- お役立ち記事
- Self-location estimation technology and map matching
Self-location estimation technology and map matching
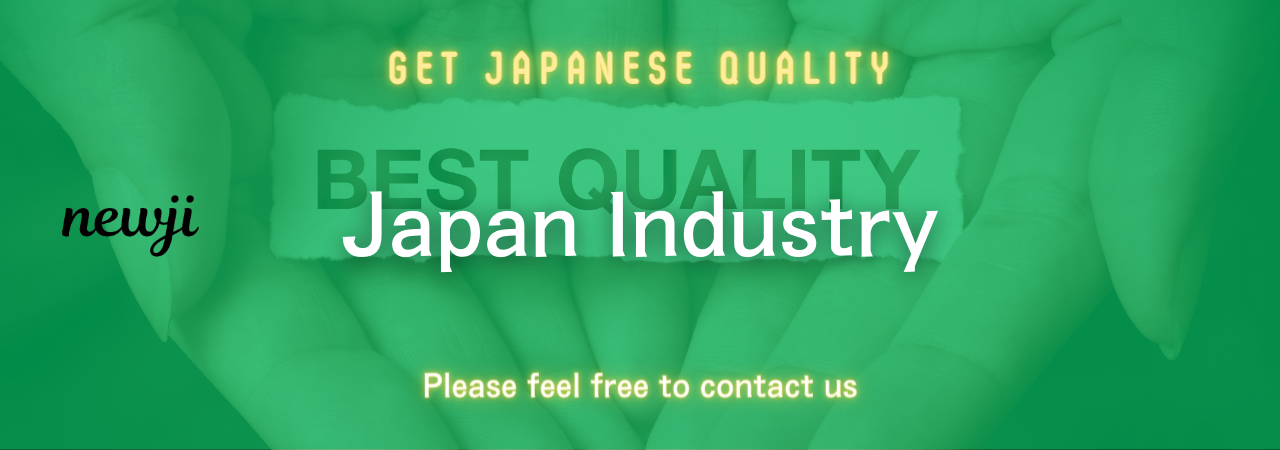
目次
Understanding Self-Location Estimation Technology
Self-location estimation technology is a fascinating field that allows devices to determine their geographical position.
This technology is integral to numerous applications, from navigation systems in vehicles to smartphone apps that guide us through unfamiliar cities.
The primary goal of self-location estimation is to accurately determine where an object or person is located in real-time.
How Self-Location Estimation Works
To understand how self-location estimation works, let’s look at its fundamental components.
This technology typically relies on a combination of GPS (Global Positioning System), sensors, and algorithms.
GPS provides satellite-based data, offering a broad overview of a device’s location, but it works best when the signal is unobstructed.
However, GPS alone is often not enough for precise location estimation.
This is where additional sensors such as accelerometers and gyroscopes come into play, which measure movements and orientation.
Algorithms then process all this data to estimate the current location.
These algorithms consider various factors, like signal strength and environmental changes, to refine accuracy.
The use of online maps can further enhance this estimation, providing context and corrective adjustments for challenges such as signal loss or obfuscations by buildings.
Map Matching for Enhanced Accuracy
Map matching is a crucial component that dramatically enhances self-location estimation technology.
In essence, map matching takes raw location data and aligns it with geographical information on a digital map.
Why Map Matching is Important
Without map matching, location estimations are prone to errors, especially in urban environments with tall buildings blocking satellite signals.
Errors could even arise from slight deviations in satellite transmissions.
Map matching mitigates these issues by using known map features, like roads and walkways, to correct location data.
This technique not only recalibrates the raw data to known pathways but also helps determine the most likely routes taken, making the navigation experience more intuitive and reliable for the user.
How Map Matching Works
Map matching uses several steps to ensure that estimated locations are accurate:
1. Collecting Raw Data: This involves gathering data from GPS and sensors to determine the initial position.
2. Mapping Data Points: These raw data points are then plotted onto a digital map.
3. Aligning Pathways: The system aligns these data points with the closest road or path on the map, correcting any deviations by considering road networks, directional flows, and logical travel pathways.
