns and increased customer satisfaction.
Precision Machinery
A precision machinery manufacturer adopted machine learning for supply chain optimization.
By analyzing supplier performance data, the company negotiated better terms and reduced procurement costs by 10%, while maintaining high-quality standards.
Future Outlook: The Evolving Role of ML in Japanese Manufacturing
The future of Japanese manufacturing is intrinsically linked to the continued evolution of machine learning technologies:
Integration with IoT and Industry 4.0
The convergence of ML with the Internet of Things (IoT) and other Industry 4.0 technologies will create more interconnected and intelligent manufacturing environments.
This integration will further enhance operational efficiency and enable more sophisticated data analytics.
Advanced Predictive Analytics
As ML algorithms become more advanced, their predictive capabilities will improve, allowing for even more accurate forecasting and proactive decision-making in procurement and purchasing.
AI-Driven Innovation
Artificial Intelligence (AI) will drive innovation in product design, process optimization, and customer service.
Manufacturers will leverage AI to stay competitive in a rapidly changing market landscape.
Sustainable Manufacturing Practices
Machine learning will play a pivotal role in achieving sustainability goals by optimizing resource usage, reducing waste, and minimizing environmental impact.
Conclusion
The integration of machine learning experimental design and active learning approaches is revolutionizing Japanese manufacturing.
From enhancing procurement and purchasing processes to driving operational efficiency and innovation, machine learning offers a myriad of benefits.
While challenges such as data quality, implementation costs, and cultural resistance exist, the strategic adoption of ML technologies can lead to significant competitive advantages.
By embracing these advanced methodologies, Japanese manufacturers can continue to uphold their legacy of excellence while spearheading the future of global manufacturing.
資料ダウンロード
QCD管理受発注クラウド「newji」は、受発注部門で必要なQCD管理全てを備えた、現場特化型兼クラウド型の今世紀最高の受発注管理システムとなります。
ユーザー登録
受発注業務の効率化だけでなく、システムを導入することで、コスト削減や製品・資材のステータス可視化のほか、属人化していた受発注情報の共有化による内部不正防止や統制にも役立ちます。
NEWJI DX
製造業に特化したデジタルトランスフォーメーション(DX)の実現を目指す請負開発型のコンサルティングサービスです。AI、iPaaS、および先端の技術を駆使して、製造プロセスの効率化、業務効率化、チームワーク強化、コスト削減、品質向上を実現します。このサービスは、製造業の課題を深く理解し、それに対する最適なデジタルソリューションを提供することで、企業が持続的な成長とイノベーションを達成できるようサポートします。
製造業ニュース解説
製造業、主に購買・調達部門にお勤めの方々に向けた情報を配信しております。
新任の方やベテランの方、管理職を対象とした幅広いコンテンツをご用意しております。
お問い合わせ
コストダウンが利益に直結する術だと理解していても、なかなか前に進めることができない状況。そんな時は、newjiのコストダウン自動化機能で大きく利益貢献しよう!
(β版非公開)