- お役立ち記事
- Signal Processing and Noise Reduction for Sensor Data with Applications in Anomaly Detection
月間76,176名の
製造業ご担当者様が閲覧しています*
*2025年3月31日現在のGoogle Analyticsのデータより
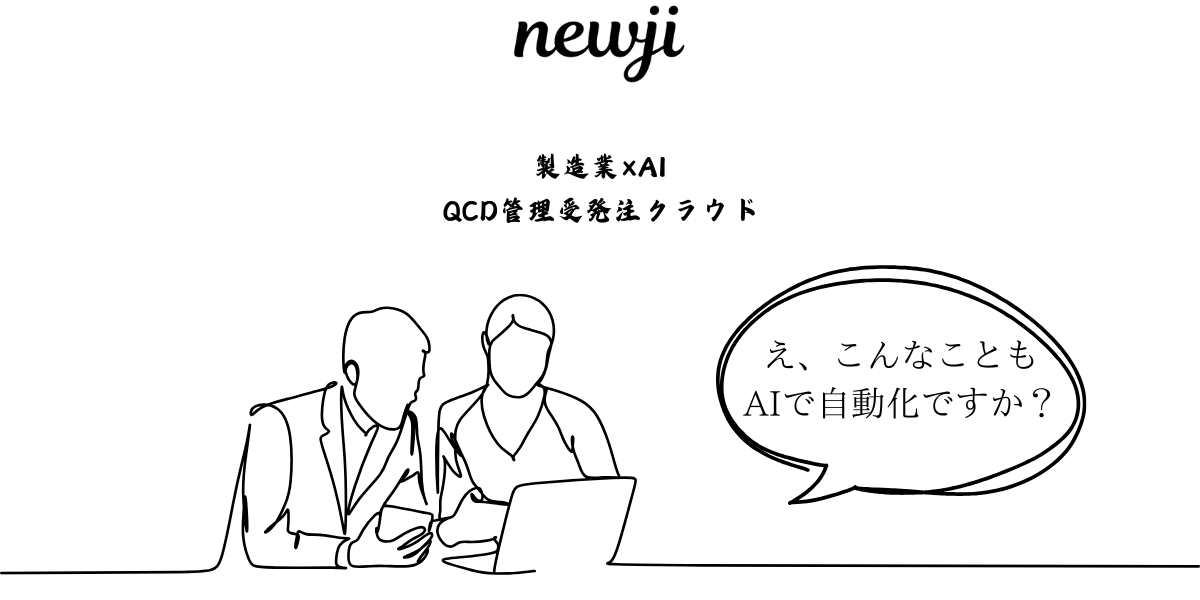
Signal Processing and Noise Reduction for Sensor Data with Applications in Anomaly Detection
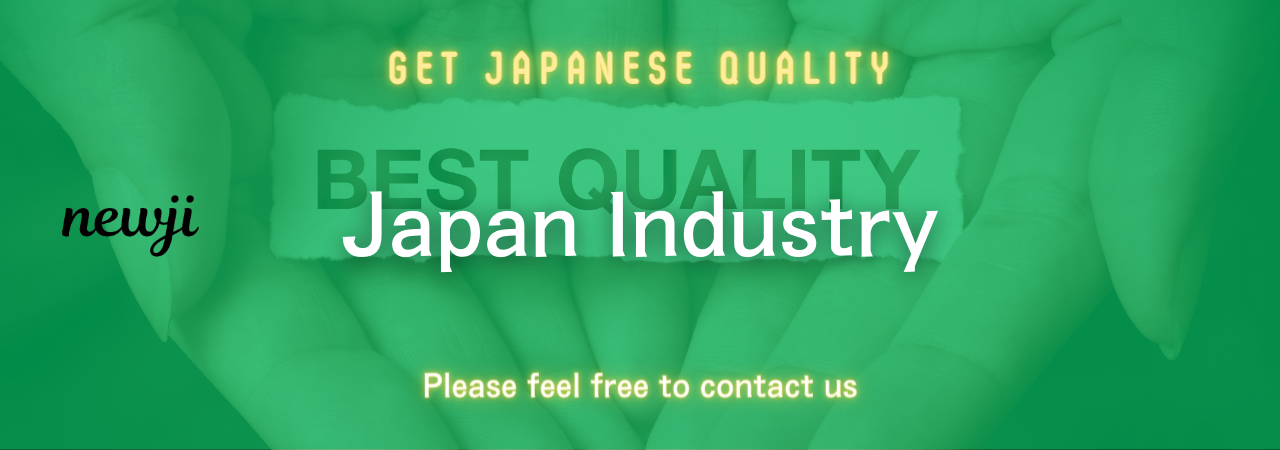
目次
Understanding Signal Processing
Signal processing is a critical aspect of handling sensor data, crucial for analyzing, interpreting, and extracting meaningful insights from noisy and raw data collected from various sources.
At its core, signal processing involves manipulating a signal to improve its quality or extract valuable information.
This can include filtering out unwanted noise, amplifying desired components, and converting data into a more interpretable form.
In many applications, whether in telecommunications, acoustics, or electronics, signal processing techniques help enhance data interpretation.
Components of Signal Processing
Signal processing primarily entails three main components: acquisition, transformation, and analysis.
During the acquisition phase, the signal is captured using sensors, such as microphones for sound or cameras for images.
Following acquisition, transformation processes like filtering or Fourier transform are employed to modify the signal.
These transformations help isolate specific characteristics or remove noise, making the signal more understandable.