4. Refinement: Ongoing feedback from sensors is used to continuously refine this estimated location, helping the device stay on track even in less-than-perfect conditions.
Applications in Everyday Life
The integration of self-location estimation technology and map matching has revolutionized various aspects of daily life.
Navigation Systems
Perhaps the most recognizable application of this technology is in navigation systems.
Devices in vehicles use self-location estimation and map matching to provide real-time guidance, helping drivers smoothly reach their destinations.
These systems can adjust routes based on traffic conditions and unexpected detours, thanks to the accuracy provided by map matching.
Smartphone Applications
Many apps on smartphones rely on self-location estimation to enhance user experiences.
For example, ride-sharing services use this technology to locate both vehicles and passengers accurately.
Fitness apps track running or cycling routes to provide users with detailed fitness metrics.
Emergency Services
Emergency services benefit significantly from the precision of self-location technology.
First responders rely on accurate location data to reach people in need as quickly as possible.
In situations where every second counts, such precision can make a vital difference in saving lives.
Challenges in Self-Location Estimation and Map Matching
Despite its many benefits, self-location estimation technology faces several challenges.
Environmental Constraints
One major issue is environmental constraints.
In dense urban areas or indoors, GPS signals can be weak or obstructed.
This affects the accuracy of location data and poses a hurdle for enhancing reliability.
Data Processing
Processing the enormous amount of data from satellites, sensors, and maps in real-time is computationally demanding.
Optimizing algorithm efficiency is crucial to maintain quick and accurate location updates, especially in scenarios with limited computational resources.
Privacy Concerns
With the ability to track precise locations comes the concern of privacy.
Users need to be assured that their data is being handled securely and ethically, with appropriate consent and transparency regarding its use.
The Future of Self-Location Estimation Technology
As technology advances, the potential for self-location estimation and map matching continues to grow.
Integration with AI
Artificial Intelligence (AI) will play a pivotal role in enhancing this technology.
AI algorithms can predict routes, make sense of contextual data, and even anticipate potential errors before they occur.
This would revolutionize how we interact with our environment, providing better-customized experiences in navigation and beyond.
Improved Accuracy
Advancements in sensor technology and improved satellite infrastructures will lead to even more accurate location estimations.
Innovations like the use of 5G networks promise to enhance the precision and speed of data transmission, supporting more reliable and real-time location updates.
Extended Applications
Beyond navigation, applications could expand into fields like autonomous vehicles, robotics, and augmented reality.
These areas demand precise location awareness, and advancements in this technology will pave the way for new developments and innovations.
In conclusion, self-location estimation technology, complemented by map matching, has and will continue to transform our world.
Its applications are vast and impactful, improving convenience and safety in numerous aspects of life.
As we move forward, embracing these advancements will lead to exciting new possibilities, enriching our daily interactions with the world around us.
資料ダウンロード
QCD調達購買管理クラウド「newji」は、調達購買部門で必要なQCD管理全てを備えた、現場特化型兼クラウド型の今世紀最高の購買管理システムとなります。
ユーザー登録
調達購買業務の効率化だけでなく、システムを導入することで、コスト削減や製品・資材のステータス可視化のほか、属人化していた購買情報の共有化による内部不正防止や統制にも役立ちます。
NEWJI DX
製造業に特化したデジタルトランスフォーメーション(DX)の実現を目指す請負開発型のコンサルティングサービスです。AI、iPaaS、および先端の技術を駆使して、製造プロセスの効率化、業務効率化、チームワーク強化、コスト削減、品質向上を実現します。このサービスは、製造業の課題を深く理解し、それに対する最適なデジタルソリューションを提供することで、企業が持続的な成長とイノベーションを達成できるようサポートします。
オンライン講座
製造業、主に購買・調達部門にお勤めの方々に向けた情報を配信しております。
新任の方やベテランの方、管理職を対象とした幅広いコンテンツをご用意しております。
お問い合わせ
コストダウンが利益に直結する術だと理解していても、なかなか前に進めることができない状況。そんな時は、newjiのコストダウン自動化機能で大きく利益貢献しよう!
(Β版非公開)