- お役立ち記事
- Statistical Modeling for Accurate Prediction and Data Analysis
Statistical Modeling for Accurate Prediction and Data Analysis
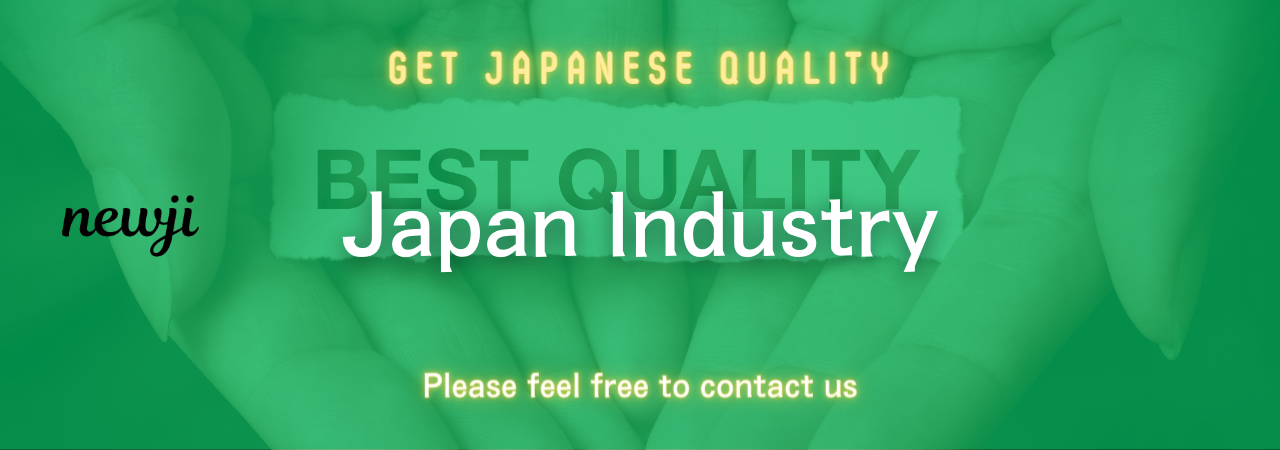
目次
Understanding Statistical Modeling
Statistical modeling is a powerful tool used to predict outcomes and interpret data patterns across various fields.
It involves the creation of mathematical models that represent a process or a system.
This allows researchers and analysts to perform accurate predictions, identify trends, and make informed decisions based on data.
The essence of statistical modeling lies in its ability to simplify complex real-world phenomena into understandable models.
These models can then be used to make predictions about future events or behaviors.
With the advent of big data, statistical modeling has become an integral part of data analysis, providing insights that are crucial for effective decision-making.
Applications of Statistical Modeling
Statistical modeling is used extensively in multiple domains.
In the business world, companies rely on these models to forecast sales, understand customer behavior, and manage risks.
For instance, retail businesses use predictive models to anticipate demand for products, helping them optimize inventory levels and reduce waste.
In healthcare, statistical models assist in predicting disease outbreaks, patient outcomes, and the efficacy of treatments.
Epidemiologists use these models to understand the spread of diseases, which is particularly important in times of a pandemic.
Furthermore, clinicians make use of predictive modeling to personalize treatment plans for patients based on their unique health data.
Financial institutions also benefit from statistical modeling.
Banks and investment firms utilize these models to assess the risk of financial products and make investment decisions.
Credit risk modeling helps lenders decide on interest rates and creditworthiness of borrowers, which directly impacts profitability.
The Process of Creating a Statistical Model
Creating a statistical model involves several systematic steps.
It begins with data collection, where high-quality and relevant data is gathered.
The quality of data directly influences the accuracy and reliability of the model, making it essential to ensure that data is clean and well-organized.
Once data is prepared, the next step involves selecting the appropriate statistical method.
There are numerous methods available, such as linear regression, logistic regression, time series analysis, and more.
The choice of method largely depends on the nature of the data and the specific problem at hand.
Following this, the model is trained and tested on the dataset.