Finally, during analysis, various algorithms and methods are applied to interpret the processed signal and extract relevant data insights.
This comprehensive approach allows clear knowledge discovery from jumbled and unclear original data.
The Challenge of Noise
Noise is an undesirable component in a signal that can significantly affect the quality and accuracy of data interpretation.
Sensor data is particularly vulnerable to noise because of environmental factors, such as temperature fluctuations or electromagnetic interference, that may introduce irrelevant information into the dataset.
Inaccurate measurements and improper readings due to noise hinder the effectiveness of decisions based on the collected data.
Therefore, effectively mitigating noise is crucial in signal processing to ensure high-quality and precise information extraction.
Types of Noise in Sensor Data
Different sources contribute to noise in sensor data, including thermal noise, shot noise, and environmental noise.
Thermal noise, for example, is generated by random movements of electrons within sensors, leading to small fluctuations in the signal.
Shot noise, on the other hand, arises from the quantum process of photon absorption in optical sensors.
Environmental noise encompasses external disruptions like radiofrequency interference and vibrations, which can obscure actual sensor readings.
Identifying the type of noise helps in applying the appropriate noise reduction techniques to improve data quality.
Techniques for Noise Reduction
Noise reduction in signal processing involves several methodologies aimed at enhancing signal quality by suppressing unwanted noise components.
Commonly used techniques include filtering, spectral subtraction, and wavelet transformation.
Filtering, such as using low-pass or high-pass filters, allows certain frequency components to pass through while attenuating others, effectively reducing unwanted noise.
Spectral subtraction minimizes noise by estimating the noise spectrum and subtracting it from the signal spectrum.
Wavelet transformation offers a versatile approach by decomposing signals into different frequency components, allowing for precise noise suppression tailored to specific applications.
Adaptive Filtering for Real-Time Processing
Adaptive filtering is a dynamic technique used for updating filtering parameters in real-time based on sensor data characteristics.
Unlike fixed filters, adaptive filters can adjust according to the evolving environment, making it especially useful in changing conditions.
This approach is vital in applications like radar and communications systems, where conditions constantly fluctuate.
Adaptive filtering improves signal-to-noise ratio by continually refining its parameters and ensures more accurate and consistent data interpretation.
Anomaly Detection through Signal Processing
Anomaly detection involves identifying patterns in data that do not conform to expected behavior, an essential task in various fields like finance, cybersecurity, and healthcare.
Signal processing techniques play a pivotal role in detecting such anomalies by enhancing data quality and highlighting deviations from the norm.
By analyzing processed signals, anomalies can be identified through statistical models, rule-based methods, or machine learning algorithms.
Applications in Real-World Scenarios
In healthcare, signal processing enables early detection of irregular heartbeats or abnormal brain activities by scrutinizing medical signals such as ECG or EEG.
In cybersecurity, anomaly detection helps identify abnormal network traffic, suggesting potential security breaches.
Signal processing techniques also enhance predictive maintenance in industrial settings by detecting irregularities in machinery performance before failures occur.
These applications underline the significance of signal processing in uncovering anomalies crucial for timely and effective decision-making.
The Role of Machine Learning
Machine learning algorithms significantly augment signal processing by learning from data patterns to improve anomaly detection accuracy.
These algorithms can automatically identify complex patterns in signals and classify anomalies more efficiently than traditional methods.
Integrating machine learning allows for building robust models that evolve over time and continuously improve their detection capabilities.
Combined with signal processing, machine learning strengthens interpretative power, offering a comprehensive anomaly detection system adaptable across various industries.
Future Directions and Innovations
Advancements in artificial intelligence and machine learning are paving the way for more sophisticated signal processing techniques.
Researchers are focusing on developing more adaptive methods that can seamlessly handle vast and noisy datasets.
Emerging technologies like edge computing are enhancing real-time signal processing capabilities, crucial for applications demanding immediate data interpretation.
The confluence of these innovations continues to push boundaries, offering more refined solutions for noise reduction and anomaly detection.
Understanding and applying techniques in signal processing and anomaly detection empower industries to derive clearer insights from sensor data.
Addressing the challenges of noise and leveraging advanced processing methods are vital steps in transforming raw data into reliable, actionable information.
資料ダウンロード
QCD管理受発注クラウド「newji」は、受発注部門で必要なQCD管理全てを備えた、現場特化型兼クラウド型の今世紀最高の受発注管理システムとなります。
ユーザー登録
受発注業務の効率化だけでなく、システムを導入することで、コスト削減や製品・資材のステータス可視化のほか、属人化していた受発注情報の共有化による内部不正防止や統制にも役立ちます。
NEWJI DX
製造業に特化したデジタルトランスフォーメーション(DX)の実現を目指す請負開発型のコンサルティングサービスです。AI、iPaaS、および先端の技術を駆使して、製造プロセスの効率化、業務効率化、チームワーク強化、コスト削減、品質向上を実現します。このサービスは、製造業の課題を深く理解し、それに対する最適なデジタルソリューションを提供することで、企業が持続的な成長とイノベーションを達成できるようサポートします。
製造業ニュース解説
製造業、主に購買・調達部門にお勤めの方々に向けた情報を配信しております。
新任の方やベテランの方、管理職を対象とした幅広いコンテンツをご用意しております。
お問い合わせ
コストダウンが利益に直結する術だと理解していても、なかなか前に進めることができない状況。そんな時は、newjiのコストダウン自動化機能で大きく利益貢献しよう!
(β版非公開)