- お役立ち記事
- Fundamentals of surrogate models and their application to improving product design efficiency: Fusion technology of CAE and data science
Fundamentals of surrogate models and their application to improving product design efficiency: Fusion technology of CAE and data science
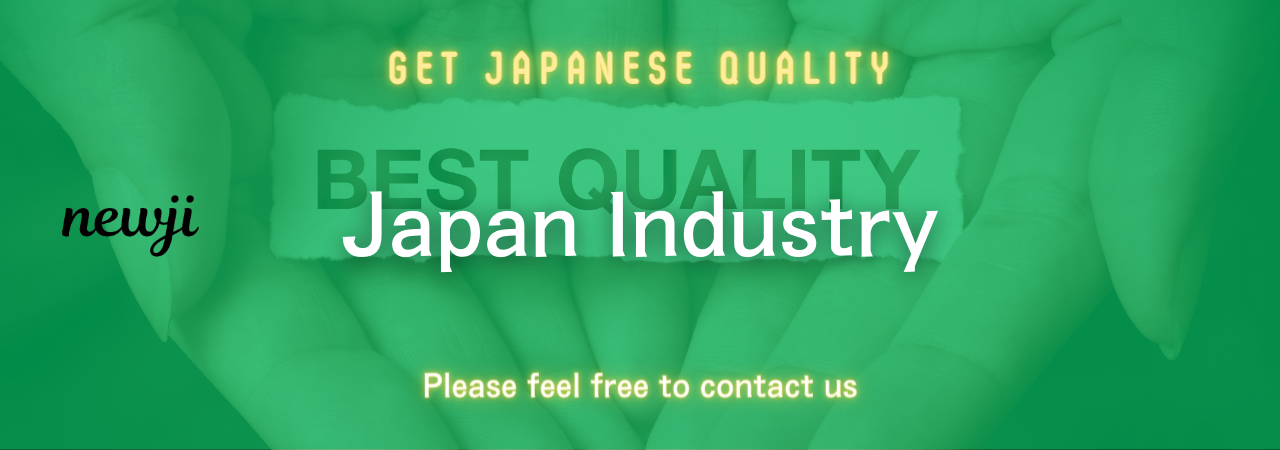
目次
Understanding Surrogate Models
Surrogate models are mathematical approaches used to approximate complex simulations or physical experiments.
They serve as substitutes for more computationally intensive processes, allowing quicker evaluations.
In essence, surrogate models act as simplified representations of a more complex system.
The primary motivation behind using surrogate models is to enhance efficiency.
When dealing with complex systems, especially in areas like computational fluid dynamics or structural analysis, simulations can be time-consuming and expensive.
Surrogate models help reduce this computational cost by providing a faster alternative.
These models are often constructed using a set of existing data, acting as a bridge between comprehensive simulations and real-time analysis.
By doing so, they enable engineers and designers to make informed decisions quickly.
Types of Surrogate Models
There are several types of surrogate models, each with its strengths and weaknesses.
Some of the most common types include:
1. **Response Surface Models (RSM):** These models use polynomial approximations to predict outcomes.
They are suitable for problems where responses are smooth and continuous.
2. **Kriging Models:** Known for their flexibility, Kriging models are often used in geostatistics.
They provide an estimate for unknown points using a weighted average of nearby known points.
3. **Artificial Neural Networks (ANNs):** These models mimic the functioning of the human brain.
They are beneficial for capturing complex, non-linear relationships within data sets.
4. **Radial Basis Function Models:** This type employs functions centered at data points to interpolate or extrapolate data.
They are effective in multi-dimensional interpolation tasks.
Each surrogate model type has its applications depending upon the problem’s nature and data complexity.
Implementation in Product Design
The integration of surrogate models in product design significantly enhances efficiency.
Product design often involves iterative testing and refining processes.
These processes can be time-consuming with traditional methodologies.
Surrogate models facilitate rapid iteration by providing quick evaluations.
Design variations can be tested and refined with less computational resource usage.
This results in a more streamlined design cycle, reducing the time to market.
In industries like automotive or aerospace, these efficiencies translate into massive cost savings.
