- お役立ち記事
- Standard system identification method using prediction error method
月間77,185名の
製造業ご担当者様が閲覧しています*
*2025年2月28日現在のGoogle Analyticsのデータより
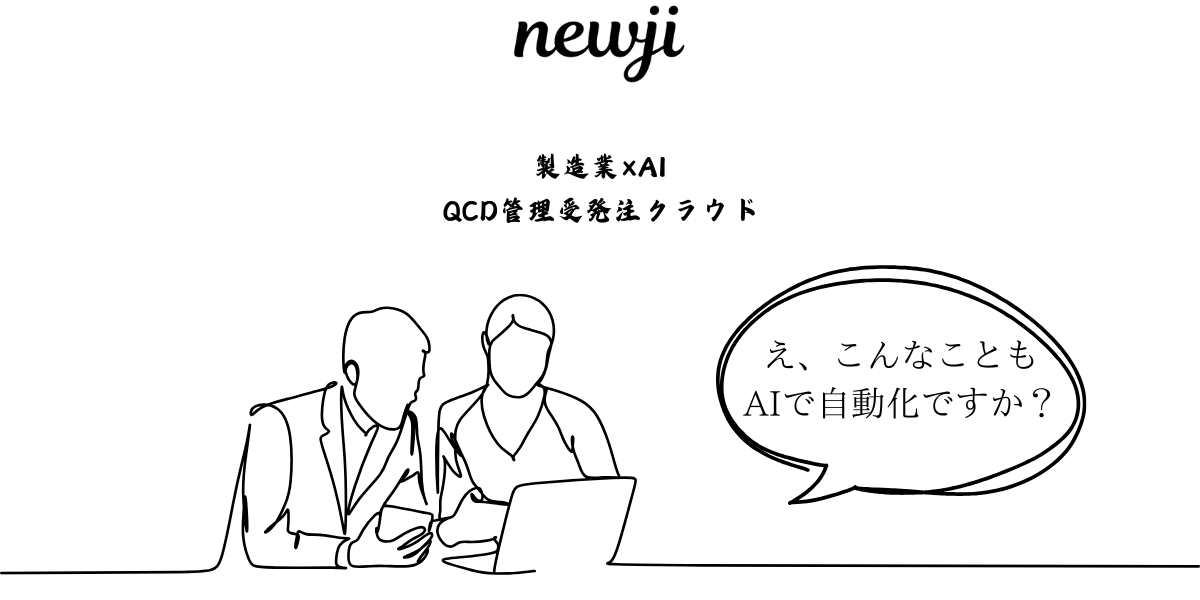
Standard system identification method using prediction error method
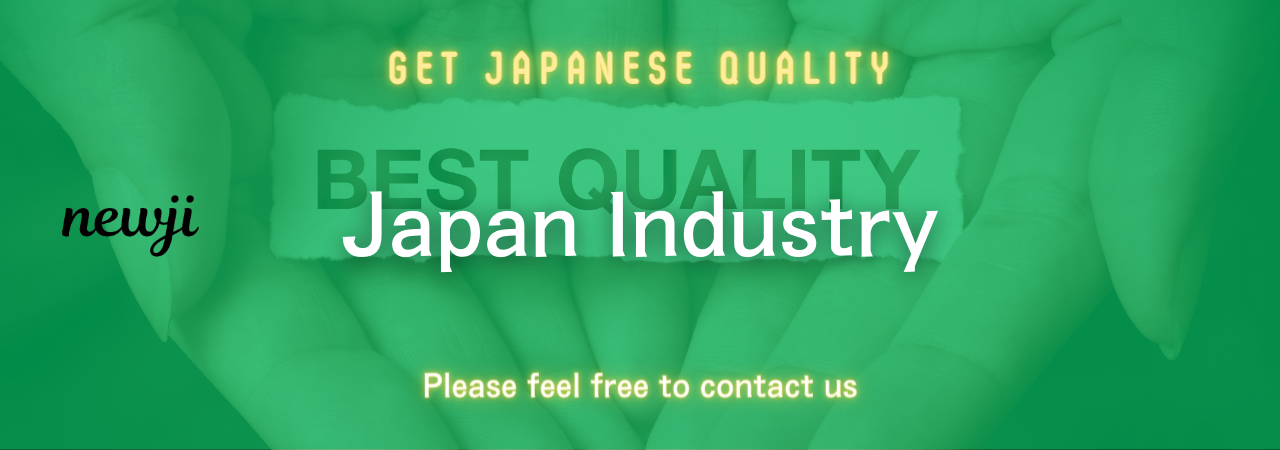
目次
Introduction to System Identification
System identification is an essential technique in the field of engineering and data science that involves building mathematical models of dynamic systems using measured data.
This process is vital in various applications, from designing control systems to optimizing performance in complex systems.
One of the widely recognized methods in system identification is the prediction error method.
In this article, we will explore the prediction error method as a standard system identification technique.
Understanding the Basics of System Identification
Before delving into the prediction error method, it’s important to understand the basics of system identification.
At its core, system identification is about determining the underlying model of a system based on its input and output data.
The goal is to create a model that accurately represents the system’s behavior.
There are several types of system models, including linear, nonlinear, static, and dynamic models.
