- お役立ち記事
- Safety evaluation methods for automated driving systems to achieve Level 4 and application to safety design
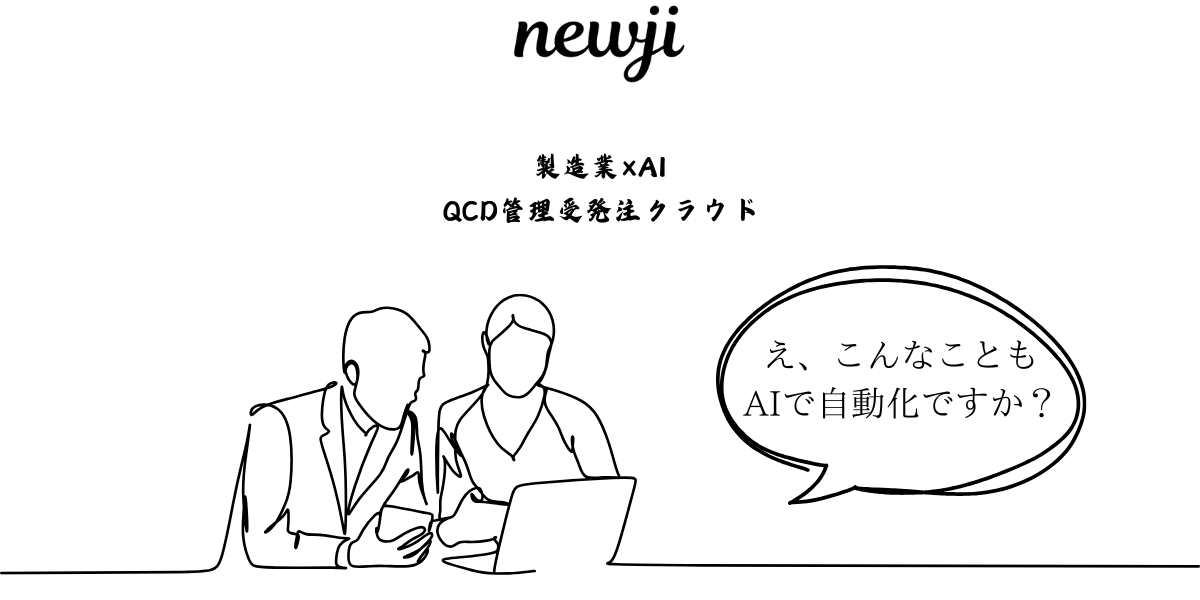
Safety evaluation methods for automated driving systems to achieve Level 4 and application to safety design
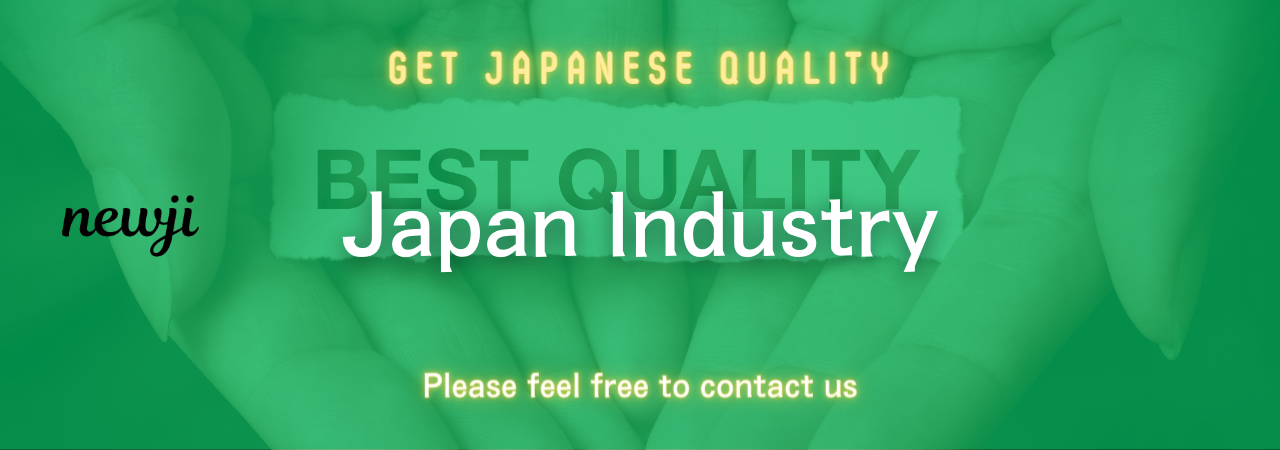
目次
Introduction to Automated Driving Systems
Automated driving systems have rapidly evolved, with advancements in technology paving the way for vehicles to handle increasingly complex driving tasks.
A major area of focus is developing systems that can achieve Level 4 autonomy, where vehicles can operate without human intervention within certain conditions or environments.
As these systems approach mass production and deployment, ensuring their safety becomes paramount.
Evaluating the safety of automated driving systems is crucial not only for consumer confidence but also for regulatory approvals.
Understanding Level 4 Autonomy
Level 4 autonomy, as defined by the Society of Automotive Engineers (SAE), means a vehicle can perform all driving tasks under specific conditions.
This includes navigating urban environments, understanding road signs, and responding appropriately to unexpected obstacles.
Level 4 vehicles are designed to operate without any human input, making it essential for the systems to be rigorously tested for safety.
Achieving Level 4 autonomy requires a robust combination of sensors, artificial intelligence, and machine learning algorithms to interpret and respond to real-world scenarios.
