- お役立ち記事
- Successful examples of AI utilization for production line optimization and lessons learned
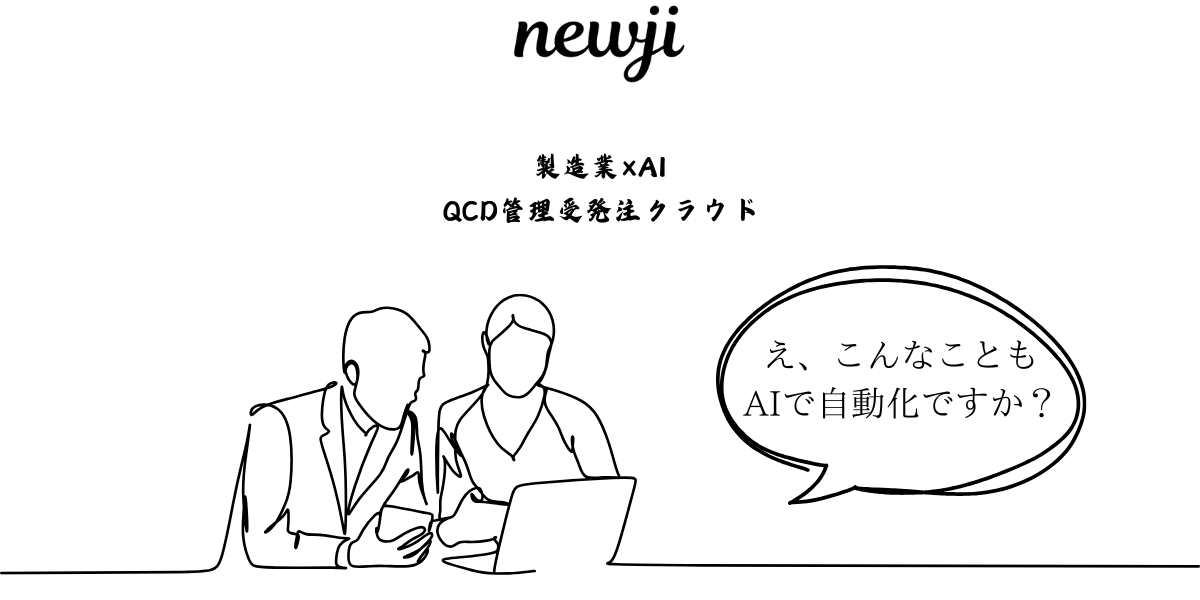
Successful examples of AI utilization for production line optimization and lessons learned
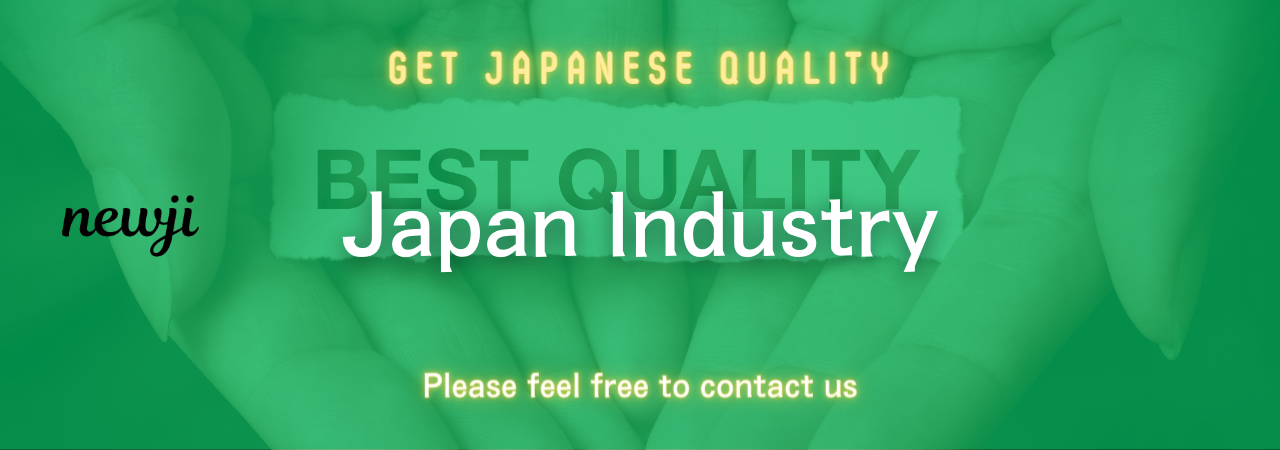
目次
Introduction to AI in Production Line Optimization
Artificial intelligence (AI) is transforming industries worldwide, and production lines are no exception.
As companies strive for efficiency and competitiveness, AI provides innovative solutions to optimize production processes.
The successful integration of AI can lead to reduced operational costs, improved product quality, and accelerated production cycles.
Let’s explore some successful examples of AI in production line optimization and the valuable lessons learned from these implementations.
AI-Driven Predictive Maintenance
One prominent application of AI in production lines is predictive maintenance.
By leveraging machine learning algorithms, companies can predict when equipment is likely to fail and perform maintenance before breakdowns occur.
This proactive approach minimizes downtime and prevents costly disruptions.
For instance, a major automotive manufacturer adopted AI-driven predictive maintenance across its assembly lines.
The AI system analyzed data from sensors embedded in machinery to forecast potential failures.
As a result, the company reduced unexpected machine downtimes by over 30%, significantly boosting overall productivity.
