- お役立ち記事
- Utilization of traditional design of experiments and AI
月間76,176名の
製造業ご担当者様が閲覧しています*
*2025年3月31日現在のGoogle Analyticsのデータより
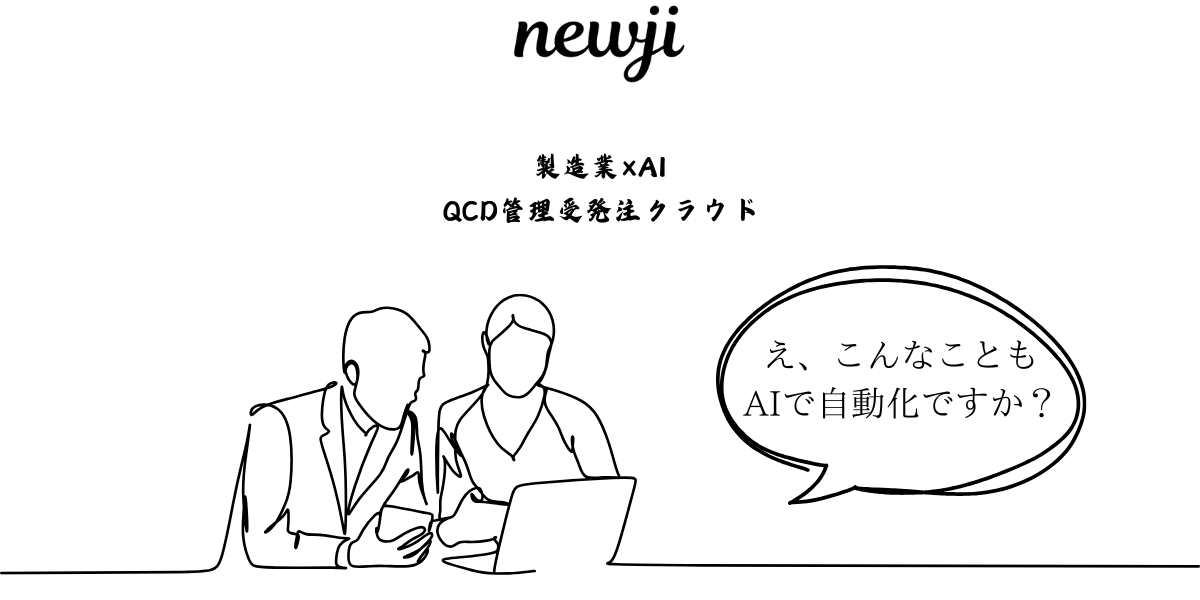
Utilization of traditional design of experiments and AI
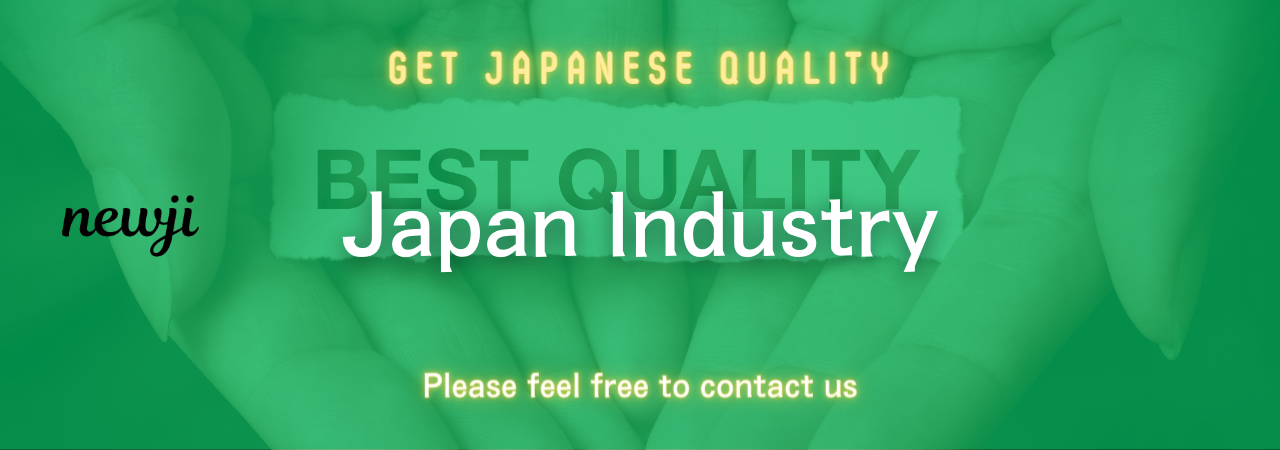
目次
Understanding Traditional Design of Experiments
Design of experiments (DOE) is a structured, organized method for determining the relationship between factors affecting a process and the output of that process.
The traditional design of experiments involves a systematic approach to planning and conducting experiments and analyzing the results.
It helps in identifying which inputs (or factors) have the most significant effect on the output, allowing researchers and engineers to make data-driven decisions.
In a typical traditional DOE, experiments are conducted by varying one or more factors systematically.
The goal is to understand the effect of these variables on the system or process being studied.
For example, in a manufacturing process, factors like temperature, pressure, or speed may be studied to determine their impact on product quality.
