- お役立ち記事
- Utilization of machine learning for predictive maintenance and product inspection and its key points
Utilization of machine learning for predictive maintenance and product inspection and its key points
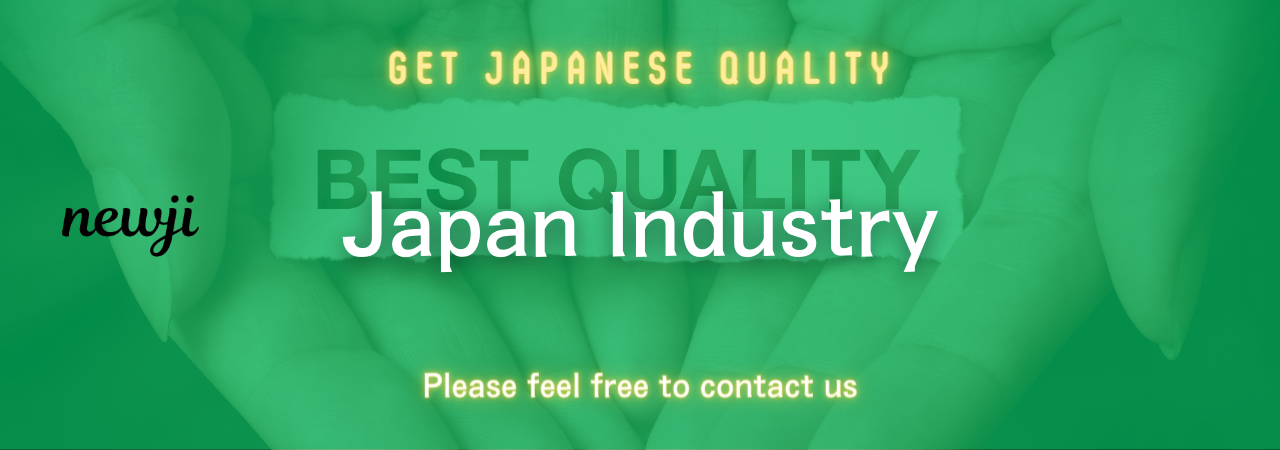
目次
Introduction to Machine Learning in Predictive Maintenance
Predictive maintenance is a strategy that uses data analysis tools and techniques to predict when equipment failures might occur.
This allows maintenance to be performed just in time before a failure happens, which can save time, money, and resources.
Machine learning plays a crucial role in enhancing predictive maintenance.
By analyzing historical data, machine learning models can identify patterns and trends that signal potential equipment failures.
These insights enable companies to plan maintenance schedules more effectively, increasing operational efficiency.
How Machine Learning Enhances Predictive Maintenance
Machine learning can process vast amounts of data much faster and more accurately than human analysts.
It can analyze historical data from various sources, such as sensor readings, maintenance records, and operational logs.
By applying machine learning algorithms, predictive maintenance systems can learn the normal operating behavior of equipment.
This learning allows the system to detect anomalies that may signify impending failures.
Moreover, machine learning models improve over time as they receive more data feedback, continuously enhancing their accuracy.
Algorithms such as regression analysis and time-series forecasting are fundamental in predicting equipment failures.
They can help identify when a machine will likely fail, allowing maintenance teams to act accordingly.
The Role of Machine Learning in Product Inspection
Product inspection is crucial to maintaining quality throughout the manufacturing process.
Machine learning can significantly enhance product inspection by automating and improving the accuracy of quality checks.
Automated Quality Control
Traditionally, product inspection is a manual and labor-intensive process.
Inspectors visually check products for defects, which is time-consuming and prone to human error.
Machine learning can automate this process through image recognition and computer vision technologies.
By training algorithms on images of both defective and defect-free products, machine learning models can learn to differentiate between them.
These models can then inspect products quickly and with high accuracy, reducing the likelihood of defective products reaching consumers.
Consistent and Objective Inspections
Machine learning ensures more consistent inspections compared to human inspectors, who may suffer from fatigue or subjective biases.
Automated inspections powered by machine learning provide objective and reproducible results, helping maintain consistent product quality.
This consistency is crucial for industries where precision is essential, such as in electronics or pharmaceuticals.
Key Points in Utilizing Machine Learning
Implementing machine learning for predictive maintenance and product inspection involves several critical considerations.
Data Quality and Quantity
High-quality and abundant data are fundamental to training effective machine learning models.
Organizations need to ensure that the data collected from their operations is accurate, relevant, and comprehensive.
The more data available, the better the machine learning models can learn and generalize insights.
However, it is also essential to clean and preprocess the data to remove any inconsistencies or noise that could lead to inaccurate predictions.
Choosing the Right Algorithms
Different machine learning tasks require different types of algorithms.
For predictive maintenance, algorithms like regression models, decision trees, and neural networks are commonly used.
In product inspection, convolutional neural networks (CNNs) are often the preferred choice due to their prowess in image recognition tasks.
Selecting the right algorithm depends on the specific needs of the application and the nature of the available data.
Integration with Existing Systems
Integrating machine learning models into existing maintenance and quality control systems can be challenging.
It requires compatibility between the machine learning solutions and the current IT infrastructure.
This integration should be seamless to avoid disruptions in operations.
IT and data science teams need to work together to ensure that machine learning models integrate efficiently with existing hardware and software.
Challenges and Future Prospects
Despite its potential, utilizing machine learning for predictive maintenance and product inspection is not without challenges.
One of the primary hurdles is the availability of labeled data for training machine learning models.
Creating labeled datasets can be costly and labor-intensive.
Additionally, the effectiveness of machine learning models heavily relies on the quality of data, as poor data can lead to erroneous predictions.
There is also the challenge of keeping machine learning systems up to date with the latest data and trends in technology.
In the future, as machine learning tools continue to evolve, we can anticipate more sophisticated algorithms capable of making even more precise predictions.
We can also expect improved integration capabilities, enabling easier implementation across various industrial sectors.
Such advancements will likely drive further adoption of machine learning in predictive maintenance and product inspection, helping industries maintain higher standards of efficiency and quality.
Conclusion
Machine learning has proven to be a game-changer in the fields of predictive maintenance and product inspection.
By leveraging its capabilities, organizations can drastically reduce downtime, improve quality, and enhance productivity.
While challenges remain, the potential benefits and continued advancements in technology make it a worthwhile investment for industries aiming to sustain competitive advantages.
資料ダウンロード
QCD調達購買管理クラウド「newji」は、調達購買部門で必要なQCD管理全てを備えた、現場特化型兼クラウド型の今世紀最高の購買管理システムとなります。
ユーザー登録
調達購買業務の効率化だけでなく、システムを導入することで、コスト削減や製品・資材のステータス可視化のほか、属人化していた購買情報の共有化による内部不正防止や統制にも役立ちます。
NEWJI DX
製造業に特化したデジタルトランスフォーメーション(DX)の実現を目指す請負開発型のコンサルティングサービスです。AI、iPaaS、および先端の技術を駆使して、製造プロセスの効率化、業務効率化、チームワーク強化、コスト削減、品質向上を実現します。このサービスは、製造業の課題を深く理解し、それに対する最適なデジタルソリューションを提供することで、企業が持続的な成長とイノベーションを達成できるようサポートします。
オンライン講座
製造業、主に購買・調達部門にお勤めの方々に向けた情報を配信しております。
新任の方やベテランの方、管理職を対象とした幅広いコンテンツをご用意しております。
お問い合わせ
コストダウンが利益に直結する術だと理解していても、なかなか前に進めることができない状況。そんな時は、newjiのコストダウン自動化機能で大きく利益貢献しよう!
(Β版非公開)