- お役立ち記事
- Technologies and application examples using deep learning
月間76,176名の
製造業ご担当者様が閲覧しています*
*2025年3月31日現在のGoogle Analyticsのデータより
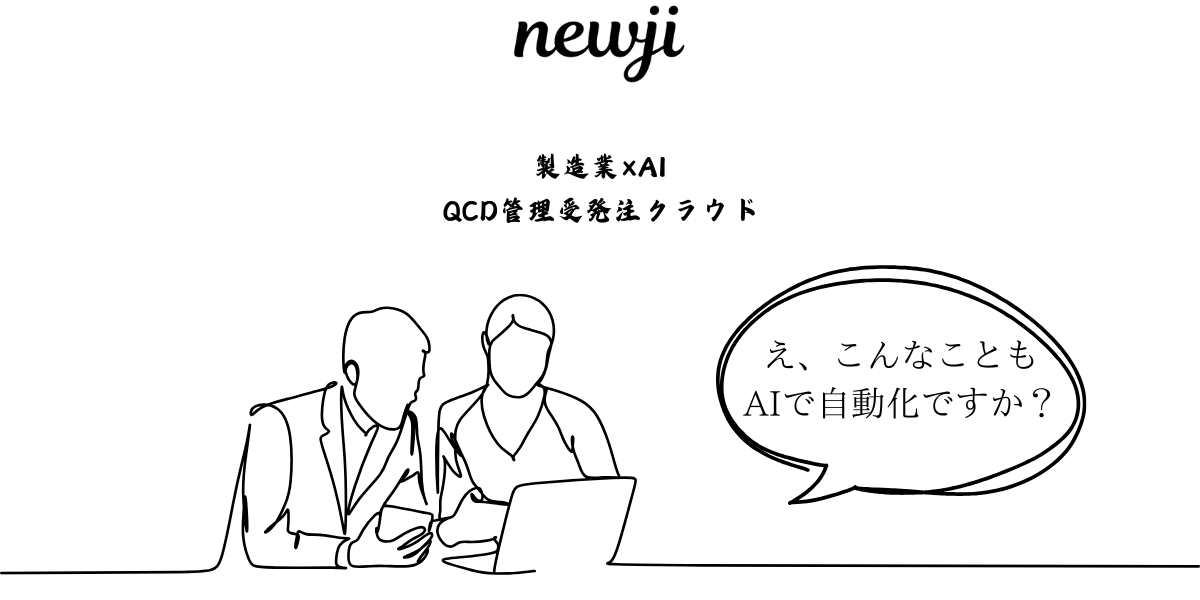
Technologies and application examples using deep learning
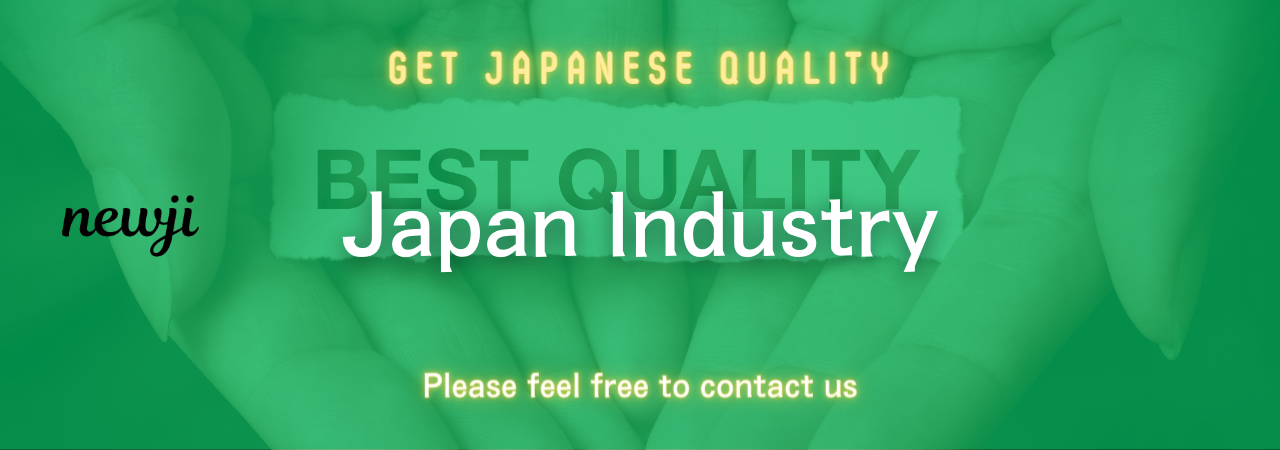
目次
What is Deep Learning?