The traditional DOE uses several different designs such as factorial, fractional factorial, and response surface methods.
The choice of design depends on the complexity of the experiment and the number of factors being tested.
These methods require careful planning and execution to ensure accurate and reliable results.
Benefits of Traditional Design of Experiments
One of the main advantages of traditional DOE is its ability to provide a clear understanding of how different factors influence an outcome.
Researchers can pinpoint key factors that have the most significant effects and thus optimize the process.
This informed decision-making process not only improves efficiency but also minimizes costs by reducing the number of experiments needed.
Traditional DOE is also highly structured, which means it provides a systematic way to handle complex experiments.
The structured approach allows researchers to control for variability and ensure the reliability of results, which is crucial in scientific and industrial applications.
Furthermore, the insights gained from traditional DOE can be vital in establishing process control and establishing standards for quality assurance.
It aids in defining critical process parameters that must be maintained to ensure a product or service meets its quality requirements.
The Role of Artificial Intelligence in Experiments
Artificial Intelligence (AI) is revolutionizing many fields, including experimental research.
AI brings powerful computational tools and methods to the table, enabling researchers to handle large datasets and detect patterns that would be impractical through traditional means.
AI can complement traditional DOE by providing sophisticated modeling techniques.
Algorithms such as machine learning can analyze vast amounts of data to find complex relationships and trends that may be missed with a conventional DOE approach.
The incorporation of AI in experiments often leads to more flexible, dynamic models that can adjust to new data more effectively.
This capability helps in predicting outcomes with precision and tailoring experiments based on real-time results.
AI Techniques Used in Experiments
Machine learning algorithms are prominently used in AI-driven experimental designs.
These algorithms can learn from data without explicit programming and are used to recognize patterns and predict outcomes.
Neural networks, a subset of machine learning, are particularly powerful in handling vast and unstructured data.
They are used in experiments that involve complex systems where the relationships between variables are not linear or clearly defined.
Another AI tool is optimization algorithms, which can process multiple variables and constraints to find optimal solutions.
This is especially useful in experiments where resources are limited, allowing for the best possible outcomes with minimal effort or cost.
AI also plays a crucial role in data analysis and visualization, providing researchers a clearer understanding of experimental data through advanced visualizations and insights.
Integrating Traditional DOE with AI
The integration of traditional DOE with AI presents a hybrid approach that leverages the strengths of both methodologies.
While traditional DOE excels at systematic experimentation and control, AI offers computational power and adaptability.
By combining these approaches, researchers can create more robust and comprehensive experimental designs.
This integration allows for greater flexibility as AI can adjust experimental conditions in real time, based on initial results and ongoing data analysis.
For instance, AI can be used to process initial DOE data, identifying patterns that may suggest new hypotheses or directions for research.
These insights can guide subsequent experiments, making the entire process more efficient and informed.
Practical Applications of Integrating DOE and AI
Industries such as pharmaceuticals, agriculture, and manufacturing can significantly benefit from merging DOE with AI.
In pharmaceuticals, this approach can expedite drug discovery processes by quickly identifying the most promising compounds for development.
In agriculture, AI-driven experiments can help optimize crop yield by understanding the most effective growth conditions.
By integrating traditional DOE with AI, farmers can tailor practices that lead to better productivity and sustainability.
Manufacturers can use the combined approach to improve process optimization and quality control by adapting production processes based on real-time data and predictive models.
This results in higher efficiency and reduced waste.
Conclusion
The utilization of traditional design of experiments alongside AI offers a powerful framework for conducting research and improving processes.
Traditional DOE provides a rigorous, structured approach, while AI introduces flexibility, adaptability, and the capability to handle complex and large datasets.
By leveraging the strengths of both methodologies, researchers and companies can achieve more precise, reliable, and cost-effective outcomes.
This hybrid approach will likely play a significant role in shaping the future of experimental research across various industries, fostering innovation and advancement.
資料ダウンロード
QCD管理受発注クラウド「newji」は、受発注部門で必要なQCD管理全てを備えた、現場特化型兼クラウド型の今世紀最高の受発注管理システムとなります。
ユーザー登録
受発注業務の効率化だけでなく、システムを導入することで、コスト削減や製品・資材のステータス可視化のほか、属人化していた受発注情報の共有化による内部不正防止や統制にも役立ちます。
NEWJI DX
製造業に特化したデジタルトランスフォーメーション(DX)の実現を目指す請負開発型のコンサルティングサービスです。AI、iPaaS、および先端の技術を駆使して、製造プロセスの効率化、業務効率化、チームワーク強化、コスト削減、品質向上を実現します。このサービスは、製造業の課題を深く理解し、それに対する最適なデジタルソリューションを提供することで、企業が持続的な成長とイノベーションを達成できるようサポートします。
製造業ニュース解説
製造業、主に購買・調達部門にお勤めの方々に向けた情報を配信しております。
新任の方やベテランの方、管理職を対象とした幅広いコンテンツをご用意しております。
お問い合わせ
コストダウンが利益に直結する術だと理解していても、なかなか前に進めることができない状況。そんな時は、newjiのコストダウン自動化機能で大きく利益貢献しよう!
(β版非公開)