- お役立ち記事
- System identification of continuous transfer function model
月間77,185名の
製造業ご担当者様が閲覧しています*
*2025年2月28日現在のGoogle Analyticsのデータより
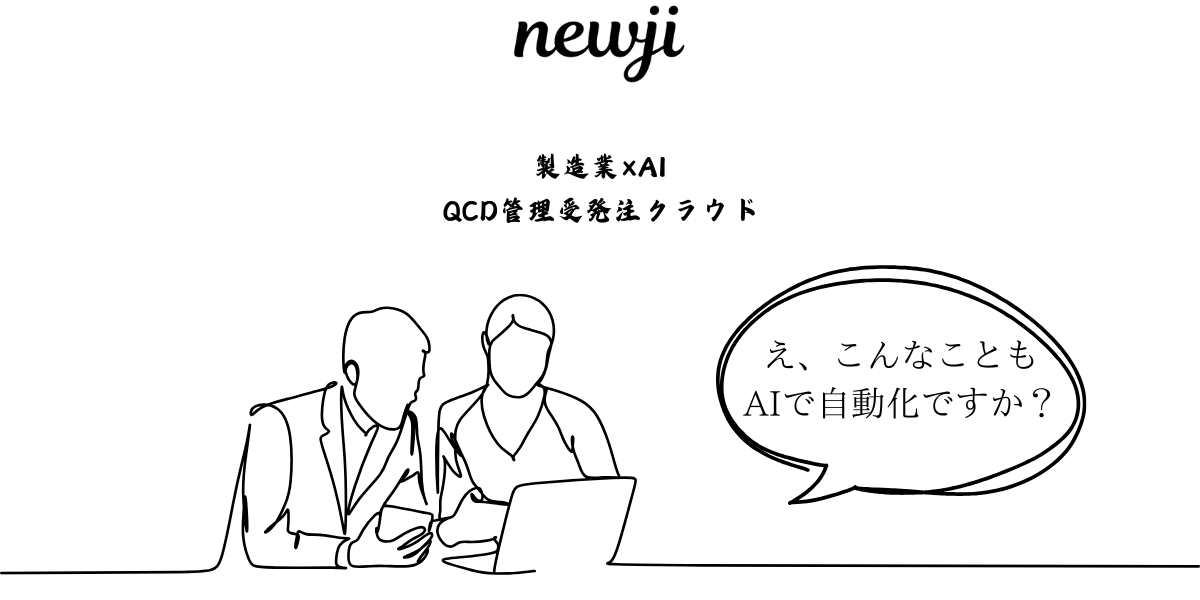
System identification of continuous transfer function model
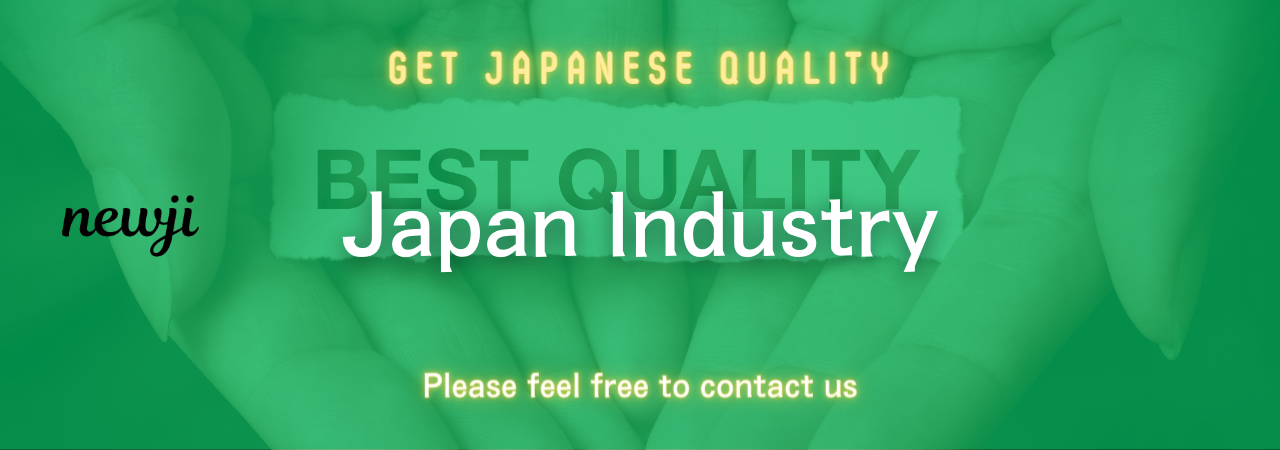
目次
Understanding System Identification
System identification is a fascinating field that involves developing mathematical models to describe how a physical system behaves based on observed data.
It involves collecting data from a system, whether it be mechanical, electrical, or even biological, and then using that data to create a model that can predict future behavior or understand the internal mechanisms.
At its core, system identification relies on determining the relationships between inputs and outputs.
This process utilizes various mathematical techniques and computational algorithms to generate models that can represent the dynamics of the system accurately.
The Importance of Transfer Functions
In the context of system identification, a transfer function is a powerful tool used to model linear time-invariant (LTI) systems.
Essentially, a transfer function represents the input-output relationship of a system in the Laplace domain.
It is expressed as a ratio of polynomials, where the numerator and denominator represent the system’s behavior.
