- お役立ち記事
- The difference between Deep Learning and Reinforcement Learning
The difference between Deep Learning and Reinforcement Learning
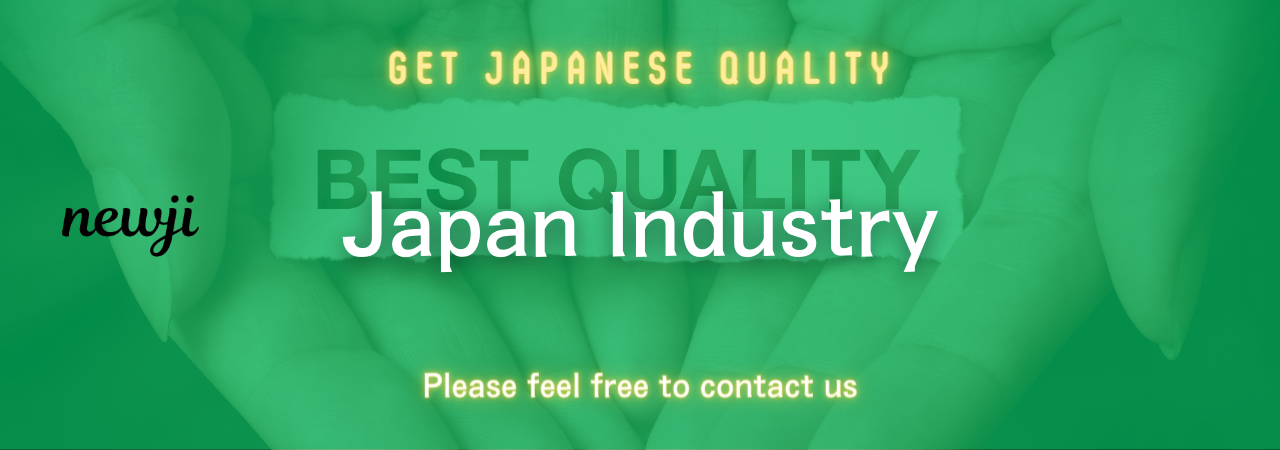
In the world of artificial intelligence, many terms and techniques can be quite confusing.
Two key concepts often discussed are deep learning and reinforcement learning.
Although they might sound similar and are connected to AI, they serve different purposes and function in unique ways.
In this article, we will dive into the differences between deep learning and reinforcement learning to help you understand these fascinating fields.
目次
What is Deep Learning?
Deep learning is a subset of machine learning, which in turn is a subset of artificial intelligence.
In deep learning, computers are taught to recognize patterns in data through neural networks.
These neural networks are designed to mimic how the human brain works.
They are composed of layers of interconnected “neurons” that each process a small part of the information.
How Does Deep Learning Work?
Deep learning networks are made up of multiple layers.
When data is fed into the network, each layer processes the data and extracts features.
The more layers there are, the more complex patterns the network can recognize.
This process enables deep learning systems to perform tasks like image recognition, speech recognition, and natural language processing with impressive accuracy.
Common Uses of Deep Learning
Deep learning is used in various applications across different industries.
For instance, in the healthcare industry, deep learning algorithms can analyze medical images to detect diseases.
In the automotive industry, deep learning is a key component in developing self-driving cars.
Social media platforms also utilize deep learning to filter content and recommend posts to users.
What is Reinforcement Learning?
Reinforcement learning, on the other hand, is a type of machine learning that is all about making decisions.
It involves training algorithms to make a sequence of decisions by rewarding them for correct actions and penalizing them for incorrect ones.
Reinforcement learning is inspired by how animals learn from their environment through trial and error.
How Does Reinforcement Learning Work?
In reinforcement learning, an agent interacts with its environment by performing actions.
Each action leads to a new state and a reward (or penalty).
The agent’s goal is to maximize its cumulative reward over time.
To do this, it must learn which actions yield the highest rewards.
One popular method within reinforcement learning is Q-learning, where the agent learns a value function that helps predict the usefulness of actions in various states.
Common Uses of Reinforcement Learning
Reinforcement learning is widely used in fields where decision-making is critical.
For example, in robotics, reinforcement learning can help robots learn how to navigate and manipulate objects.
In finance, it can be used for automated trading strategies.
Another exciting area is gaming, where reinforcement learning algorithms have been used to develop AI that can play games like Go, chess, and even complex video games at superhuman levels.
Key Differences Between Deep Learning and Reinforcement Learning
Though deep learning and reinforcement learning are both part of machine learning, there are several differences between them.
Purpose and Goals
The primary purpose of deep learning is to recognize patterns and make predictions based on data.
It excels at tasks involving large amounts of structured data, such as images or text.
In contrast, reinforcement learning focuses on making decisions and optimizing actions over time.
It is best suited for environments where an agent must learn from the consequences of its actions to achieve a goal.
Learning Process
In deep learning, the learning process involves training neural networks on a dataset by adjusting weights through a process called backpropagation.
The network learns to minimize errors through multiple iterations.
Reinforcement learning, on the other hand, is more dynamic.
The agent learns through a cycle of action and feedback.
It continuously interacts with the environment, receives rewards or penalties, and updates its strategy accordingly.
Types of Problems Addressed
Deep learning is particularly effective for tasks that require processing and understanding vast amounts of data.
Examples include image classification, language translation, and voice recognition.
Reinforcement learning, however, is optimal for problems that require sequential decision-making.
Such tasks include controlling a robot, playing a game, or managing resources in an uncertain environment.
Working Together
In some cases, deep learning and reinforcement learning can be combined to create powerful AI systems.
For instance, deep learning can be used to process raw sensory data into useful features, which can then be fed into a reinforcement learning algorithm to make decisions.
This combination has been used in advanced applications like autonomous driving and complex strategy games.
Conclusion
Understanding the difference between deep learning and reinforcement learning is important for anyone interested in artificial intelligence.
While both are key components of AI, they serve different purposes and are suited to different types of problems.
Deep learning excels in pattern recognition and prediction, while reinforcement learning shines in decision-making and strategy.
By leveraging the strengths of both, we can continue to make incredible advancements in technology and AI.
By clarifying these concepts, we hope to have provided a clearer picture of how AI agents learn and perform their tasks.
Whether it’s recognizing images or making decisions, each approach has its unique advantages and applications.
So, the next time you come across these terms, you’ll know exactly what they mean and how they differ.
資料ダウンロード
QCD調達購買管理クラウド「newji」は、調達購買部門で必要なQCD管理全てを備えた、現場特化型兼クラウド型の今世紀最高の購買管理システムとなります。
ユーザー登録
調達購買業務の効率化だけでなく、システムを導入することで、コスト削減や製品・資材のステータス可視化のほか、属人化していた購買情報の共有化による内部不正防止や統制にも役立ちます。
NEWJI DX
製造業に特化したデジタルトランスフォーメーション(DX)の実現を目指す請負開発型のコンサルティングサービスです。AI、iPaaS、および先端の技術を駆使して、製造プロセスの効率化、業務効率化、チームワーク強化、コスト削減、品質向上を実現します。このサービスは、製造業の課題を深く理解し、それに対する最適なデジタルソリューションを提供することで、企業が持続的な成長とイノベーションを達成できるようサポートします。
オンライン講座
製造業、主に購買・調達部門にお勤めの方々に向けた情報を配信しております。
新任の方やベテランの方、管理職を対象とした幅広いコンテンツをご用意しております。
お問い合わせ
コストダウンが利益に直結する術だと理解していても、なかなか前に進めることができない状況。そんな時は、newjiのコストダウン自動化機能で大きく利益貢献しよう!
(Β版非公開)