- お役立ち記事
- The basics of Weibull analysis, its practical use for reliability improvement measures, and its key points
月間76,176名の
製造業ご担当者様が閲覧しています*
*2025年3月31日現在のGoogle Analyticsのデータより
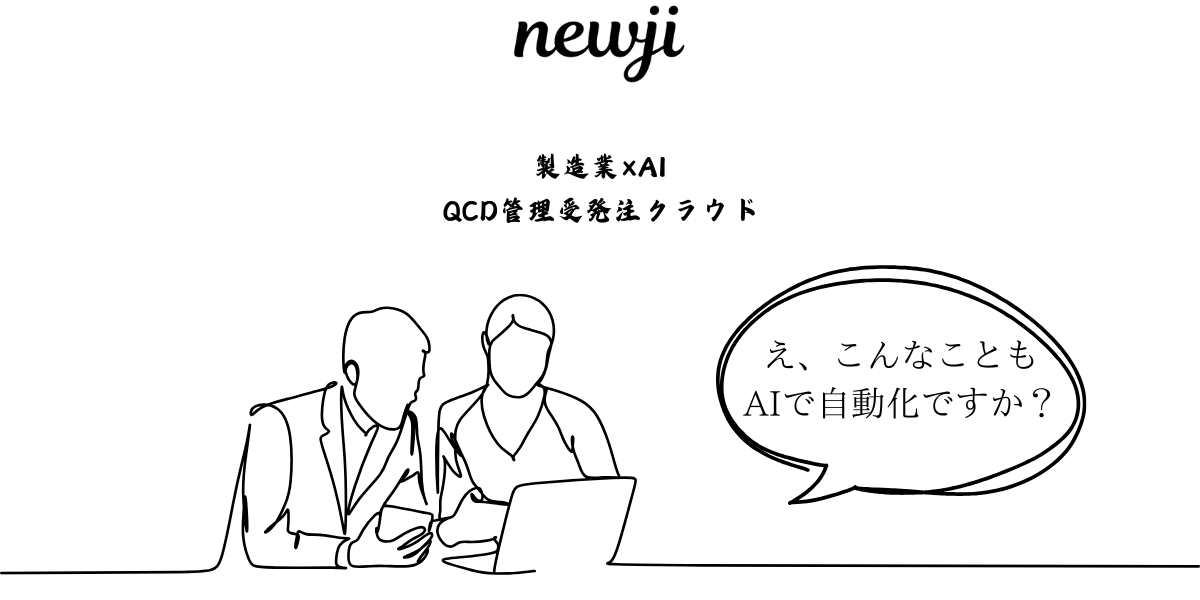
The basics of Weibull analysis, its practical use for reliability improvement measures, and its key points
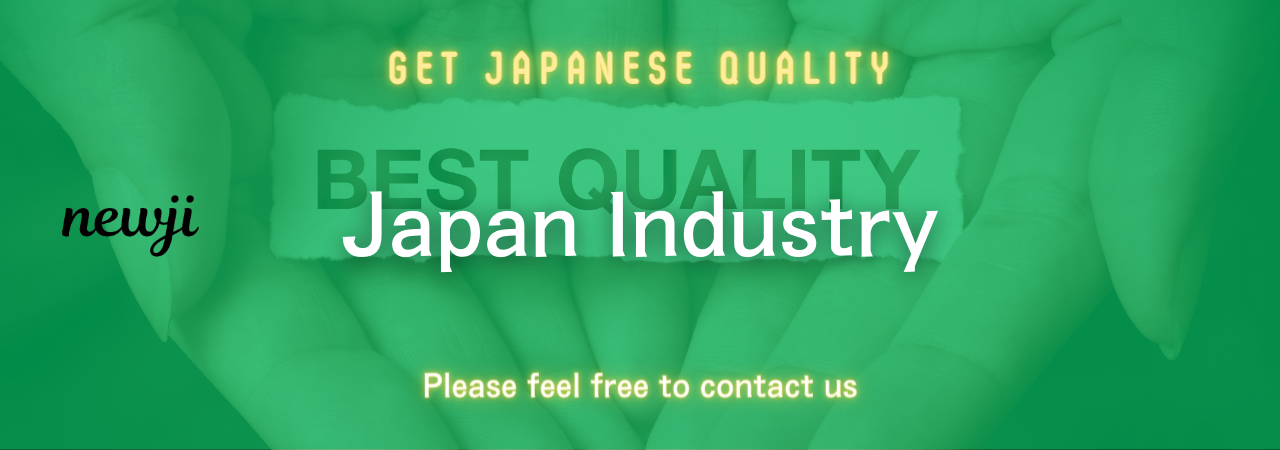
目次
Understanding Weibull Analysis
Weibull analysis is a statistical method used to analyze and predict the failure rates of products or systems.
This analysis is popular in reliability engineering for determining a product’s lifecycle — from early failures to wear-out failures.
It is named after Wallodi Weibull, who introduced the Weibull distribution in 1951.
The Weibull distribution helps in modeling various types of failure rates, providing insight into how long products are likely to last and what factors could lead to early failures.
This understanding is crucial for improving product reliability and reducing potential risks in production.
Basic Concepts of Weibull Distribution
At the heart of Weibull analysis lies the Weibull distribution, a versatile probability distribution used to model diverse data types.
