- お役立ち記事
- Visualization of multidimensional data using principal component analysis
この記事は、当社の提供するお役立ち記事の一部です。詳しくは公式サイトをご覧ください。
Visualization of multidimensional data using principal component analysis
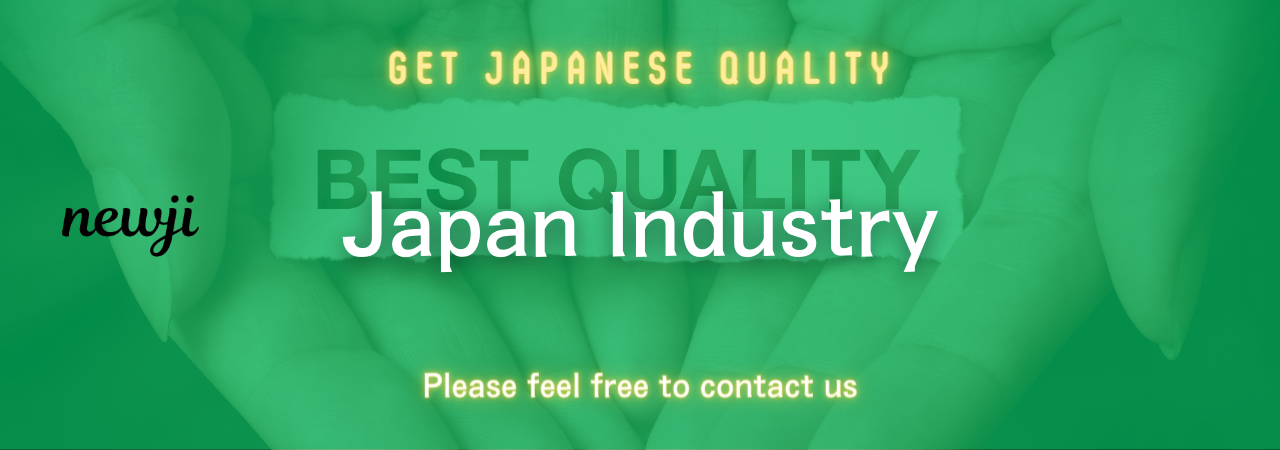
目次
Understanding Multidimensional Data
Multidimensional data is all around us.
Whenever you log in to a social media app, make an online purchase, or fill out a survey, you’re creating data.
Each piece of that data can have multiple attributes.
For instance, in a survey, you might have attributes like age, gender, preferences, and feedback.
These attributes create a data set with many dimensions.
Analyzing and visualizing such multidimensional data can be complex.
The Importance of Visualizing Data
Before we dive deeper, let’s take a moment to understand why visualizing data is crucial.
When data is presented visually, it becomes easier to detect patterns, trends, and outliers.
Visualization helps transform complex data structures into insights that are easier to comprehend.
Imagine a business looking at consumer preferences across different regions.
Using visual tools, it can quickly spot areas with high product demand or dissatisfaction.
Introduction to Principal Component Analysis
Principal Component Analysis (PCA) is a savior in the realm of multidimensional data.
But what exactly is PCA, and how does it help in visualization?
PCA is a statistical procedure.
Its main aim is to convert a set of correlated variables into a set of uncorrelated variables, termed principal components.
It reduces the dimensionality of the data while retaining as much variation as possible.
Think of PCA as compacting a big suitcase.
It’s about squeezing in all the essentials without exceeding the weight limit.
How PCA Works
To grasp how PCA functions, let’s break it down:
1. **Standardization**:
The first step is to standardize the data.
This involves transforming data into a standard format, ensuring that each variable contributes equally.
2. **Covariance Matrix Computation**:
Once standardized, PCA computes the covariance matrix.
It shows the relationship between different variables.
In simpler terms, it tells you how variables move together.
3. **Eigenvalues and Eigenvectors**:
These are mathematical constructs derived from the covariance matrix.
Eigenvectors determine the direction of the new data dimensions.
Eigenvalues, on the other hand, decide how significant each of these dimensions is.
4. **Feature Vector Formation**:
The next step involves selecting the components that capture most variability.
This is called forming a feature vector.
5. **Reformulating the Data**:
Finally, using the feature vector, you transform the dataset into its principal components.
This results in new dimensions that are uncorrelated.
The Benefits of Using PCA
One might wonder, why opt for PCA?
Here are some compelling reasons:
– **Dimensionality Reduction**:
With huge datasets, it’s tough to pinpoint what matters.
PCA reduces the number of dimensions, focusing on what’s truly important.
This makes analysis more efficient.
– **Data Visualization**:
Even complex, multidimensional data can be visualized in just two or three dimensions using PCA.
Imagine a tangled ball of yarn turned into a straight line.
– **Noise Reduction**:
Unnecessary noise can obscure true patterns.
PCA filters that noise, highlighting the genuine signals in your data.
– **Compares and Contrasts**:
PCA simplifies how different variables relate to one another, making comparisons easier.
The Limitations of PCA
It’s essential to remember that no tool is perfect.
While PCA offers numerous advantages, it does come with limitations:
– **Linear Assumptions**:
PCA assumes linear relationships, making it less effective for non-linear data distributions.
– **Interpretation Challenges**:
When dimensions get reduced, understanding what each principal component represents can be challenging.
– **Data Sensitivity**:
PCA can be influenced by outliers in the data, possibly skewing results.
Practical Applications of PCA
PCA’s versatility finds applications across sectors:
– **Finance**:
Stock market analysts use PCA to identify trends and predict market movements.
– **Biology**:
Biologists employ PCA to analyze gene expression patterns and understand diseases.
– **Image Processing**:
In facial recognition technology, PCA helps in reducing image dimensions, speeding up the recognition process.
– **Marketing**:
Marketers use PCA to segment consumer groups, tailoring campaigns to specific audience needs.
Conclusion
Principal Component Analysis is indeed a powerful ally in the world of multidimensional data analysis.
It simplifies complex data, uncovering insights previously hidden by sheer volume.
Whether you’re in finance, marketing, biology, or any field dealing with intricate data, PCA can be your guiding light.
While it does have its limitations, its strength in providing clear, actionable insights makes it a valuable tool in any analyst’s toolkit.
Remember, every piece of data tells a story.
PCA helps you to hear that story loud and clear.
資料ダウンロード
QCD調達購買管理クラウド「newji」は、調達購買部門で必要なQCD管理全てを備えた、現場特化型兼クラウド型の今世紀最高の購買管理システムとなります。
ユーザー登録
調達購買業務の効率化だけでなく、システムを導入することで、コスト削減や製品・資材のステータス可視化のほか、属人化していた購買情報の共有化による内部不正防止や統制にも役立ちます。
NEWJI DX
製造業に特化したデジタルトランスフォーメーション(DX)の実現を目指す請負開発型のコンサルティングサービスです。AI、iPaaS、および先端の技術を駆使して、製造プロセスの効率化、業務効率化、チームワーク強化、コスト削減、品質向上を実現します。このサービスは、製造業の課題を深く理解し、それに対する最適なデジタルソリューションを提供することで、企業が持続的な成長とイノベーションを達成できるようサポートします。
オンライン講座
製造業、主に購買・調達部門にお勤めの方々に向けた情報を配信しております。
新任の方やベテランの方、管理職を対象とした幅広いコンテンツをご用意しております。
お問い合わせ
コストダウンが利益に直結する術だと理解していても、なかなか前に進めることができない状況。そんな時は、newjiのコストダウン自動化機能で大きく利益貢献しよう!
(Β版非公開)