Deep learning is a subset of artificial intelligence (AI) and machine learning (ML) that simulates the workings of the human brain in processing data and creating patterns for use in decision making.
It involves neural networks with three or more layers, known as artificial neural networks, which strive to mimic the logic of how a human brain operates.
These networks are designed to analyze vast amounts of data efficiently and make predictions with high accuracy.
The Importance of Deep Learning
Deep learning has transformed many industries by providing insights and accuracy that were not possible with traditional machine learning algorithms.
Because of its ability to manage a large volume of unstructured data, deep learning is pivotal in fields like healthcare, finance, automotive, and technology.
Its application in image and speech recognition, for example, has revolutionized how we approach tasks ranging from diagnoses to personal virtual assistants.
Technologies Behind Deep Learning
Neural Networks
The backbone of deep learning is the artificial neural network.
Neural networks consist of layers of nodes, made up of an input layer, hidden layers, and an output layer.
Each node or neuron in the network has an associated weight and threshold.
If the output of an individual node exceeds a given threshold value, the node is activated, sending data to the next layer of the network.
Convolutional Neural Networks (CNNs)
CNNs are tailored for processing structured grid data like images.
They are used to detect and recognize faces, individuals, street signs, and many other aspects of visual data.
The convolutional layer is essential as it helps in effectively detecting patterns and features with less computational power.
Recurrent Neural Networks (RNNs)
RNNs are specialized neural networks designed for sequence-based data such as time series and natural language.
They possess internal memory, which helps them remember previous inputs, making them effective for tasks like language modeling, translation, and predictive typing.
Generative Adversarial Networks (GANs)
GANs consist of two neural networks, pitted one against the other: a generator and a discriminator.
The generator creates new data instances, while the discriminator evaluates them for authenticity.
They are widely used in creating realistic images, videos, and audio, finding applications in fields like art, gaming, and virtual reality.
Applications of Deep Learning
Image and Voice Recognition
Deep learning has immensely enhanced image and voice recognition, paving the way for various innovations such as facial recognition systems and virtual assistants like Siri and Alexa.
It is also utilized in diagnosing medical conditions from imaging data, offering more reliable and faster results than human analysis.
Autonomous Vehicles
Deep learning drives advancements in the automotive sector, especially in developing self-driving cars.
It helps these vehicles process information from their sensors to understand and navigate their environment safely, complying with traffic rules and recognizing objects in their path.
Financial Services
In the finance industry, deep learning is used for fraud detection, risk management, and customer service automation.
Its ability to analyze complex datasets in real-time aids financial institutions in offering personalized services and detecting fraudulent activities quickly.
Healthcare
Deep learning is making significant contributions to the healthcare industry by analyzing patient data and medical images to predict diseases and recommend treatments.
Its use in genomics research has also been pivotal in understanding genetic conditions and developing personalized medicine.
The Future of Deep Learning
The future of deep learning is promising, with endless opportunities for growth and impact across all sectors.
The ongoing research in AI aims at creating more autonomous systems that can learn and evolve beyond human intervention.
With advancements in computation capabilities and data storage, deep learning technologies are expected to become more sophisticated, providing even more accurate and autonomous solutions.
More than just a technological trend, deep learning is positioning itself as a cornerstone of modern AI development.
Its potential to innovate, when combined with ethical considerations and robust frameworks, could lead to a future where applications of deep learning enrich human lives in safer, smarter, and more efficient ways.
資料ダウンロード
QCD管理受発注クラウド「newji」は、受発注部門で必要なQCD管理全てを備えた、現場特化型兼クラウド型の今世紀最高の受発注管理システムとなります。
ユーザー登録
受発注業務の効率化だけでなく、システムを導入することで、コスト削減や製品・資材のステータス可視化のほか、属人化していた受発注情報の共有化による内部不正防止や統制にも役立ちます。
NEWJI DX
製造業に特化したデジタルトランスフォーメーション(DX)の実現を目指す請負開発型のコンサルティングサービスです。AI、iPaaS、および先端の技術を駆使して、製造プロセスの効率化、業務効率化、チームワーク強化、コスト削減、品質向上を実現します。このサービスは、製造業の課題を深く理解し、それに対する最適なデジタルソリューションを提供することで、企業が持続的な成長とイノベーションを達成できるようサポートします。
製造業ニュース解説
製造業、主に購買・調達部門にお勤めの方々に向けた情報を配信しております。
新任の方やベテランの方、管理職を対象とした幅広いコンテンツをご用意しております。
お問い合わせ
コストダウンが利益に直結する術だと理解していても、なかなか前に進めることができない状況。そんな時は、newjiのコストダウン自動化機能で大きく利益貢献しよう!
(β版非公開)