There are three main parameters in the Weibull distribution:
1. **Shape Parameter (β)**: It determines the type of failure rate behavior. When β < 1, it indicates a decreasing failure rate, often seen in early-life failures. When β equals 1, it suggests a constant failure rate, associated with random failures. A β > 1 indicates an increasing failure rate, implying wear-out failures.
2. **Scale Parameter (η)**: Often referred to as the characteristic life, it signifies the time by which 63.2% of items are expected to fail. It helps identify the lifespan or durability of the product under analysis.
3. **Location Parameter (γ)**: This is optional in some cases and shifts the entire distribution along the time axis. It represents a minimum life or delayed failure time.
These parameters are crucial for crafting predictions and decisions based on the fail rates across different time periods.
Practical Use of Weibull Analysis in Reliability Engineering
Weibull analysis serves multiple practical applications in industries aiming to enhance product reliability and improve operational processes.
Here’s how Weibull analysis can be effectively applied:
Failure Analysis and Prediction
By modeling the failure data, Weibull analysis helps in predicting future failures.
This prediction provides invaluable insight, allowing manufacturers to make data-driven decisions regarding warranty periods and product designs.
Understanding failure patterns assists engineers in identifying potential weaknesses in products, aiding in the creation of better and more reliable designs.
Warranty Analysis
Weibull analysis enables companies to structure warranties based on estimated product life cycles.
By understanding the failure distribution, companies can set realistic warranty periods that minimize their financial risk while optimizing customer satisfaction.
This also aids in reducing the costs associated with returns and replacements.
Maintenance Planning and Optimization
In maintenance strategies, Weibull analysis plays a critical role in predicting when a system is likely to require repairs.
This helps organizations schedule proactive maintenance before failure occurs, thereby avoiding costly downtime and extending the useful life of equipment.
Effective maintenance planning reduces operational interruptions and enhances safety.
Quality Control and Testing
During product development, Weibull analysis aids in determining the necessary sample size and testing duration required to ensure product reliability.
This ensures an accelerated and accurate assessment without incurring excessive testing costs.
The analysis helps in identifying protentional design errors early on, enabling corrective measures to be taken before market release.
Key Points for Effective Weibull Analysis
To conduct a successful Weibull analysis, there are key considerations and practices that should be kept in mind:
Data Collection and Preparation
Accurate data is the backbone of any statistical analysis.
Ensure that failure data is collected systematically and includes all relevant operational details.
Inaccurate or incomplete data can skew results and lead to incorrect conclusions.
Furthermore, understanding the operational conditions under which the data has been collected is vital for interpreting results appropriately.
Choosing the Right Weibull Model
Deciding on the correct Weibull model involves selecting the appropriate form (two-parameter or three-parameter model).
This choice impacts how failure data is analyzed and interpreted.
A simple approach is often preferred for typical applications, like selecting the two-parameter model unless a specific reason calls for a more complex model.
Analyzing and Interpreting Results
After applying the Weibull model, focus on interpreting the results in the context of your specific needs.
Identify which part of the lifecycle (infancy, normal life, or wear-out) the majority of failures occur in.
This insight is crucial for strategizing effective reliability improvements.
Consultation with experienced statisticians or reliability engineers can provide added value in ensuring results are accurately interpreted.
Incorporation into Reliability Improvement Measures
Weibull analysis does not end with mere prediction; it should inform actionable improvements.
Results should guide modifications in design, testing, manufacturing processes, or use conditions to mitigate identified risks.
Engage cross-functional teams, including design, quality, and production, to implement data-driven strategies and enhance product reliability.
Conclusion
Weibull analysis serves as an essential tool in understanding and improving the reliability of systems and components.
By accurately predicting failure behaviors, this analysis assists in making informed decisions in product design and lifecycle management.
Employing Weibull analysis effectively requires attention to detail in data collection, model selection, and result interpretation.
Incorporating insights from this analysis into practical processes leads to enhanced product quality and customer satisfaction while reducing operational costs and risks.
Reliability engineering benefits significantly from precise, data-driven approaches such as Weibull analysis, making it a cornerstone of effective risk management and product improvement strategies.
資料ダウンロード
QCD管理受発注クラウド「newji」は、受発注部門で必要なQCD管理全てを備えた、現場特化型兼クラウド型の今世紀最高の受発注管理システムとなります。
ユーザー登録
受発注業務の効率化だけでなく、システムを導入することで、コスト削減や製品・資材のステータス可視化のほか、属人化していた受発注情報の共有化による内部不正防止や統制にも役立ちます。
NEWJI DX
製造業に特化したデジタルトランスフォーメーション(DX)の実現を目指す請負開発型のコンサルティングサービスです。AI、iPaaS、および先端の技術を駆使して、製造プロセスの効率化、業務効率化、チームワーク強化、コスト削減、品質向上を実現します。このサービスは、製造業の課題を深く理解し、それに対する最適なデジタルソリューションを提供することで、企業が持続的な成長とイノベーションを達成できるようサポートします。
製造業ニュース解説
製造業、主に購買・調達部門にお勤めの方々に向けた情報を配信しております。
新任の方やベテランの方、管理職を対象とした幅広いコンテンツをご用意しております。
お問い合わせ
コストダウンが利益に直結する術だと理解していても、なかなか前に進めることができない状況。そんな時は、newjiのコストダウン自動化機能で大きく利益貢献しよう!
(β版非公開)