The choice of model type depends on the specifics of the system being studied.
Once the appropriate model type is selected, system identification techniques are employed to estimate the parameters of the model.
The Role of Data
Data plays a crucial role in system identification.
It is gathered by observing the system’s input-output relationship over a period.
This data is then used to develop a model that can predict the system’s future behavior.
The accuracy and reliability of the model heavily depend on the quality and quantity of the data gathered.
High-quality data reduces uncertainty and enhances the model’s predictive power.
The Prediction Error Method
The prediction error method (PEM) is a robust technique used to identify system models by minimizing the difference between the measured and predicted outputs of a system.
This difference is known as the prediction error.
PEM falls under the category of parametric estimation methods, where the goal is to estimate the parameters of a predefined model structure.
How PEM Works
The prediction error method works by first hypothesizing a model structure that reflects the system’s input-output relationship.
Common model structures used in PEM include ARX (Auto-Regressive with eXogenous inputs), ARMAX (Auto-Regressive Moving Average with eXogenous inputs), and OE (Output Error).
Each of these structures is characterized by specific parameters that need to be estimated from data.
Once the model structure is chosen, PEM aims to find the set of parameters that minimize the prediction error.
This is typically achieved using numerical optimization algorithms.
These algorithms iteratively adjust the parameters, reducing the sum of squared prediction errors over the dataset.
Parameter Estimation
Parameter estimation is central to the prediction error method.
It involves selecting the optimal parameters that best fit the observed data.
In PEM, the parameter estimation process can be carried out using several optimization techniques.
Some popular optimization methods include the Gauss-Newton algorithm, the Levenberg-Marquardt algorithm, and gradient-based methods.
The choice of optimization algorithm depends on the model structure and the specifics of the system.
These algorithms adjust the parameters iteratively, striving to minimize the prediction error.
Advantages of the Prediction Error Method
The prediction error method offers several advantages in system identification, which have contributed to its popularity and widespread use.
Accuracy and Flexibility
One of the key benefits of PEM is its high accuracy in estimating model parameters.
By minimizing the prediction error, PEM provides precise estimates, resulting in reliable models that accurately reflect system dynamics.
Additionally, PEM is adaptable and can accommodate a variety of model structures, making it applicable to diverse systems and problem types.
Robustness
PEM is known for its robustness in handling noisy and complex data environments.
Systems often operate in environments with noise and uncertainty, and PEM excels in such scenarios by effectively distinguishing between the actual system behavior and noise.
This robustness ensures that the identified models remain reliable even in challenging conditions.
Compatibility with Advanced Techniques
The prediction error method seamlessly integrates with advanced techniques such as machine learning and adaptive control.
This compatibility allows practitioners to enhance model performance and extend the applications of PEM beyond traditional system identification.
Challenges and Considerations
While the prediction error method offers numerous advantages, it is essential to be aware of the challenges and considerations associated with its application.
Model Selection
Choosing the correct model structure is crucial in PEM.
An inappropriate model structure may lead to poor parameter estimates and inaccurate predictions.
Practitioners must carefully evaluate the system requirements and characteristics to select a suitable model.
Computational Complexity
The parameter estimation process in PEM can be computationally intensive, particularly for complex model structures.
Optimization algorithms may require significant computational resources, which can be a limiting factor in some applications.
Sensitivity to Initial Conditions
PEM can be sensitive to initial parameter estimates.
Starting with inaccurate initial estimates may lead to suboptimal parameter estimates or convergence issues.
Practitioners must employ techniques to mitigate sensitivity to initial conditions.
Conclusion
The prediction error method is a powerful and widely used technique in system identification.
Its ability to accurately identify system models by minimizing prediction errors makes it an invaluable tool in various industries.
By understanding its principles, advantages, and challenges, practitioners can harness the full potential of PEM to build reliable and accurate models.
Whether optimizing industrial processes or designing control systems, the prediction error method remains a cornerstone of system identification, driving advancements in engineering and data science.
資料ダウンロード
QCD管理受発注クラウド「newji」は、受発注部門で必要なQCD管理全てを備えた、現場特化型兼クラウド型の今世紀最高の受発注管理システムとなります。
ユーザー登録
受発注業務の効率化だけでなく、システムを導入することで、コスト削減や製品・資材のステータス可視化のほか、属人化していた受発注情報の共有化による内部不正防止や統制にも役立ちます。
NEWJI DX
製造業に特化したデジタルトランスフォーメーション(DX)の実現を目指す請負開発型のコンサルティングサービスです。AI、iPaaS、および先端の技術を駆使して、製造プロセスの効率化、業務効率化、チームワーク強化、コスト削減、品質向上を実現します。このサービスは、製造業の課題を深く理解し、それに対する最適なデジタルソリューションを提供することで、企業が持続的な成長とイノベーションを達成できるようサポートします。
製造業ニュース解説
製造業、主に購買・調達部門にお勤めの方々に向けた情報を配信しております。
新任の方やベテランの方、管理職を対象とした幅広いコンテンツをご用意しております。
お問い合わせ
コストダウンが利益に直結する術だと理解していても、なかなか前に進めることができない状況。そんな時は、newjiのコストダウン自動化機能で大きく利益貢献しよう!
(β版非公開)