Transfer functions are vital because they simplify the analysis and design of control systems.
By transforming differential equations into algebraic equations, they allow engineers and researchers to work with complex systems more efficiently.
This simplification is crucial when dealing with real-world systems that require precise modeling for applications such as robotics, automotive control, and audio signal processing.
Steps in System Identification
The process of system identification involves several critical steps.
Each step plays a role in ensuring the accuracy and reliability of the developed model.
Data Collection
The first step in system identification is collecting accurate and relevant data from the system of interest.
This data typically includes both input signals, which are the external influences acting on the system, and output signals, which are the system’s response.
The quality and quantity of this data will significantly influence the success of the model.
It’s important to use sensors and measurement devices that can accurately capture the input and output signals.
For example, in an electrical system, this might involve using voltage and current sensors, while in a mechanical system, it could involve displacement or velocity sensors.
Data Preprocessing
Once the data is collected, it often requires preprocessing to make it suitable for analysis.
This step may involve filtering noise, normalizing values, or dealing with missing data points.
Effective preprocessing ensures that the data accurately reflects the system’s true behavior without distortions.
During preprocessing, engineers must consider various noise reduction techniques, such as low-pass filtering, to remove unwanted fluctuations.
Additionally, normalizing the data can prevent scale-related issues during the subsequent modeling phase.
Model Structure Selection
After preprocessing, the next step is to choose an appropriate model structure.
This involves deciding on the complexity and form of the model that will represent the system.
Common model structures include ARX (AutoRegressive with eXogenous inputs) models, state-space models, and transfer functions.
The choice of model structure depends on several factors, such as the system’s dynamics and the nature of the data.
Transfer functions, for instance, are particularly useful for linear systems where the behavior can be captured through a ratio of polynomials.
Parameter Estimation
With the model structure determined, the next step is parameter estimation.
This process involves finding the values of the parameters within the chosen model structure that best fit the observed data.
Numerous algorithms, like least squares or maximum likelihood estimation, are used for this purpose.
The goal is to minimize the difference between the model’s predicted output and the actual observed output.
By fine-tuning the parameters, the model can closely replicate the system’s dynamics and improve its predictive power.
Model Validation
Once the model parameters are estimated, it’s essential to validate the model to ensure its accuracy and reliability.
Model validation involves comparing the model’s predictions with a separate set of data that was not used during the estimation process.
This helps determine how well the model generalizes to new situations.
Some common techniques for model validation include calculating the model’s fit percentage or using cross-validation methods.
If the model’s performance is satisfactory, it can be used for further analysis or system design.
Applications of System Identification
System identification has a wide range of applications across various industries.
Selected examples illustrate its practical value in enhancing system performance and developing efficient control strategies.
Engineering and Control Systems
In engineering, system identification is fundamental for designing control systems that manage processes in sectors like manufacturing, aerospace, and automotive industries.
By understanding the system dynamics through modeling, engineers can create controllers that improve stability, efficiency, and responsiveness.
For instance, in the automotive industry, system identification plays a crucial role in developing advanced driver-assistance systems (ADAS).
These systems rely on models to interpret sensor data and make real-time decisions to enhance vehicle safety and performance.
Medical and Biological Systems
System identification is also vital in the medical field, where it aids in the modeling of physiological processes.
Accurate models can help in understanding complex interactions within the human body, leading to better diagnoses and treatments.
Biomedical engineers use system identification to create models of glucose-insulin interactions in diabetic patients.
Such models inform the development of insulin delivery systems and improve the management of blood sugar levels.
Conclusion
The system identification of continuous transfer function models is a powerful tool that enables engineers and researchers to understand and predict the behavior of complex systems.
By following a structured process involving data collection, preprocessing, model selection, parameter estimation, and validation, it’s possible to create models that enhance our ability to control and optimize systems across various domains.
System identification serves as a bridge between theoretical analysis and practical application, making it indispensable in modern engineering and scientific research.
As technology advances and data becomes increasingly available, the significance of system identification continues to grow, offering new opportunities to innovate and improve existing systems.
資料ダウンロード
QCD管理受発注クラウド「newji」は、受発注部門で必要なQCD管理全てを備えた、現場特化型兼クラウド型の今世紀最高の受発注管理システムとなります。
ユーザー登録
受発注業務の効率化だけでなく、システムを導入することで、コスト削減や製品・資材のステータス可視化のほか、属人化していた受発注情報の共有化による内部不正防止や統制にも役立ちます。
NEWJI DX
製造業に特化したデジタルトランスフォーメーション(DX)の実現を目指す請負開発型のコンサルティングサービスです。AI、iPaaS、および先端の技術を駆使して、製造プロセスの効率化、業務効率化、チームワーク強化、コスト削減、品質向上を実現します。このサービスは、製造業の課題を深く理解し、それに対する最適なデジタルソリューションを提供することで、企業が持続的な成長とイノベーションを達成できるようサポートします。
製造業ニュース解説
製造業、主に購買・調達部門にお勤めの方々に向けた情報を配信しております。
新任の方やベテランの方、管理職を対象とした幅広いコンテンツをご用意しております。
お問い合わせ
コストダウンが利益に直結する術だと理解していても、なかなか前に進めることができない状況。そんな時は、newjiのコストダウン自動化機能で大きく利益貢献しよう!
(β版非公開)