- お役立ち記事
- Statistical process control using surrogate modeling and its application to manufacturing process optimization and its key points
Statistical process control using surrogate modeling and its application to manufacturing process optimization and its key points
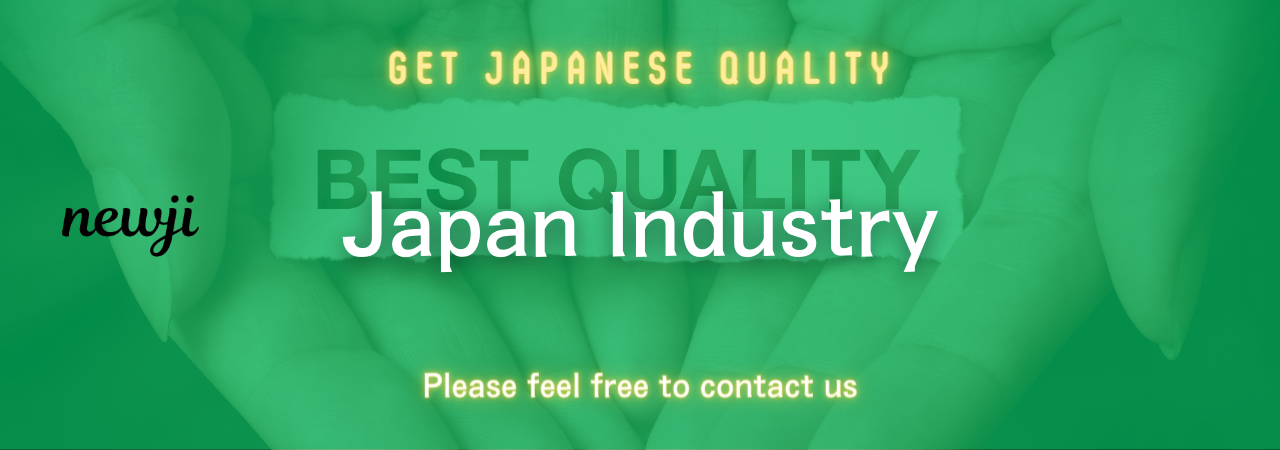
目次
Introduction to Statistical Process Control
Statistical process control (SPC) is a method used to monitor and control a process to ensure that it operates at its fullest potential.
SPC helps to identify and reduce variability in the manufacturing process, leading to higher quality products and less waste.
By employing statistical methods, manufacturers can detect anomalies in the production process in real-time, allowing for immediate corrective measures.
SPC encompasses a wide range of tools and techniques, such as control charts, process capability analysis, and design of experiments.
These methods are designed to ensure that the manufacturing process runs smoothly and efficiently.
This article will explore the role of surrogate modeling in SPC and how it can optimize manufacturing processes.
Understanding Surrogate Modeling
Surrogate modeling involves creating a simplified representation of a complex system.
Surrogate models are used to simulate and analyze systems that are otherwise too expensive or time-consuming to evaluate directly.
These models often take the form of mathematical functions that approximate the behavior of the real system.
In the context of manufacturing, surrogate models can be used to predict the outcomes of different process settings without the need for extensive physical testing.
This makes it possible to experiment with various parameters and optimize the manufacturing process efficiently.
The main advantage of surrogate models is their ability to provide quick and accurate predictions, which are crucial for process optimization.
Different Types of Surrogate Models
There are several types of surrogate models that can be applied in SPC, including polynomial regression models, Kriging models, radial basis functions, and support vector machines.
Each type has its own strengths and is suitable for different kinds of data and objectives.
Polynomial regression models are one of the simplest forms and are suited for processes with linear relationships.
Kriging models, also known as Gaussian process regression, are ideal for more complex, non-linear processes.
Radial basis functions and support vector machines offer more flexibility for capturing non-linear dynamics in the process.
Selecting the appropriate surrogate model depends on the specific characteristics of the manufacturing process and the desired objectives of the optimization effort.
Applying Surrogate Modeling in SPC
When applying surrogate modeling in SPC, the goal is to create a model that accurately represents the manufacturing process.
This model can then be used to identify the best settings to optimize production.
The application process generally involves several steps beginning with data collection.
Collecting accurate and relevant data is essential for training a valid surrogate model.
This data serves as the foundation for building a model that accurately represents the process.
Model Training and Validation
Once data is collected, the next step is model training.