Significance of Safety Evaluation
Safety evaluation for Level 4 automated driving systems is vital for several reasons.
Firstly, it ensures that vehicles can handle complex situations without compromising the safety of passengers, pedestrians, and other road users.
Additionally, safety evaluation helps gain regulatory approval and public trust, which are necessary for wide-scale adoption.
It involves testing how the system reacts to a myriad of situations, including emergencies, diverse weather conditions, and other challenges that a human driver would typically encounter.
Challenges in Safety Evaluation
One of the main challenges in evaluating the safety of automated driving systems is replicating the unpredictability of real-world environments.
Unlike human drivers, automated systems must rely entirely on sensors and pre-programmed responses, which may not always predict every possible scenario.
Furthermore, the transition of control in any unexpected situation needs to be smooth and reliable to maintain safety at all times.
Another hurdle is the ethical aspect, in making decisions akin to moral dilemmas that a human driver may face.
These complexities make the safety evaluation of automated systems not only a technical challenge but a philosophical one as well.
Methods for Safety Evaluation
Several methods are utilized to evaluate the safety of Level 4 autonomous systems.
Simulation and Virtual Testing
Simulation is an integral part of the safety evaluation process for automated driving systems.
By creating virtual environments, developers can test the system’s performance in a multitude of scenarios without the risks associated with real-world testing.
These simulations can encompass a variety of weather conditions, road types, and traffic situations.
It allows for continuous testing and improvements without the logistical constraints of using physical vehicles on roads.
Simulation helps in identifying software issues, sensor inaccuracies, and other potential malfunctions while saving time and resources.
Real-World Testing
While simulations are invaluable, real-world testing remains a crucial step in the safety evaluation of automated systems.
Actual road tests help validate the data gathered from simulations and assess the system’s response to real-world stimuli.
Such tests should cover diverse routes and conditions, including urban and rural environments, varying weather conditions, and peak traffic times.
Real-world testing also helps evaluate the interaction between automated vehicles and human-controlled ones, ensuring safe integration on public roads.
Data-Driven Analysis
Automated driving systems generate massive amounts of data that can be leveraged for safety evaluation.
Data-driven analysis helps in identifying patterns, potential risks, and areas that require improvement.
Using machine learning and big data analytics, developers can continuously refine and upgrade their systems to enhance safety and performance.
Data-driven approaches also allow for the assessment of long-term performance and highlight opportunities to improve or modify system behaviors.
Application to Safety Design
Translating safety evaluation outcomes into safety design is essential for the production of reliable automated systems.
Improving Sensor Technologies
Safety evaluations often reveal the need for enhanced sensor technologies.
Accurate sensors are critical for detecting objects, road conditions, and other vehicles.
Upgrades to radar, LIDAR, cameras, and ultrasonic sensors can significantly improve detection and response capabilities.
Investing in sensors that provide better resolution, range, and reliability is vital for achieving Level 4 autonomy.
Enhancing Software Algorithms
The algorithms running autonomous systems must be capable of real-time decision-making.
Safety design should include continuous updates and improvements to these algorithms based on evaluation results.
Efforts should be made to improve the system’s ability to predict the actions of other road users, manage unexpected situations, and ensure the redundancy of critical systems.
Robust software minimizes the chances of failure and ensures a consistent, safe operation under various conditions.
Adopting a Human-Centric Approach
Finally, safety design should consider the end-users — human passengers.
Creating interfaces that are intuitive and allow for smooth transition controls can greatly enhance user trust and acceptance.
Ensuring occupants are informed about the vehicle’s status and upcoming maneuvers contributes to a safer and more reassuring experience.
The integration of a human-centric approach includes designing systems that foresee potential comfort issues and adapt likewise to provide a pleasant ride.
Conclusion
Safety evaluation methods are a pivotal component in the march towards Level 4 automated driving systems.
The blend of advanced simulations, rigorous real-world testing, and thorough data analysis form the backbone of ensuring these systems are safe for public roads.
Translating these evaluations into effective safety designs involves improving sensor accuracy, enhancing decision-making software, and focusing on user experience.
As these pivotal advancements continue, achieving Level 4 autonomy becomes a closer reality, paving the way for safer, smarter transportation for everyone.
資料ダウンロード
QCD調達購買管理クラウド「newji」は、調達購買部門で必要なQCD管理全てを備えた、現場特化型兼クラウド型の今世紀最高の購買管理システムとなります。
ユーザー登録
調達購買業務の効率化だけでなく、システムを導入することで、コスト削減や製品・資材のステータス可視化のほか、属人化していた購買情報の共有化による内部不正防止や統制にも役立ちます。
NEWJI DX
製造業に特化したデジタルトランスフォーメーション(DX)の実現を目指す請負開発型のコンサルティングサービスです。AI、iPaaS、および先端の技術を駆使して、製造プロセスの効率化、業務効率化、チームワーク強化、コスト削減、品質向上を実現します。このサービスは、製造業の課題を深く理解し、それに対する最適なデジタルソリューションを提供することで、企業が持続的な成長とイノベーションを達成できるようサポートします。
オンライン講座
製造業、主に購買・調達部門にお勤めの方々に向けた情報を配信しております。
新任の方やベテランの方、管理職を対象とした幅広いコンテンツをご用意しております。
お問い合わせ
コストダウンが利益に直結する術だと理解していても、なかなか前に進めることができない状況。そんな時は、newjiのコストダウン自動化機能で大きく利益貢献しよう!
(Β版非公開)