- お役立ち記事
- System identification of continuous transfer function model
月間77,185名の
製造業ご担当者様が閲覧しています*
*2025年2月28日現在のGoogle Analyticsのデータより
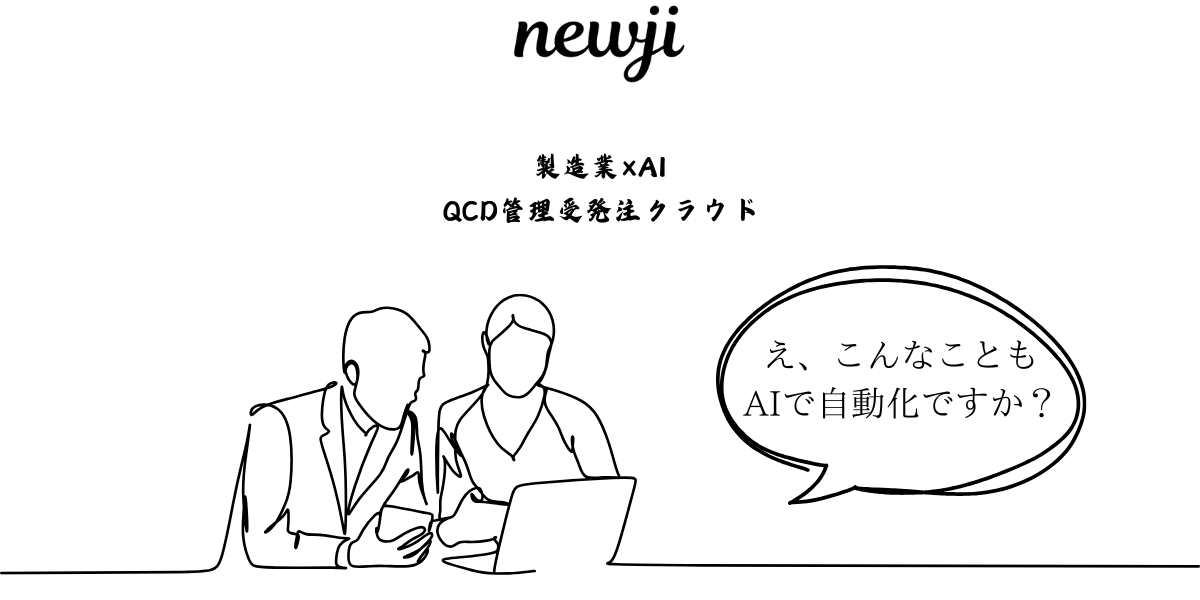
System identification of continuous transfer function model
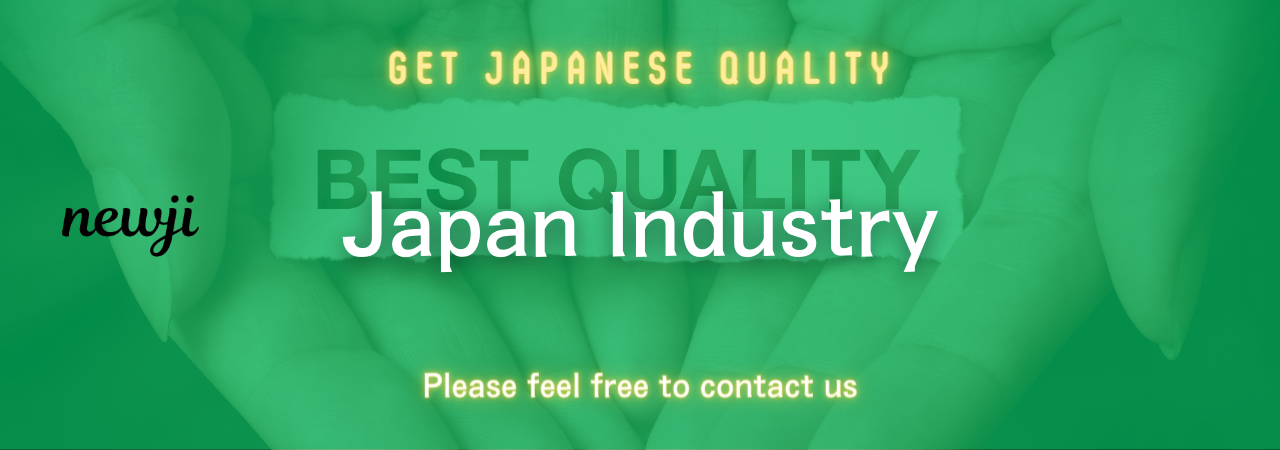
目次
Understanding System Identification
System identification is a fascinating concept that’s like being a detective with mathematical tools.
It helps uncover how systems behave using data and equations.
Imagine you want to understand how a car engine responds to different amounts of fuel.
Instead of physically changing and testing every part, system identification helps predict outcomes through models.
This saves time, resources, and sometimes even prevents catastrophic failures by simulating changes before applying them.
What is a Continuous Transfer Function Model?
When it comes to system identification, the continuous transfer function model plays a central role.
It is a mathematical representation used to describe the input-output relationship of a system.
This model is defined within the s-domain, using Laplace transforms, which consider how systems react over time continuously.
To keep things simple, think of it as a sophisticated way to express if you press the gas pedal by a certain amount, how smoothly and quickly your car accelerates.
