- お役立ち記事
- The latest deep learning technology based on TDA and tensor decomposition and its application to time series data graph data analysis
月間76,176名の
製造業ご担当者様が閲覧しています*
*2025年3月31日現在のGoogle Analyticsのデータより
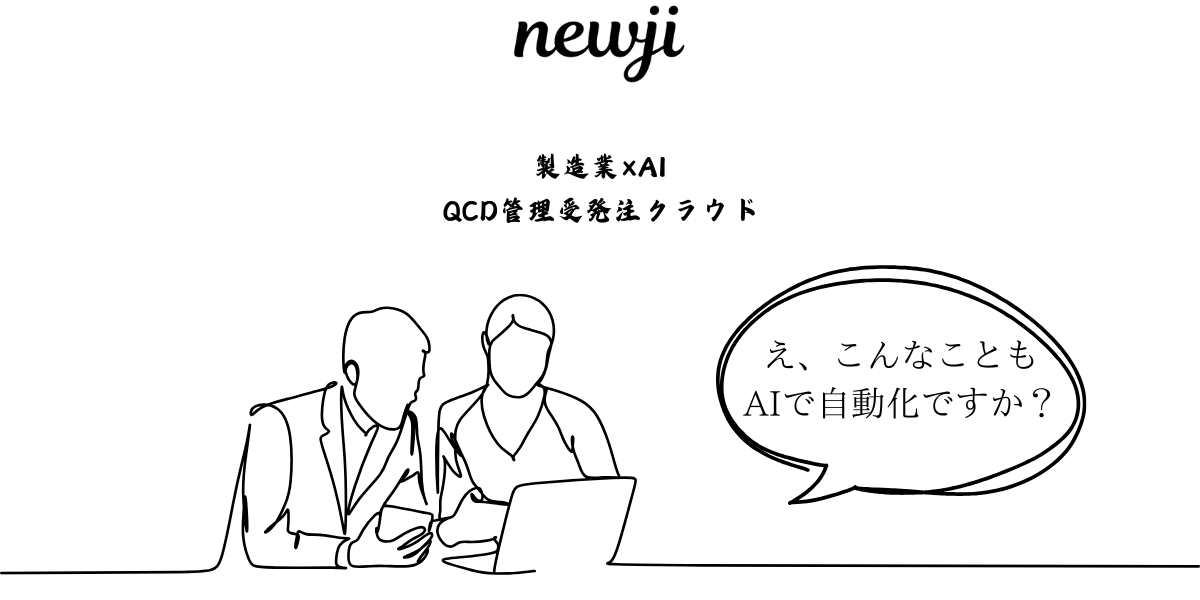
The latest deep learning technology based on TDA and tensor decomposition and its application to time series data graph data analysis
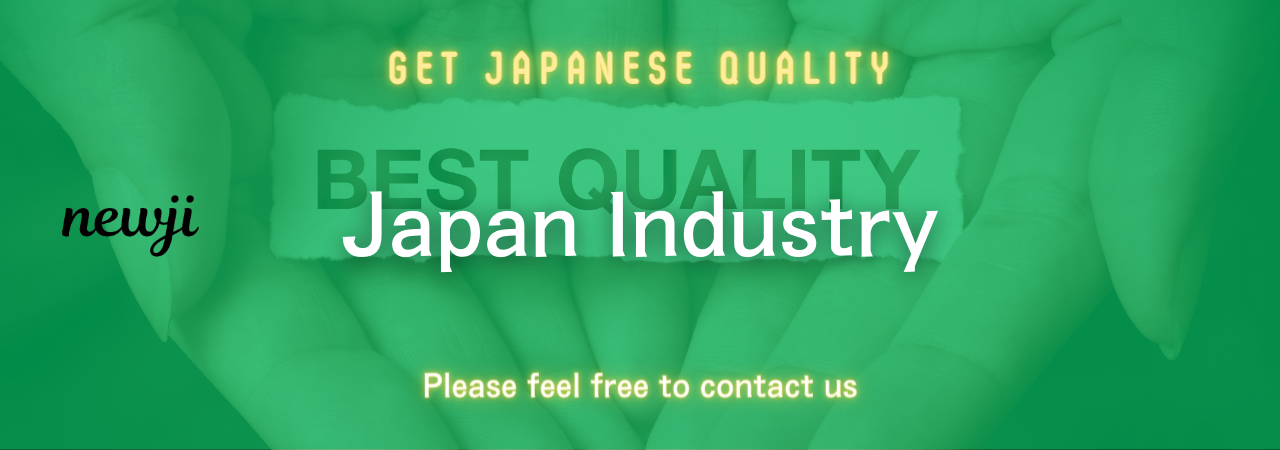
目次
Understanding Deep Learning
Deep learning is a subset of artificial intelligence (AI) that focuses on training computers to learn from data in a manner that’s similar to how humans learn.
It involves the use of neural networks, which are computer systems modeled after the human brain.
These networks are characterized by layers of interconnected nodes, with each layer learning progressively more complex features from the input data.