The lesson here is clear: Predictive maintenance not only saves costs but also enhances the reliability of production equipment.
Organizations should prioritize gathering high-quality data from equipment to train AI models effectively.
AI in Quality Control and Assurance
Quality control is crucial in manufacturing to ensure products meet the required standards.
AI offers remarkable capabilities in identifying defects and inconsistencies with greater speed and accuracy compared to human inspection.
Consider the example of a consumer electronics company that implemented AI for quality control.
Their AI system used computer vision to inspect products for defects during the assembly process.
This technology enabled a 40% reduction in product rejection rates due to improved defect detection accuracy.
A critical takeaway from this success is the importance of integrating AI seamlessly into existing quality assurance workflows.
Training AI models to recognize specific product defects is essential for optimal performance, demanding substantial domain-specific data.
AI-Enhanced Demand Forecasting
Accurate demand forecasting is essential for optimal inventory management and production scheduling.
AI algorithms excel at analyzing complex datasets to predict future demand trends, leading to better decision-making.
A leading beverage manufacturer utilized AI-powered demand forecasting to optimize production scheduling.
By analyzing historic sales data and external factors such as weather patterns, the AI model accurately predicted product demand.
This resulted in a 15% reduction in inventory holding costs and a 20% improvement in order fulfillment rates.
The lesson from this example emphasizes the importance of integrating diverse data sources to enhance the accuracy of AI-driven forecasts.
Organizations should combine internal data with external factors to enrich AI predictions and drive higher accuracy.
Robotic Process Automation with AI
Robotic Process Automation (RPA) combined with AI revolutionizes how repetitive and manual tasks are performed on production lines.
Unlike traditional automation, AI-enabled RPA systems can adapt to changes and improve over time.
A food processing company successfully implemented AI-powered RPA for packaging operations.
The intelligent robots adapted to varied packaging formats without manual reprogramming, improving process flexibility.
Consequently, the company observed a 25% increase in packaging efficiency and a significant reduction in manual labor costs.
This example underscores the potential of AI in enhancing traditional RPA systems.
Organizations must focus on developing AI solutions that are flexible and can constantly learn from their environment.
AI-Optimized Supply Chain Management
Optimizing supply chain operations is vital for seamless production line functioning.
AI-driven supply chain management tools offer real-time insights and dynamic decision-making capabilities.
A global logistics company employed AI to improve its supply chain operations.
The AI models optimized routes for delivery trucks, reducing fuel consumption and decreasing transit times.
The company reported a 10% decrease in logistics costs within the first year of implementation.
The key lesson here is that AI can uncover inefficiencies in supply chains that might be missed by human analysis.
Emphasizing real-time data collection and analysis will yield substantial improvements in supply chain management.
Lessons Learned from AI Integration
The journey of integrating AI into production lines holds valuable lessons for all industries:
– **Data Quality and Quantity:** Successful AI models rely on high-quality and comprehensive datasets.
Organizations should invest in collecting, cleaning, and organizing data to train robust AI models.
– **Cross-Functional Collaboration:** Implementing AI solutions requires collaborative efforts across various departments such as IT, manufacturing, and quality assurance.
Cross-functional teams should bring diverse expertise to ensure a well-rounded AI strategy.
– **Continuous Learning:** AI models must be regularly updated and refined to adapt to changing production conditions and emerging trends.
Continuous learning is essential for maintaining the effectiveness of AI-driven processes.
– **Scalability and Flexibility:** AI solutions should be scalable to support future growth and flexible to accommodate changes in production needs.
Organizations should plan for incremental AI adoption, allowing systems to evolve over time.
– **Ethical Considerations:** Responsible AI deployment requires ethical considerations to ensure transparency and address concerns such as data privacy and bias in AI models.
Conclusion
AI is undeniably a powerful tool for optimizing production lines, providing a competitive edge to companies that embrace its potential.
The success stories shared here illustrate how AI can transform predictive maintenance, quality control, demand forecasting, robotic process automation, and supply chain management.
By learning from these examples and applying the lessons learned, businesses can embark on their AI optimization journey and achieve remarkable improvements in efficiency and productivity.
資料ダウンロード
QCD調達購買管理クラウド「newji」は、調達購買部門で必要なQCD管理全てを備えた、現場特化型兼クラウド型の今世紀最高の購買管理システムとなります。
ユーザー登録
調達購買業務の効率化だけでなく、システムを導入することで、コスト削減や製品・資材のステータス可視化のほか、属人化していた購買情報の共有化による内部不正防止や統制にも役立ちます。
NEWJI DX
製造業に特化したデジタルトランスフォーメーション(DX)の実現を目指す請負開発型のコンサルティングサービスです。AI、iPaaS、および先端の技術を駆使して、製造プロセスの効率化、業務効率化、チームワーク強化、コスト削減、品質向上を実現します。このサービスは、製造業の課題を深く理解し、それに対する最適なデジタルソリューションを提供することで、企業が持続的な成長とイノベーションを達成できるようサポートします。
オンライン講座
製造業、主に購買・調達部門にお勤めの方々に向けた情報を配信しております。
新任の方やベテランの方、管理職を対象とした幅広いコンテンツをご用意しております。
お問い合わせ
コストダウンが利益に直結する術だと理解していても、なかなか前に進めることができない状況。そんな時は、newjiのコストダウン自動化機能で大きく利益貢献しよう!
(Β版非公開)