Engineers can simulate various scenarios without running exhaustive physical tests.
Enhancing Computer-Aided Engineering (CAE)
Surrogate models play a pivotal role in enhancing Computer-Aided Engineering (CAE).
CAE tools are integral during the design process, enabling virtual testing and optimization.
However, these tools often involve computationally expensive simulations.
By incorporating surrogate models, the CAE process becomes more efficient.
Engineers can run numerous simulations with much faster turnaround times.
This allows for extensive exploration of design spaces, leading to better optimization.
The amalgamation of CAE and surrogate models paves the way for more innovative designs.
With the ability to evaluate hundreds of design variations quickly, engineers can push boundaries and explore unconventional solutions.
The Role of Data Science
Data science further enhances the capabilities of surrogate models.
With advancements in machine learning and data analytics, surrogate models have become more accurate and reliable.
Data science techniques, such as training with large datasets, provide surrogate models with the ability to learn from historical data.
This learning process improves the model’s prediction accuracy, making it more useful for real-world applications.
Moreover, through data science, surrogate models can adapt to changes and new data inputs.
This adaptability ensures that the models remain relevant and useful over time.
Fusion Technology: Bridging Gaps
Integrating CAE and data science through surrogate models is a fusion technology.
It represents a significant advancement in the way engineering and design processes are approached.
This fusion allows for a comprehensive understanding of the design process.
By bridging the gap between traditional engineering techniques and modern data-driven approaches, efficiency is heightened.
By utilizing data-driven insights, engineers can uncover hidden patterns and trends during the design process.
This leads to more informed decisions and innovative product developments.
Future Prospects and Challenges
The future of surrogate models holds tremendous potential.
As computational power grows and machine learning techniques evolve, the capability and accuracy of these models will only increase.
However, certain challenges remain.
The need for high-quality datasets is crucial for accurate surrogate model development.
Without sufficient data, these models may fail to capture the complexities of real-world scenarios.
Furthermore, balancing model accuracy with computational efficiency is an ongoing challenge.
While surrogate models reduce computation times, ensuring their predictions remain reliable is vital.
The ethical use of data and ensuring privacy in model development is also a concern.
As more data is utilized, keeping it secure and anonymized is imperative.
Conclusion
Surrogate models represent a transformative approach in improving product design efficiency.
By serving as substitutes for detailed simulations, they provide faster evaluations, streamline the design process, and enable comprehensive exploration of design alternatives.
The fusion of CAE and data science within surrogate models exemplifies the advancing synergy between traditional engineering and modern data methodologies.
While challenges remain, the potential benefits in cost savings, design innovation, and competitive advantage make them a staple in contemporary engineering practices.
As technology progresses, the continued refinement and application of surrogate models promise to drive further innovation and efficiency across various sectors, ultimately shaping the future of product design and engineering.
資料ダウンロード
QCD調達購買管理クラウド「newji」は、調達購買部門で必要なQCD管理全てを備えた、現場特化型兼クラウド型の今世紀最高の購買管理システムとなります。
ユーザー登録
調達購買業務の効率化だけでなく、システムを導入することで、コスト削減や製品・資材のステータス可視化のほか、属人化していた購買情報の共有化による内部不正防止や統制にも役立ちます。
NEWJI DX
製造業に特化したデジタルトランスフォーメーション(DX)の実現を目指す請負開発型のコンサルティングサービスです。AI、iPaaS、および先端の技術を駆使して、製造プロセスの効率化、業務効率化、チームワーク強化、コスト削減、品質向上を実現します。このサービスは、製造業の課題を深く理解し、それに対する最適なデジタルソリューションを提供することで、企業が持続的な成長とイノベーションを達成できるようサポートします。
オンライン講座
製造業、主に購買・調達部門にお勤めの方々に向けた情報を配信しております。
新任の方やベテランの方、管理職を対象とした幅広いコンテンツをご用意しております。
お問い合わせ
コストダウンが利益に直結する術だと理解していても、なかなか前に進めることができない状況。そんな時は、newjiのコストダウン自動化機能で大きく利益貢献しよう!
(Β版非公開)