Training involves using a portion of the data to allow the model to learn from it.
Testing, on the other hand, evaluates the model’s performance by assessing its predictions on a separate subset of the data.
This phase is crucial as it helps determine whether a model is accurate and valid.
Model validation is an essential step that involves checking the model’s results against known data to ensure it predicts accurately.
If the model’s predictions are not satisfactory, it may require adjustments or the selection of an alternative modeling technique.
Challenges in Statistical Modeling
While statistical modeling provides numerous benefits, it also presents several challenges.
One of the most significant challenges is dealing with overfitting.
Overfitting occurs when a model is too complex, capturing noise rather than the underlying trend.
This results in poor performance on new, unseen data.
To counteract this, techniques such as cross-validation and using a simpler model structure are often employed.
Another challenge is ensuring data quality.
Missing values, outliers, and incorrect data can significantly skew the results of a model.
It is imperative to perform thorough data cleansing and preprocessing to mitigate these risks.
Moreover, selecting the right modeling technique is crucial.
Different problems may require different approaches, and there is no one-size-fits-all method.
Analysts must have a deep understanding of both the statistical methods and the particular data set to make the right choice.
The Role of Statistical Software
With advancements in technology, statistical software plays a vital role in facilitating the modeling process.
Software tools such as R, Python, SAS, and SPSS offer comprehensive capabilities for building and analyzing statistical models.
These tools come with built-in functions and libraries that allow users to implement complex models more efficiently, handling large datasets seamlessly.
Many of these software solutions also provide user-friendly interfaces, lowering the barrier for those who may not have an extensive statistical background.
This accessibility means that more professionals across different fields can leverage the power of statistical modeling for data-driven decision-making.
Future Trends in Statistical Modeling
As we move towards a more data-centric society, the future of statistical modeling appears promising.
With the rise of artificial intelligence and machine learning, statistical modeling is evolving rapidly.
The integration of machine learning techniques is allowing for the creation of more sophisticated models that learn patterns with less human intervention.
Additionally, with the proliferation of Internet of Things (IoT) devices, there is an increasing amount of real-time data available for analysis.
This trend means that statistical models need to become more adaptable and capable of processing data continuously.
Furthermore, as privacy concerns grow, there is a push towards developing models that can make predictions without compromising sensitive information.
Techniques like federated learning, where algorithms are trained across multiple devices or servers holding local data samples without exchanging them, are gaining traction.
In conclusion, statistical modeling remains an essential tool for predicting outcomes and analyzing data.
Despite its challenges, the continuous evolution of statistical methods and technologies promises a future where models are more accurate, efficient, and widely accessible.
Whether in business, healthcare, finance, or any other field, statistical modeling is indispensable for making informed decisions based on data-driven insights.
資料ダウンロード
QCD調達購買管理クラウド「newji」は、調達購買部門で必要なQCD管理全てを備えた、現場特化型兼クラウド型の今世紀最高の購買管理システムとなります。
ユーザー登録
調達購買業務の効率化だけでなく、システムを導入することで、コスト削減や製品・資材のステータス可視化のほか、属人化していた購買情報の共有化による内部不正防止や統制にも役立ちます。
NEWJI DX
製造業に特化したデジタルトランスフォーメーション(DX)の実現を目指す請負開発型のコンサルティングサービスです。AI、iPaaS、および先端の技術を駆使して、製造プロセスの効率化、業務効率化、チームワーク強化、コスト削減、品質向上を実現します。このサービスは、製造業の課題を深く理解し、それに対する最適なデジタルソリューションを提供することで、企業が持続的な成長とイノベーションを達成できるようサポートします。
オンライン講座
製造業、主に購買・調達部門にお勤めの方々に向けた情報を配信しております。
新任の方やベテランの方、管理職を対象とした幅広いコンテンツをご用意しております。
お問い合わせ
コストダウンが利益に直結する術だと理解していても、なかなか前に進めることができない状況。そんな時は、newjiのコストダウン自動化機能で大きく利益貢献しよう!
(Β版非公開)