The transfer function can help capture all that behavior in mathematical terms, taking into account various forces and responses.
The Basics of Transfer Functions
Transfer functions are expressed as a ratio of output to input in terms of the Laplace variable ‘s’.
This model gives insight into how a system processes input signals to produce an output.
The coefficients within this function are key to defining the system’s characteristics.
For instance, if you think about an RC circuit, the transfer function can help you understand the relationship between the voltage across a resistor and capacitor with the input voltage.
Components of Transfer Functions
The transfer function model is often represented as a fraction, where the numerator and denominator are polynomials in ‘s’.
– **Numerator**: This typically defines the zeros of the system.
It represents conditions where the output is zero regardless of the input.
– **Denominator**: This defines the poles of the system.
Poles are critical as they influence system stability and performance time constants.
Understanding zeros and poles is essential, as they describe the system’s natural behavior and response to external stimuli.
Advantages of Using Continuous Models
Continuous transfer function models provide several advantages when modeling systems:
– **Precision**: They can provide very precise insight into system behavior, incorporating differential equations and continuous dynamics rather than discrete steps.
– **Predictive Capability**: With a robust mathematical framework, these models predict system responses under various conditions with high accuracy, which is invaluable in engineering and scientific research.
– **Simplicity**: For linear systems, transfer functions offer a simpler way of understanding complex systems compared to state-space models.
Real-World Applications
Continuous transfer function models are widely used across various industries.
Engineers and researchers apply these models to design control systems for aircraft, automobiles, industrial robots, and much more.
For example, automotive manufacturers use these models to improve vehicle braking systems.
By understanding the dynamics of the brake components, they can enhance safety and performance without having to test each variation physically.
In the biotech industry, transfer function models help simulate and control bioprocesses, crucial for producing pharmaceuticals, ensuring processes proceed efficiently and safely.
Steps in System Identification
Now that we understand what a transfer function is, let’s dive into the process of system identification.
1. Data Collection
The first step is gathering data.
Data is the cornerstone of system identification, captured from the system by measuring input and output variables.
Accuracy and quantity of data significantly impact the effectiveness of the modeled system.
2. Model Structure Selection
Next, choose an appropriate model structure.
This is where you decide how complex your model should be.
Do you need a simple linear model, or does the system require a more sophisticated nonlinear model?
Choosing too complex a model can lead to overfitting, while too simple a model might not capture essential dynamics.
3. Parameter Estimation
Once you have a chosen model structure, it’s time to estimate its parameters.
This might involve using algorithms such as least squares or more computational techniques like genetic algorithms or neural networks.
These parameters are pivotal in defining how closely the model aligns with real-world behavior.
4. Model Validation
Finally, validate your model.
Does it accurately predict and explain the data outside the sample used for estimation?
This step often involves comparing the modeled responses with real system responses, ensuring reliability.
Challenges and Considerations
While system identification of continuous transfer function models is powerful, it comes with challenges:
– **Noise and Disturbances**: Data is often noisy, which can distort model accuracy.
Sophisticated filtering or pre-processing techniques may be necessary.
– **Nonlinearity**: Many real-world systems exhibit nonlinearities that simple linear models can’t capture.
Identifying and incorporating nonlinearity can complicate modeling.
– **Computational Complexity**: Estimating parameters for large, complex systems can be computationally intensive, requiring significant resources or simplifications.
The Future of System Identification
The future of system identification lies in enhancing methods to handle complex, time-varying, and non-linear systems.
Advancements in machine learning and artificial intelligence are set to revolutionize this field by offering more dynamic and adaptive models.
Moreover, integrating system identification with real-time data streaming from IoT devices can result in more responsive and intelligent systems capable of real-time monitoring and adjustment.
In conclusion, system identification of continuous transfer function models is an indispensable tool in modern engineering and science.
By creating accurate models of real-world systems, we can predict behavior, optimize performance, and ensure safety, all while minimizing physical testing.
As technology evolves, so too will the capabilities of system identification, opening new avenues for innovation and efficiency.
資料ダウンロード
QCD管理受発注クラウド「newji」は、受発注部門で必要なQCD管理全てを備えた、現場特化型兼クラウド型の今世紀最高の受発注管理システムとなります。
ユーザー登録
受発注業務の効率化だけでなく、システムを導入することで、コスト削減や製品・資材のステータス可視化のほか、属人化していた受発注情報の共有化による内部不正防止や統制にも役立ちます。
NEWJI DX
製造業に特化したデジタルトランスフォーメーション(DX)の実現を目指す請負開発型のコンサルティングサービスです。AI、iPaaS、および先端の技術を駆使して、製造プロセスの効率化、業務効率化、チームワーク強化、コスト削減、品質向上を実現します。このサービスは、製造業の課題を深く理解し、それに対する最適なデジタルソリューションを提供することで、企業が持続的な成長とイノベーションを達成できるようサポートします。
製造業ニュース解説
製造業、主に購買・調達部門にお勤めの方々に向けた情報を配信しております。
新任の方やベテランの方、管理職を対象とした幅広いコンテンツをご用意しております。
お問い合わせ
コストダウンが利益に直結する術だと理解していても、なかなか前に進めることができない状況。そんな時は、newjiのコストダウン自動化機能で大きく利益貢献しよう!
(β版非公開)