Training the surrogate model involves using historical process data to calibrate the model, aligning it closely with the actual production process.
The model is then validated through testing against additional data to ensure its accuracy.
The validation phase is critical, as it verifies that the surrogate model can reliably predict outcomes.
If the model performs well during validation, it can be used for predicting process outcomes under different conditions.
Optimization and Control
With a validated surrogate model, manufacturers can start the process of optimization.
This involves using the surrogate model to explore different scenarios and adjust process parameters to achieve optimal results.
The goal is to find settings that improve product quality, reduce waste, and increase efficiency.
Surrogate models are particularly useful for evaluating multiple scenarios quickly, which can significantly speed up the process optimization cycle.
One of the consequential steps in optimization is controlling the process based on model predictions.
This might include setting control limits, addressing special-cause variations, and maintaining the desired process state.
Benefits of Using Surrogate Modeling in Manufacturing
The integration of surrogate modeling into SPC offers several benefits for manufacturing processes.
Among these are increased accuracy in process prediction, reduced costs associated with physical experimentation, and faster optimization cycles.
Additionally, surrogate models provide the ability to handle complex processes with multiple variables more efficiently than traditional methods, allowing for a more comprehensive approach to process improvement.
Cost Reduction
By enabling virtual experimentation, surrogate models help reduce the necessity for expensive physical tests.
This not only saves material costs but also minimizes production downtime.
With a robust surrogate model, manufacturers can iterate on design and process changes before implementing them on the production floor.
Improved Quality and Efficiency
The predictive power of surrogate models enhances the ability to maintain high-quality standards.
With better control over process variations, product defects can be minimized, resulting in superior quality end products.
The efficiency of production processes is also increased by identifying optimal operation settings, leading to faster production times and reduced energy consumption.
Challenges and Key Points in Implementation
While surrogate modeling offers numerous benefits, it also presents certain challenges that need to be addressed for successful implementation.
One of the main challenges is selecting appropriate data for model training.
Surrogate models rely heavily on data quality, meaning that any inaccuracies or inconsistencies in data collection can compromise the model’s reliability.
Model Complexity
Another challenge is balancing model complexity with interpretability.
While more complex models may capture process dynamics more closely, they can also become difficult to interpret and manage.
Finding the balance between accuracy and simplicity is key for effective process optimization.
Continuous Monitoring and Adaptation
Finally, continuous monitoring and model adaptation are critical in maintaining the surrogate model’s relevance.
Manufacturing processes can change over time, necessitating regular updates to the model to keep pace with any changes in production dynamics.
These challenges highlight the importance of a strategic approach when integrating surrogate modeling into statistical process control.
Conclusion
Statistical process control, enhanced through surrogate modeling, empowers manufacturers to optimize their production processes effectively.
By providing fast and accurate predictions, surrogate models enable manufacturers to make informed decisions, improve product quality, and increase efficiency while reducing costs.
A well-implemented surrogate model can be a powerful tool, but it requires careful attention to data quality, model selection, and ongoing maintenance.
Through understanding and overcoming the inherent challenges, businesses can reap the benefits of advanced SPC applications and drive their operations towards excellence.
資料ダウンロード
QCD調達購買管理クラウド「newji」は、調達購買部門で必要なQCD管理全てを備えた、現場特化型兼クラウド型の今世紀最高の購買管理システムとなります。
ユーザー登録
調達購買業務の効率化だけでなく、システムを導入することで、コスト削減や製品・資材のステータス可視化のほか、属人化していた購買情報の共有化による内部不正防止や統制にも役立ちます。
NEWJI DX
製造業に特化したデジタルトランスフォーメーション(DX)の実現を目指す請負開発型のコンサルティングサービスです。AI、iPaaS、および先端の技術を駆使して、製造プロセスの効率化、業務効率化、チームワーク強化、コスト削減、品質向上を実現します。このサービスは、製造業の課題を深く理解し、それに対する最適なデジタルソリューションを提供することで、企業が持続的な成長とイノベーションを達成できるようサポートします。
オンライン講座
製造業、主に購買・調達部門にお勤めの方々に向けた情報を配信しております。
新任の方やベテランの方、管理職を対象とした幅広いコンテンツをご用意しております。
お問い合わせ
コストダウンが利益に直結する術だと理解していても、なかなか前に進めることができない状況。そんな時は、newjiのコストダウン自動化機能で大きく利益貢献しよう!
(Β版非公開)