- お役立ち記事
- road area detection
road area detection
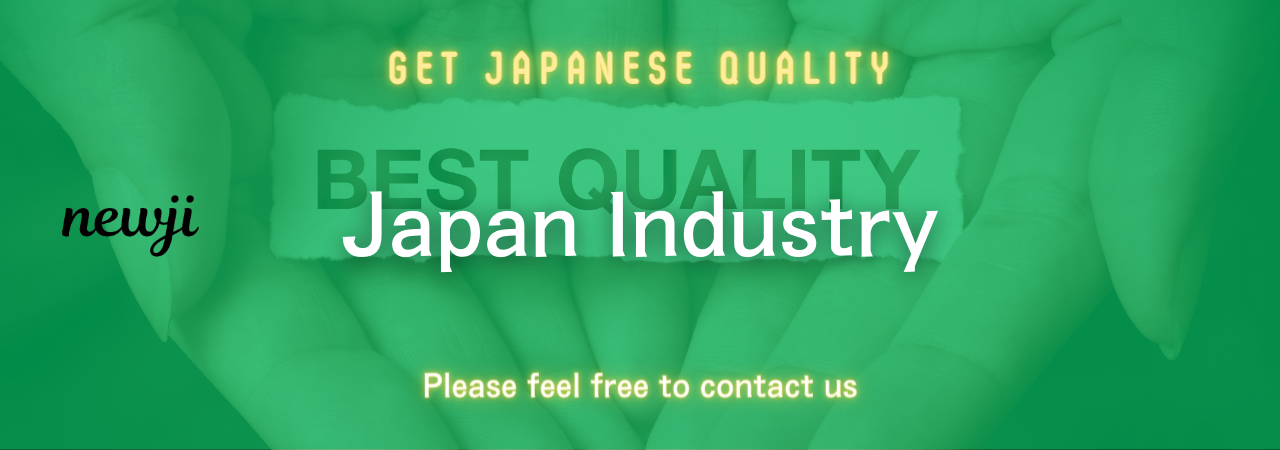
目次
Understanding Road Area Detection
Road area detection has become a crucial area of focus as we steadily move towards autonomous vehicles and smarter cities.
It ensures the safe navigation of roads by enabling vehicles and drones to understand their surroundings.
Utilizing advanced technologies, this system effectively identifies and differentiates the road area from non-road regions.
What is Road Area Detection?
Road area detection refers to the ability of a system, often powered by artificial intelligence, to identify which parts of an image or video belong to the road.
It plays a pivotal role in fields like autonomous driving, traffic monitoring, and urban planning.
By recognizing the road areas, these systems can make informed decisions, enhancing safety and efficiency.
Why is Road Area Detection Important?
Road area detection is vital for numerous reasons.
Foremost, it aids in the development of self-driving cars, providing them with the necessary vision to navigate roads safely.
This technology ensures that vehicles remain within lane boundaries and avoid obstacles.
Additionally, it contributes to improving traffic management systems.
By accurately detecting road areas, the system can communicate real-time data to manage traffic flows, reduce congestion, and enhance overall road safety.
Urban planners also benefit from accurate road area detection as it helps in designing smarter cities by optimizing road layouts and infrastructure.
How Does Road Area Detection Work?
Several technologies are employed to achieve effective road area detection, often combining multiple sources of data.
Image Processing Techniques
Image processing involves analyzing the visual elements of a captured image or video to discern road areas.
This technique often includes methods like edge detection, color segmentation, and texture analysis.
These methods help in classifying and segmenting different parts of the image, isolating the road from its surroundings.
Machine Learning Models
Machine learning models, specifically convolutional neural networks (CNNs), have become integral in enhancing road area detection.
These models are trained on massive datasets containing images labeled as road or non-road.
Through training, they learn to identify patterns and features that distinguish roads from other objects.
LiDAR and Radar Sensors
LiDAR and radar sensors are employed for their precision and reliability.
LiDAR uses laser light to measure distances to objects, creating detailed 3D maps of the environment.
When combined with machine learning models, these maps enable vehicles to distinctly recognize road boundaries and obstacles.
Radar sensors, although not as detailed as LiDAR, provide data useful in identifying larger objects, boosting system reliability under varying weather conditions.
Applications of Road Area Detection
Road area detection technology finds applications across various domains, making significant impacts:
Autonomous Vehicles
Self-driving cars heavily rely on road area detection to navigate safely and efficiently.
It assists in lane detection, avoiding obstacles, and understanding traffic signals.
This technology ensures that autonomous vehicles can coexist with human-driven vehicles by matching their adaptability and safety.
Traffic Monitoring
Incorporating road area detection in traffic monitoring systems leads to better management of traffic flows.
By analyzing real-time road conditions, traffic lights can be optimized, reducing jams and improving travel times.
It also aids in detecting accidents promptly, enabling quicker response times for assistance.
Urban Planning and Development
Urban planners utilize road area detection for designing cities with efficient transportation networks.
By analyzing road layouts, planners can optimize traffic management systems, ensuring smooth flow and reducing bottlenecks.
Moreover, it aids in integrating new technologies, like bike lanes and pedestrian paths, promoting sustainable travel options.
The Future of Road Area Detection
As we advance toward a future dominated by technology-driven solutions, road area detection will continue to evolve.
Integration with Other Technologies
The integration of road area detection with emerging technologies like Internet of Things (IoT) and 5G network will lead to more comprehensive smart city solutions.
These integrations will facilitate real-time communication between vehicles, infrastructure, and traffic management systems, improving overall efficiency.
Advancements in AI Models
Continued advancements in artificial intelligence and machine learning models will enhance the accuracy and reliability of road area detection systems.
More efficient algorithms and increased computational power will enable these systems to process data faster and make more precise decisions.
Conclusion
Road area detection stands as a significant technological advancement with immense possibilities for improvement.
From autonomous vehicles and traffic management to urban planning, the applications are vast and transformative.
As these systems become more sophisticated, they promise a future of safer, more efficient, and smarter cities.
資料ダウンロード
QCD調達購買管理クラウド「newji」は、調達購買部門で必要なQCD管理全てを備えた、現場特化型兼クラウド型の今世紀最高の購買管理システムとなります。
ユーザー登録
調達購買業務の効率化だけでなく、システムを導入することで、コスト削減や製品・資材のステータス可視化のほか、属人化していた購買情報の共有化による内部不正防止や統制にも役立ちます。
NEWJI DX
製造業に特化したデジタルトランスフォーメーション(DX)の実現を目指す請負開発型のコンサルティングサービスです。AI、iPaaS、および先端の技術を駆使して、製造プロセスの効率化、業務効率化、チームワーク強化、コスト削減、品質向上を実現します。このサービスは、製造業の課題を深く理解し、それに対する最適なデジタルソリューションを提供することで、企業が持続的な成長とイノベーションを達成できるようサポートします。
オンライン講座
製造業、主に購買・調達部門にお勤めの方々に向けた情報を配信しております。
新任の方やベテランの方、管理職を対象とした幅広いコンテンツをご用意しております。
お問い合わせ
コストダウンが利益に直結する術だと理解していても、なかなか前に進めることができない状況。そんな時は、newjiのコストダウン自動化機能で大きく利益貢献しよう!
(Β版非公開)