The latest advancements in deep learning technologies have opened up new avenues for data analysis, particularly in the areas of time series and graph data analysis.
Topological Data Analysis (TDA)
One of the groundbreaking techniques integrated into modern deep learning is Topological Data Analysis (TDA).
TDA is a method used for understanding the shape of complex data.
Unlike traditional data analysis that focuses on numeric summaries, TDA examines the underlying shape, connectivity, and topology of data.
This approach helps in identifying clusters, holes, and voids within data, which can reveal significant insights that might be missed by conventional analytics.
In the context of deep learning, TDA contributes by providing a new perspective on how data can be represented and analyzed.
By capturing the topological features, TDA allows for a richer, more nuanced understanding of data structures, which can subsequently enhance the learning process of neural networks.
The Role of Tensor Decomposition
Tensor decomposition is another pivotal advancement in deep learning.
A tensor is essentially a multi-dimensional array of data that generalizes matrices to higher dimensions.
Tensor decomposition refers to techniques used to break down these large, complex tensors into simpler, more manageable parts.
This process is crucial for reducing the computational load and improving the efficiency of deep learning models.
In practice, tensor decomposition enables the extraction of latent patterns and structures from high-dimensional data.
Such capabilities are especially valuable when dealing with massive datasets typical in modern applications, as it facilitates efficient data compression and accelerates the learning process.
Integrating TDA and Tensor Decomposition in Deep Learning
The integration of TDA and tensor decomposition within deep learning frameworks has proven to be highly effective for advanced data analysis.
Together, these techniques enhance the model’s ability to learn from complex data by providing both topological and decomposed representations, which lead to improved accuracy and insight extraction.
When applied to time series data, which involves sequences of data points indexed in time order, this combined approach can significantly improve forecasting accuracy.
Time series data often harbor patterns that are difficult to detect using traditional methods, but with TDA and tensor decomposition, these patterns become more apparent, allowing for better predictions and more informed decision-making.
In graph data analysis, where data points are represented as nodes and edges, deep learning models enhanced with TDA and tensor decomposition can more effectively identify relationships and patterns within a network.
This capability is essential in fields like social network analysis, bioinformatics, and logistics, where understanding the complexities of connections and data flows can lead to breakthroughs in efficiency and innovation.
Applications in Real-World Scenarios
The application of the latest deep learning technologies augmented with TDA and tensor decomposition has seen tangible results across various domains.
In healthcare, for example, this approach can improve diagnostic accuracy by analyzing complex medical datasets with greater precision and speed.
In finance, it enhances the ability to predict market trends and optimize trading strategies by effectively processing enormous volumes of fluctuating time series data.
Another area of application is in autonomous systems, such as self-driving vehicles, which require real-time data analysis to make split-second decisions.
These systems benefit from the quick and accurate processing capabilities offered by deep learning models utilizing TDA and tensor decomposition.
They can better interpret sensor data, recognize patterns, and make informed navigation choices.
Challenges and Future Prospects
While the integration of TDA and tensor decomposition in deep learning represents significant progress, there are still challenges to address.
The computational cost and complexity of these techniques can be high, necessitating further research into optimization and scalability to make them more accessible and practical for broader use.
However, the potential of these technologies is vast, and as computational resources continue to advance, the barriers will likely diminish.
Future research will focus on refining these methodologies, making them more efficient, and expanding their applicability to new types of data and emerging fields.
Conclusion
The latest deep learning technologies leveraging TDA and tensor decomposition mark a new era in data analysis.
Their ability to transform big data into actionable insights holds promise for numerous applications across various industries.
As these techniques continue to evolve, they will offer deeper, more nuanced insights into complex datasets, driving innovation and discovery in ways we are just beginning to comprehend.
資料ダウンロード
QCD管理受発注クラウド「newji」は、受発注部門で必要なQCD管理全てを備えた、現場特化型兼クラウド型の今世紀最高の受発注管理システムとなります。
ユーザー登録
受発注業務の効率化だけでなく、システムを導入することで、コスト削減や製品・資材のステータス可視化のほか、属人化していた受発注情報の共有化による内部不正防止や統制にも役立ちます。
NEWJI DX
製造業に特化したデジタルトランスフォーメーション(DX)の実現を目指す請負開発型のコンサルティングサービスです。AI、iPaaS、および先端の技術を駆使して、製造プロセスの効率化、業務効率化、チームワーク強化、コスト削減、品質向上を実現します。このサービスは、製造業の課題を深く理解し、それに対する最適なデジタルソリューションを提供することで、企業が持続的な成長とイノベーションを達成できるようサポートします。
製造業ニュース解説
製造業、主に購買・調達部門にお勤めの方々に向けた情報を配信しております。
新任の方やベテランの方、管理職を対象とした幅広いコンテンツをご用意しております。
お問い合わせ
コストダウンが利益に直結する術だと理解していても、なかなか前に進めることができない状況。そんな時は、newjiのコストダウン自動化機能で大きく利益貢献しよう!
(β版非公開)